Fast Portrait Matting Using Spatial Detail-Preserving Network
NEURAL INFORMATION PROCESSING (ICONIP 2018), PT VI(2018)
摘要
Image matting plays an important role in both computer vision and graphics applications. Natural image matting has recently made significant progress with the assistance of powerful Convolutional Neural Networks (CNN). However, it is often time-consuming for pixel-wise label inference. To get higher quality matting in an efficient way, we propose a well-designed SDPNet, which consists of two parallel branches-Semantic Segmentation Branch for half image resolution and Detail-Preserving Branch for full resolution, capturing both the semantic information and image details, respectively. Higher quality alpha matte can be generated while largely reducing the portion of computation. In addition, Spatial Attention Module and Boundary Refinement Module are proposed to extract distinguishable boundary features. Extensive Experiments show that SDPNet provides higher quality results on Portrait Matting benchmark, while obtaining 5x to 20x faster than previous methods.
更多查看译文
关键词
Portrait, Fast matting, Detail-preserving, Deep learning
AI 理解论文
溯源树
样例
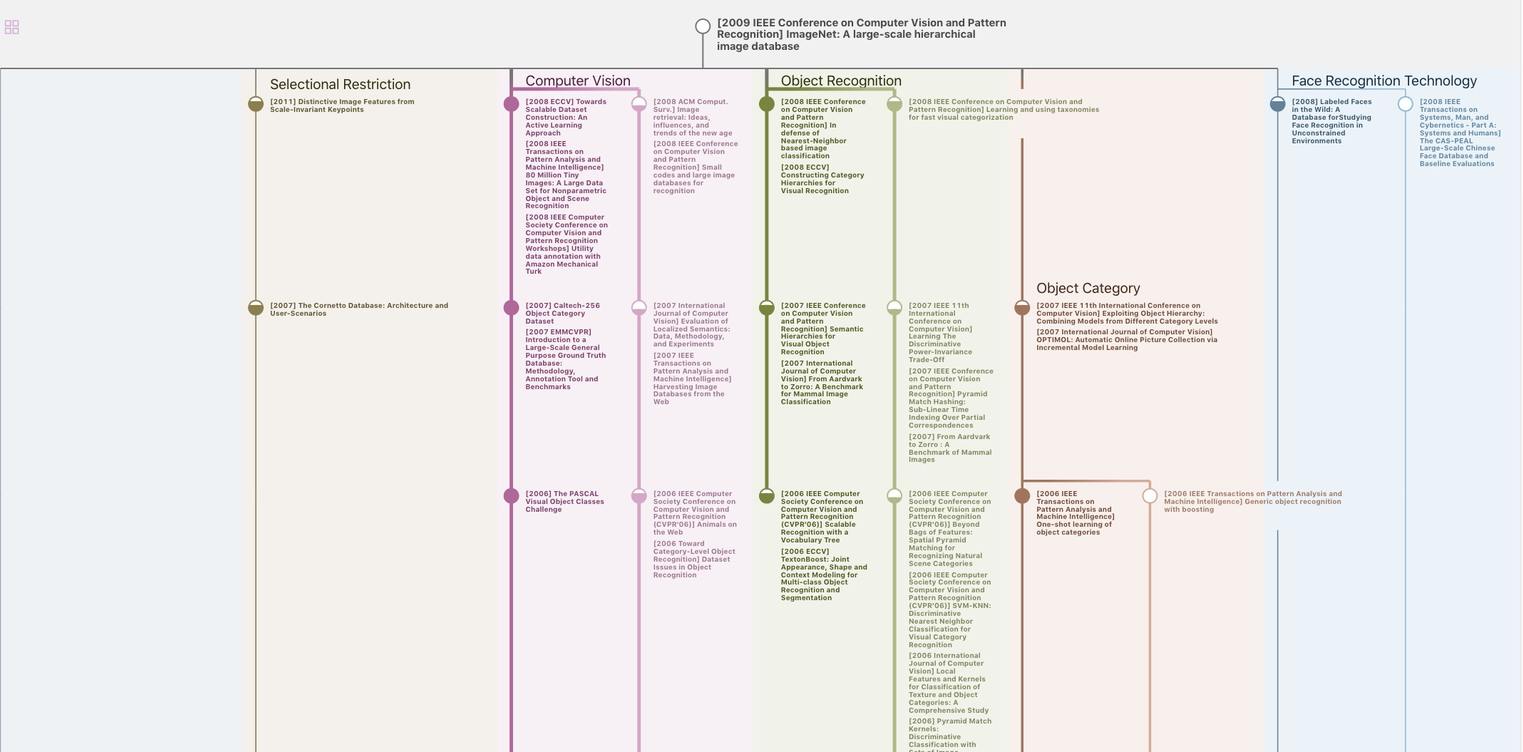
生成溯源树,研究论文发展脉络
Chat Paper
正在生成论文摘要