Fast Descriptor Extraction for Contextless 3D Registration Using a Fully Convolutional Network
2018 24TH INTERNATIONAL CONFERENCE ON PATTERN RECOGNITION (ICPR)(2018)
摘要
In recent years, numerous consumer devices have emerged that are capable of capturing 3D point data originating from depth images. Many computer vision tasks, such as object recognition, environment mapping, augmented reality, and more, rely on accurately registering 3D point sets. One method to compute this registration is to use 3D local feature descriptors for a coarse alignment, and to further refine the alignment with a variant of the Iterative Closest Point algorithm. While robust feature descriptors work well for this approach, online computation for all points in a single depth image is typically intractable. In this work, a method to facilitate real-time 3D registration by performing descriptor extraction on depth images using a Fully Convolutional Network (FCN) is presented. The input to this method is a raw depth image and results in a 33-bin descriptor for each pixel, enabling a general-purpose 3D registration process that doesn't require future network retraining and refinement. Experimental results on consumer hardware demonstrate that the proposed method significantly outperforms the state-of-the-art in terms of computation time and approaches depth sensor frame capture times, with only a slight reduction in descriptiveness.
更多查看译文
关键词
Fully Convolutional Network,fast descriptor extraction,3D local feature descriptors,Iterative Closest Point algorithm,computer vision,contextless 3D registration,general-purpose 3D registration,FCN
AI 理解论文
溯源树
样例
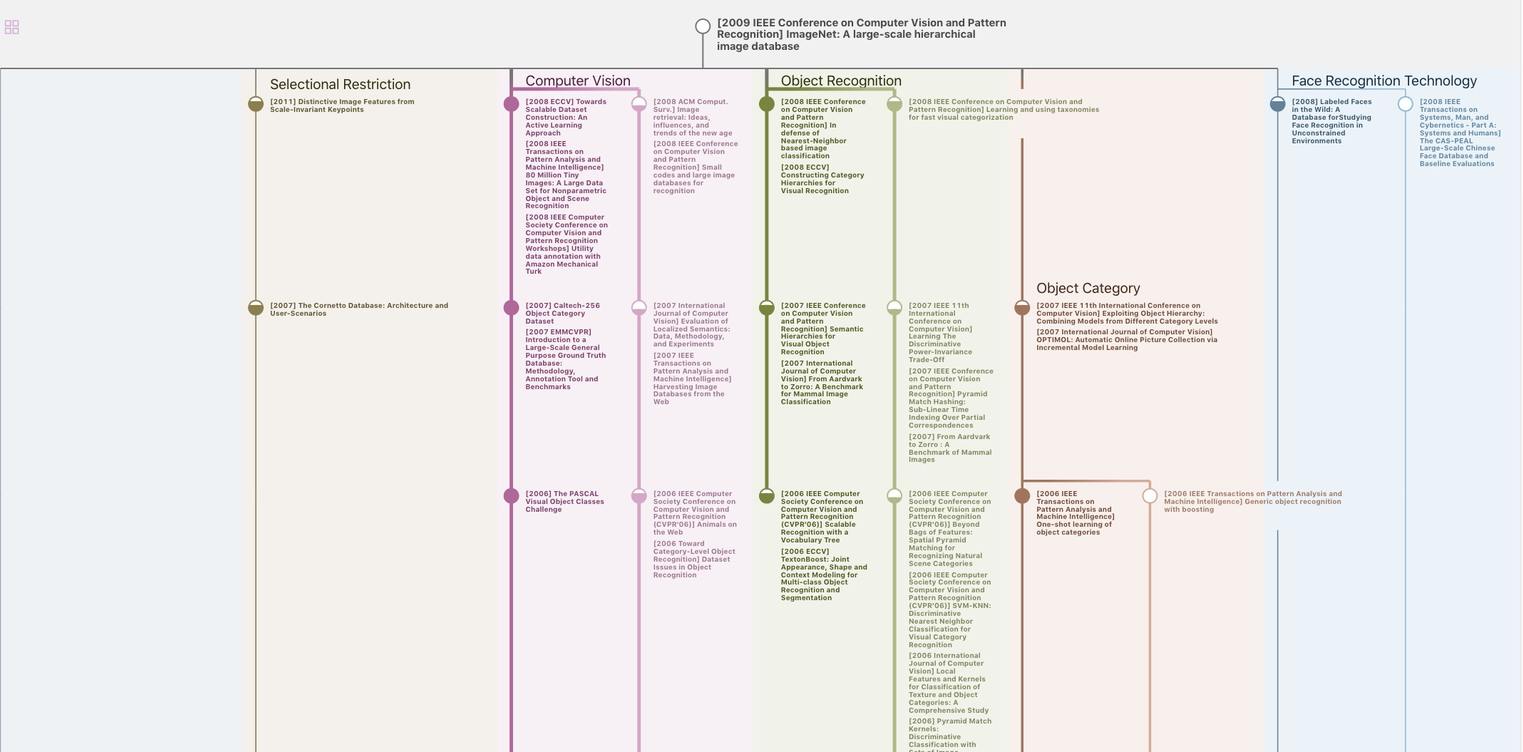
生成溯源树,研究论文发展脉络
Chat Paper
正在生成论文摘要