Medical Knowledge Constrained Semantic Breast Ultrasound Image Segmentation
2018 24TH INTERNATIONAL CONFERENCE ON PATTERN RECOGNITION (ICPR)(2018)
摘要
Computer-aided diagnosis (CAD) can help doctors in diagnosing breast cancer. Breast ultrasound (BUS) imaging is harmless, effective, portable, and is the most popular modality for breast cancer detection/diagnosis. Many researchers work on improving the performance of CAD systems. However, there are two main shortcomings: (1) Most of the existing methods are based on prerequisites that there is one and only one tumor in the image. (2) The results depend on the datasets, i.e., an algorithm using different datasets may obtain different performances. It implies that the performance of traditional methods is dataset-dependent. In this paper, we propose an effective approach: (1) using information extended images to train a fully convolutional network (FCN) to semantically segment BUS image into 3 categories: mammary layer, tumor, and background; and (2) applying layer structure information - the breast cancers are located inside the mammary layer - to the conditional random field (CRF) for conducting breast cancer segmentation and making the segmentation result more accurate. The proposed method is evaluated utilizing BUS images of 325 cases, and the result is the best comparing with that of the existing methods by achieving true positive rate 92.80%, false positive rate 9%, and Intersection over Union 82.11%. The proposed approach has solved the above mentioned two shortcomings of the existing methods.
更多查看译文
关键词
computer aided diagnosis (CAD), breast ultrasound (BUS) image, deep convolutional neural network (DCNN), semantic image segmentation, conditional random field (CRF)
AI 理解论文
溯源树
样例
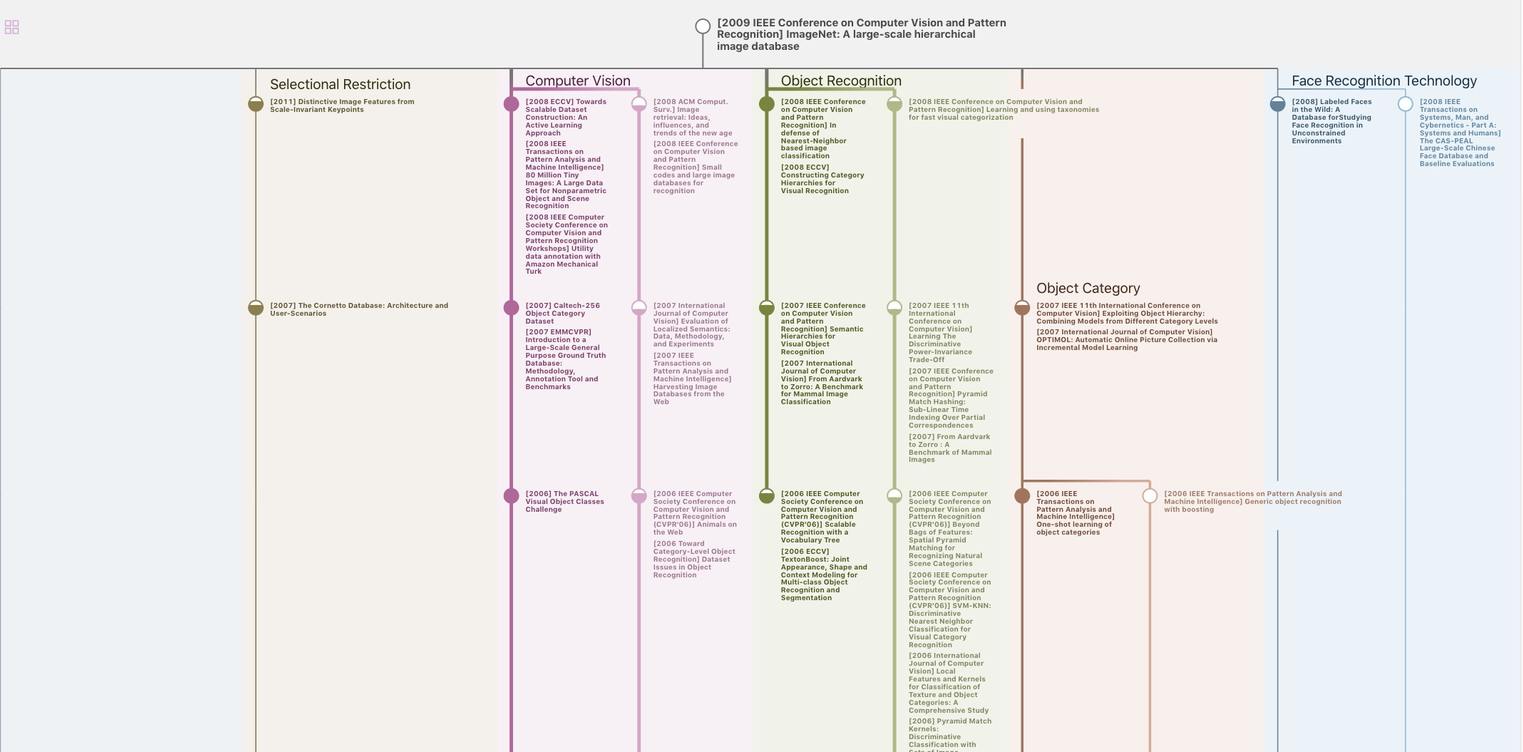
生成溯源树,研究论文发展脉络
Chat Paper
正在生成论文摘要