Privacy-Preserving Age Estimation for Content Rating
2018 IEEE 20th International Workshop on Multimedia Signal Processing (MMSP)(2018)
摘要
Content rating (aka. maturity rating) rates the suitability of kinds of media (e.g., movies and video games) to its audience. It is essential to prevent a specific age group of people such as children from inappropriate information. However, in practice the administration of content rating system is usually suggestion-based declaration by media sources or key-based password which can easily fail if someone ignores the suggestions or somehow knows the keys. In this paper, we propose to estimate user's age in a privacy-preserving manner for automatic content rating. Several privacy-preserving approaches on facial images with different degree of privacy are proposed and evaluated on a deep neural network architecture for age estimation accuracy. We also introduce an attention mechanism which can adaptively learn discriminative features from the processed facial images. Experiments show that the proposed attention-based model performs better than the baseline model and achieves a reasonable performance to that with raw images in testing.
更多查看译文
关键词
privacy-preserving age estimation,maturity rating,video games,suggestion-based declaration,media sources,automatic content rating,attention-based model,discriminative features,automatic content rating system,baseline model,deep neural network architecture,facial images,key-based password,users age estimation
AI 理解论文
溯源树
样例
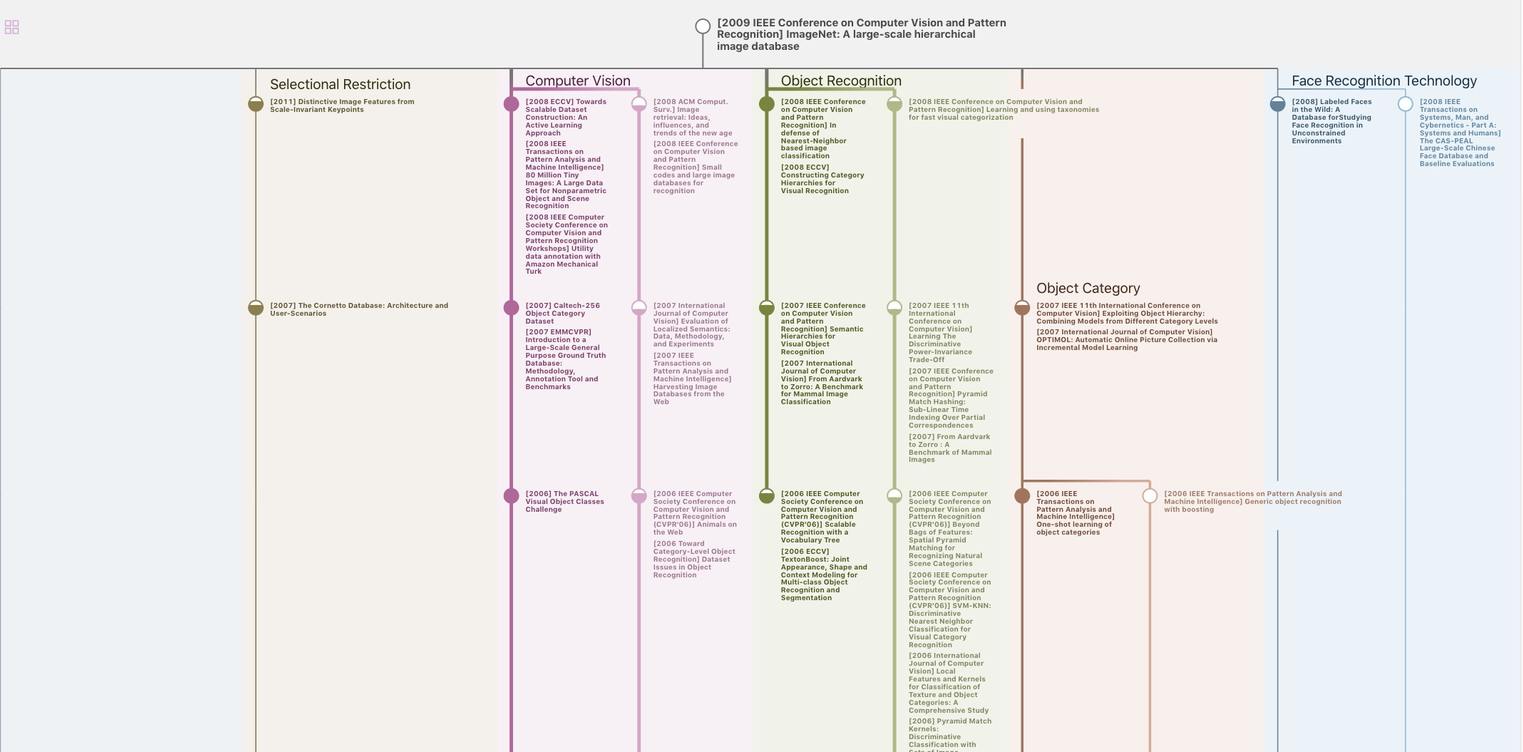
生成溯源树,研究论文发展脉络
Chat Paper
正在生成论文摘要