Focus On Scene Text Using Deep Reinforcement Learning
2018 24TH INTERNATIONAL CONFERENCE ON PATTERN RECOGNITION (ICPR)(2018)
摘要
Scene text detection has been attracting increasing interests in recent years and a rich body of approaches has been proposed. These previous works of detecting scene text have been dominated by region proposals based approaches, which always generate too many text candidates relative to the number of ground truth bounding boxes. Only a few of those candidates are output as true predictions, and most of the other is fruitlessly involved in regression or classification predictions that consume a great amount of time and storage. Thus emerges the problem of low efficiency of generating text candidates. To address the issue, we propose a method for focusing on scene text gradually guided by an active model. The model allows an agent to take the whole image as the only region proposal in each episode when locating text and therefore significantly reduces the region proposals needed. The agent is trained by deep reinforcement strategy to learn how to estimate future returns of given states and sequentially make decisions to find scene text. Considering the characteristics of scene text, we additionally propose a flexible action scheme and a new reward scheme together with lazy punishment. The experiments on the ICDAR 2013 dataset shows that the proposed method achieve a promising performance while using region proposals as few as the ground truth bounding boxes.
更多查看译文
关键词
scene text detection,region proposal,text candidates,ground truth bounding box
AI 理解论文
溯源树
样例
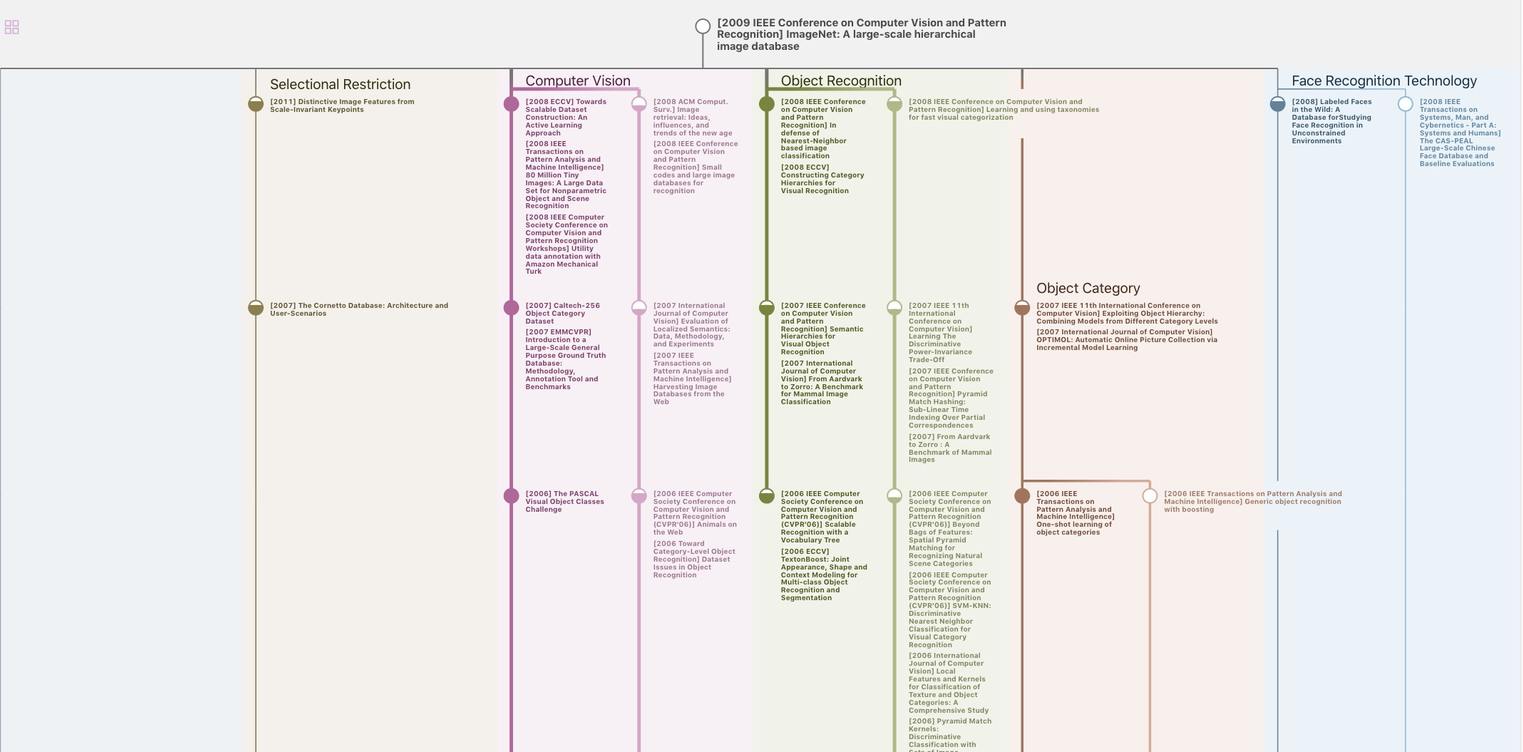
生成溯源树,研究论文发展脉络
Chat Paper
正在生成论文摘要