Cross-domain Recommendation with Probabilistic Knowledge Transfer.
ICONIP(2018)
摘要
Recommender systems have drawn great attention from both academic and practical area. One challenging and common problem in many recommendation methods is data sparsity, due to the limited number of observed user interaction with the products/services. To alleviate the data sparsity problem, cross-domain recommendation methods are developed to share group-level knowledge in several domains so that recommendation in the domain with scarce data can benefit from domains with relatively abundant data. However, divergence exists in the data of similar domains so that the extracted group-level knowledge is not always suitable to be applied in the target domain, thus recommendation accuracy in the target domain is impaired. In this paper, we propose a cross-domain recommendation method with probabilistic knowledge transfer. The proposed method maintain two sets of group-level knowledge, profiling both domain-shared and domain-specific characteristics of the data. In this way users’ mixed preferences can be profiled comprehensively thus improves the performance of the cross-domain recommender systems. Experiments are conducted on five real-world datasets in three categories: movies, books and music. The results for nine cross-domain recommendation tasks show that our proposed method has improved the accuracy compared with five benchmarks.
更多查看译文
关键词
transfer,knowledge,cross-domain
AI 理解论文
溯源树
样例
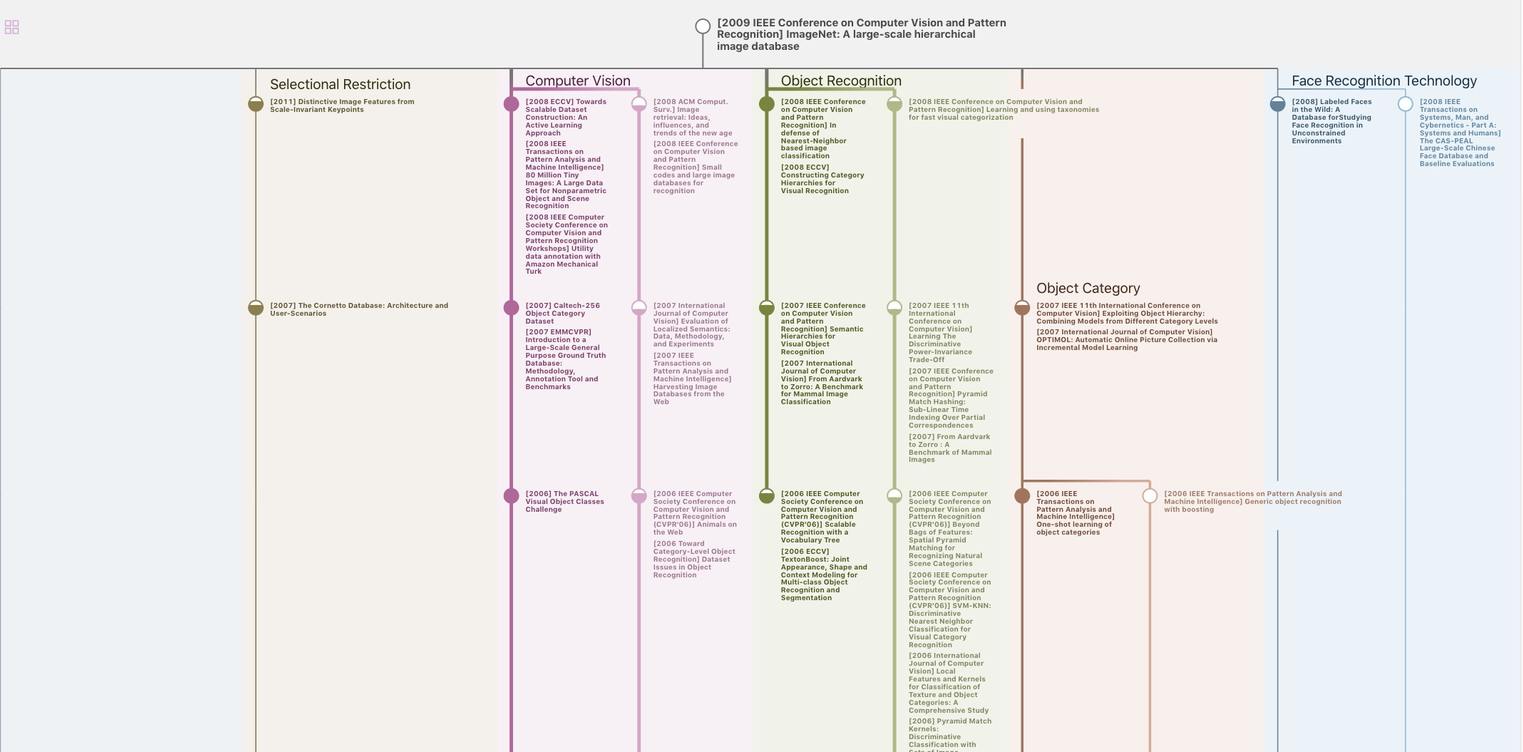
生成溯源树,研究论文发展脉络
Chat Paper
正在生成论文摘要