Anchor Free Network for Multi-Scale Face Detection
ICPR(2018)
摘要
Anchor-based deep methods are the most widely used methods for face detection and have reached the state-of-the-art result. Compared with anchor-based methods that estimates the bounding-box rely on some pre-defined anchor boxes, anchor-free methods perform the localization by predicting the offsets of a pixel inside a face to its outside boundaries whose accuracies are much more precise. However, anchor-free methods suffer the drawback of low recall-rate mainly because 1) only using single scale features lead to miss detection of small faces, 2) the highly intra-class imbalance problem among different size faces. In this paper, to address these problems, we propose a unified anchor-free network for detecting multi-scale faces by leveraging the local and global contextual information of multi-layer features. We also utilize a scale aware sampling strategy to mitigate the intra-class imbalance issue which can adaptivity select the positive samples. Furthermore, a revised focal loss function is adopted to deal with the foreground/background imbalance issue. Experimental results on two benchmark datasets demonstrate the effective of our proposed method.
更多查看译文
关键词
anchor free network,multiscale face detection,anchor-based deep methods,anchor-based methods,anchor-free methods,single scale features,intra-class imbalance problem,unified anchor-free network,multiscale faces,multilayer features,scale aware sampling strategy,intra-class imbalance issue
AI 理解论文
溯源树
样例
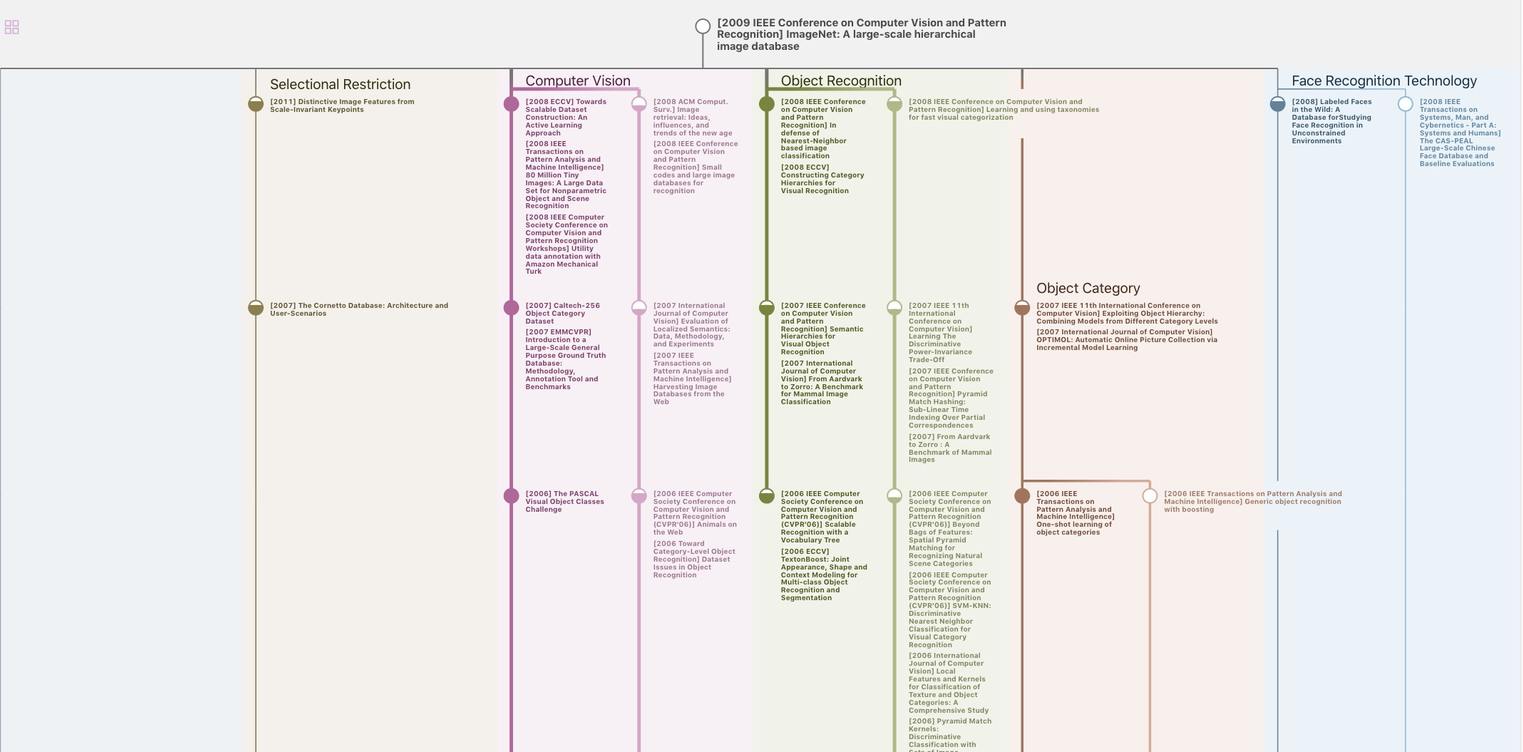
生成溯源树,研究论文发展脉络
Chat Paper
正在生成论文摘要