Enhanced Network Embedding with Text Information
2018 24th International Conference on Pattern Recognition (ICPR)(2018)
摘要
Network embedding aims at learning the low-dimensional and continuous vector representation for each node in networks, which is useful in many real applications. While most existing network embedding methods only focus on the network structure, the rich text information associated with nodes, which is often closely related to network structure, is widely neglected. Thus, how to effectively incorporate text information into network embedding is a problem worth studying. To solve the problem, we propose a Text Enhanced Network Embedding (TENE) method under the framework of non-negative matrix factorization to integrate network structure and text information together. We explore the consistent relationship between node representations and text cluster structure to make the network embedding more informative and discriminative. TENE learns the representations of nodes under the guidance of both proximity matrix which captures the network structure and text cluster membership matrix derived from clustering for text information. We evaluate the quality of network embedding on the task of multi-class classification of nodes. Experimental results on all three real-world datasets show the superior performance of TENE compared with baselines.
更多查看译文
关键词
network structure,rich text information,text cluster structure,text cluster membership matrix,low-dimensional vector representation,continuous vector representation,text enhanced network embedding method,TENE,proximity matrix
AI 理解论文
溯源树
样例
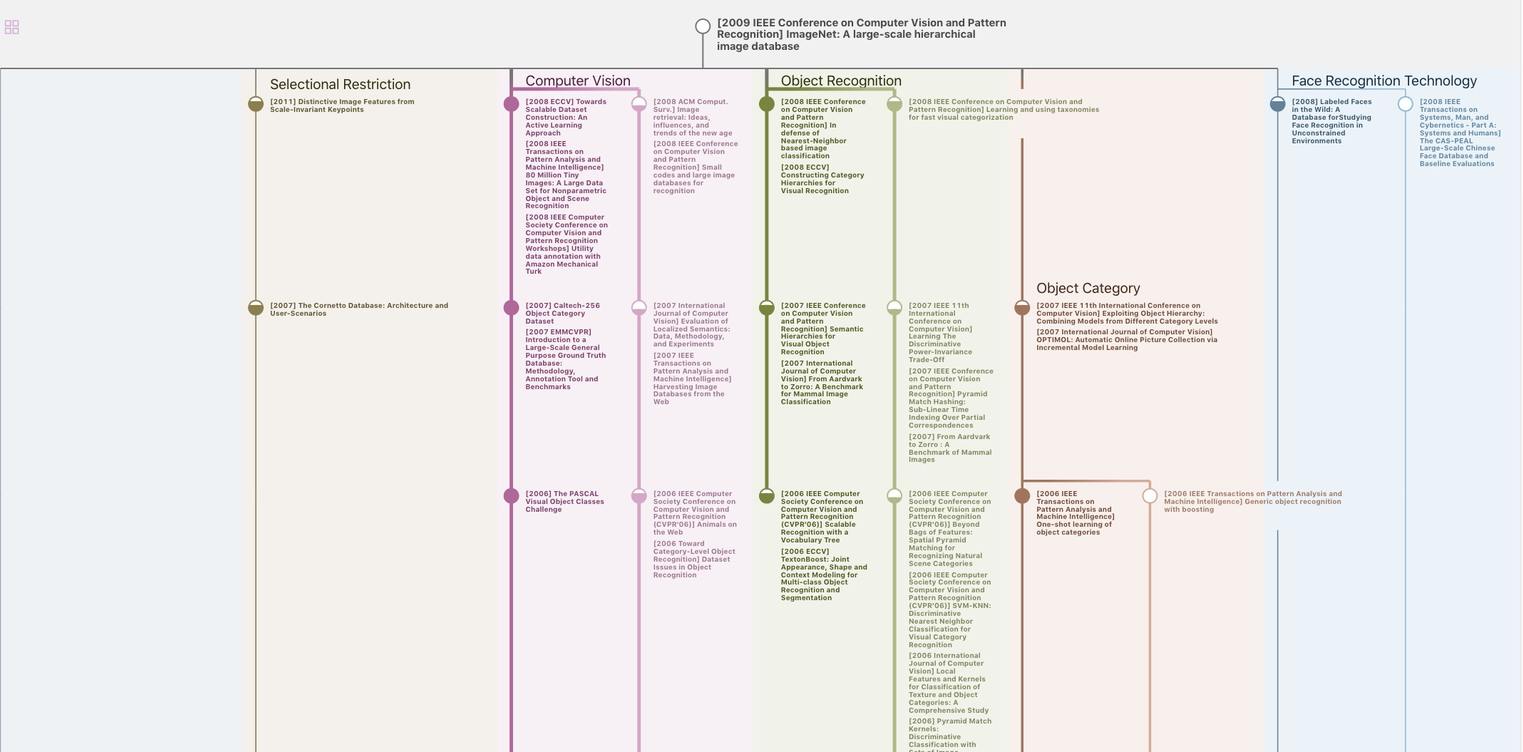
生成溯源树,研究论文发展脉络
Chat Paper
正在生成论文摘要