Learning Parallel Canonical Correlations For Scale-Adaptive Low Resolution Face Recognition
2018 24TH INTERNATIONAL CONFERENCE ON PATTERN RECOGNITION (ICPR)(2018)
摘要
Low resolution is one of the main obstacles in the application of face recognition. Although many methods have been proposed to improve the problem, they assume that low-resolution (LR) face images have a uniform scale. In real scenarios, this prerequisite is very harsh. In this paper, we propose a scale-adaptive LR face recognition approach based on two-dimensional multi-set canonical correlation analysis (2DM-CCA), where face image matrix does not need to be previously transformed into a vector. In the proposed method, training sets with different resolutions are treated as different views, and then projected in parallel into a latent coherent space where the consistency of multi-view face data is maximally enhanced. When a new LR face image with an arbitrary scale is input, we first transform it by using the left and right projection matrices of an appropriate training view, and then reconstruct its high resolution facial feature by neighborhood reconstruction. Experimental results show that our proposed method is more effective and efficient than several existing methods.
更多查看译文
关键词
face recognition, canonical correlation, low resolution, multiple resolutions
AI 理解论文
溯源树
样例
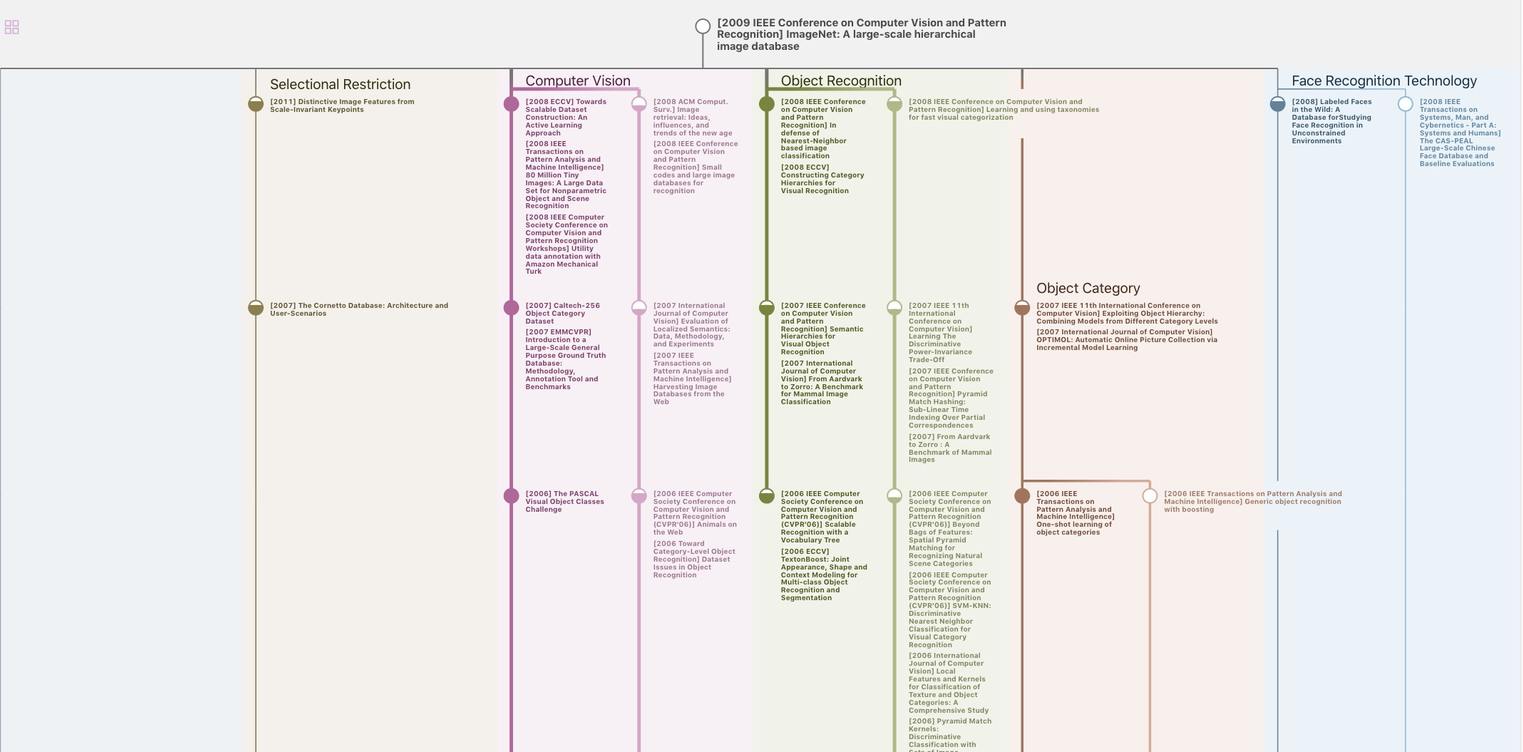
生成溯源树,研究论文发展脉络
Chat Paper
正在生成论文摘要