Evolution of Images with Diversity and Constraints Using a Generative Adversarial Network.
Neural Information Processing Lecture Notes in Computer Science(2018)
关键词
Generative Adversarial Networks (GAN),Nature-inspired Methods,Butterfly Dataset,Covariance Matrix Adaptation Evolution Strategy (CMA-ES),Deep Convolutional Generative Adversarial Networks
AI 理解论文
溯源树
样例
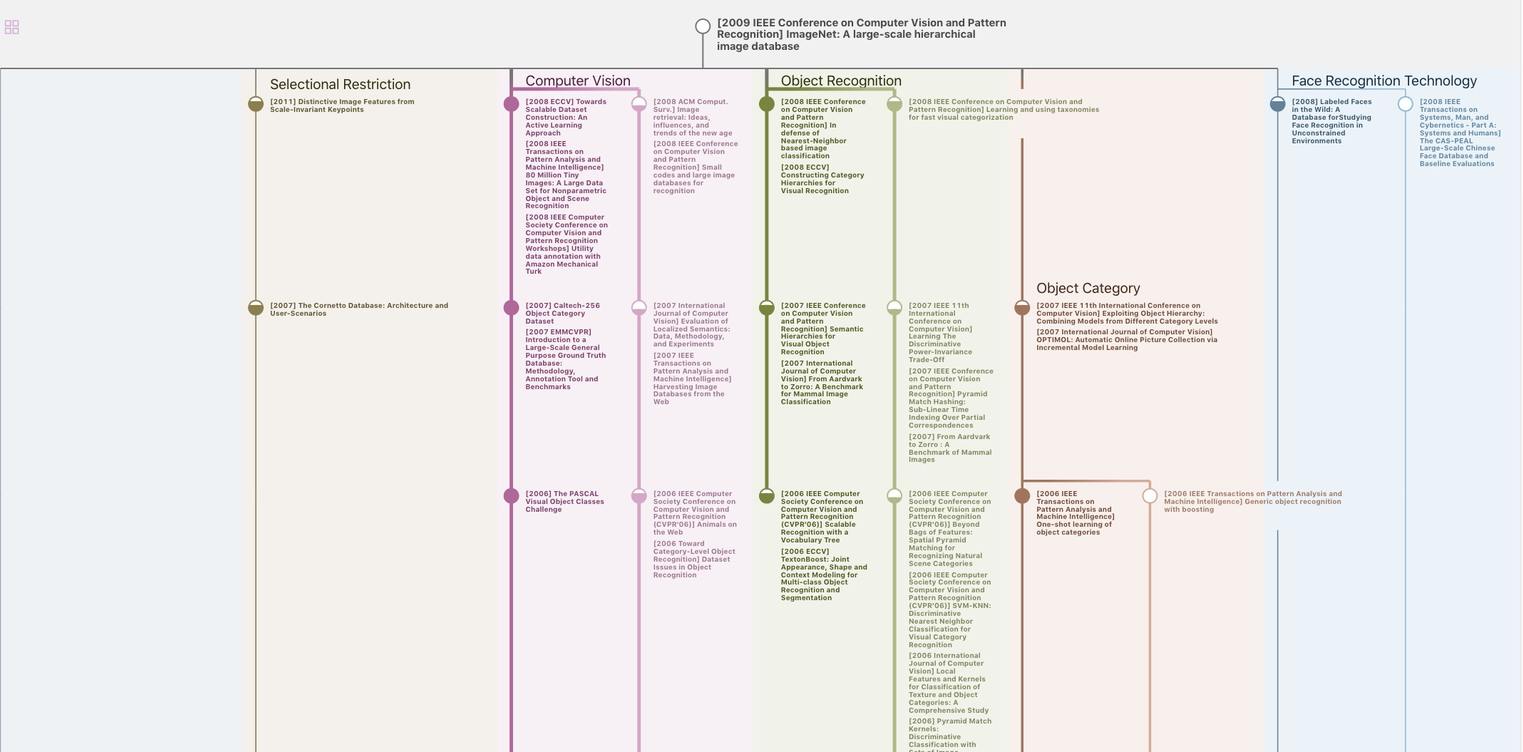
生成溯源树,研究论文发展脉络
Chat Paper
正在生成论文摘要