Modelling And Analysis Of Temporal Gene Expression Data Using Spiking Neural Networks
NEURAL INFORMATION PROCESSING (ICONIP 2018), PT I(2018)
摘要
Analysis of temporal gene expression data poses a significant challenge due to the combination of high dimensionality and low sample size. The purpose of this paper is to present a methodology for classification, modelling, and analysis of short time-series gene expression data using spiking neural networks (SNN) and to uncover temporal expression patterns for knowledge discovery. The classification is based on the NeuCube SNN model. Time-series gene expression data of mouse primary cortical neurons is examined as a case study. The results of the analysis are promising, indicating that SNN methodologies can be effectively used to model and analyse temporal gene expression data with surpassing performance over traditional machine learning algorithms. Additionally, a gene interaction network is constructed from the temporal gene activity modelled using the NeuCube architecture offering a new way of knowledge discovery. Future work will be directed towards using gene interactions networks to help guide pharmacological research for dementia.
更多查看译文
关键词
Spiking neural networks, Gene interaction networks, Gene expression, Microarray, Transcriptome data analysis
AI 理解论文
溯源树
样例
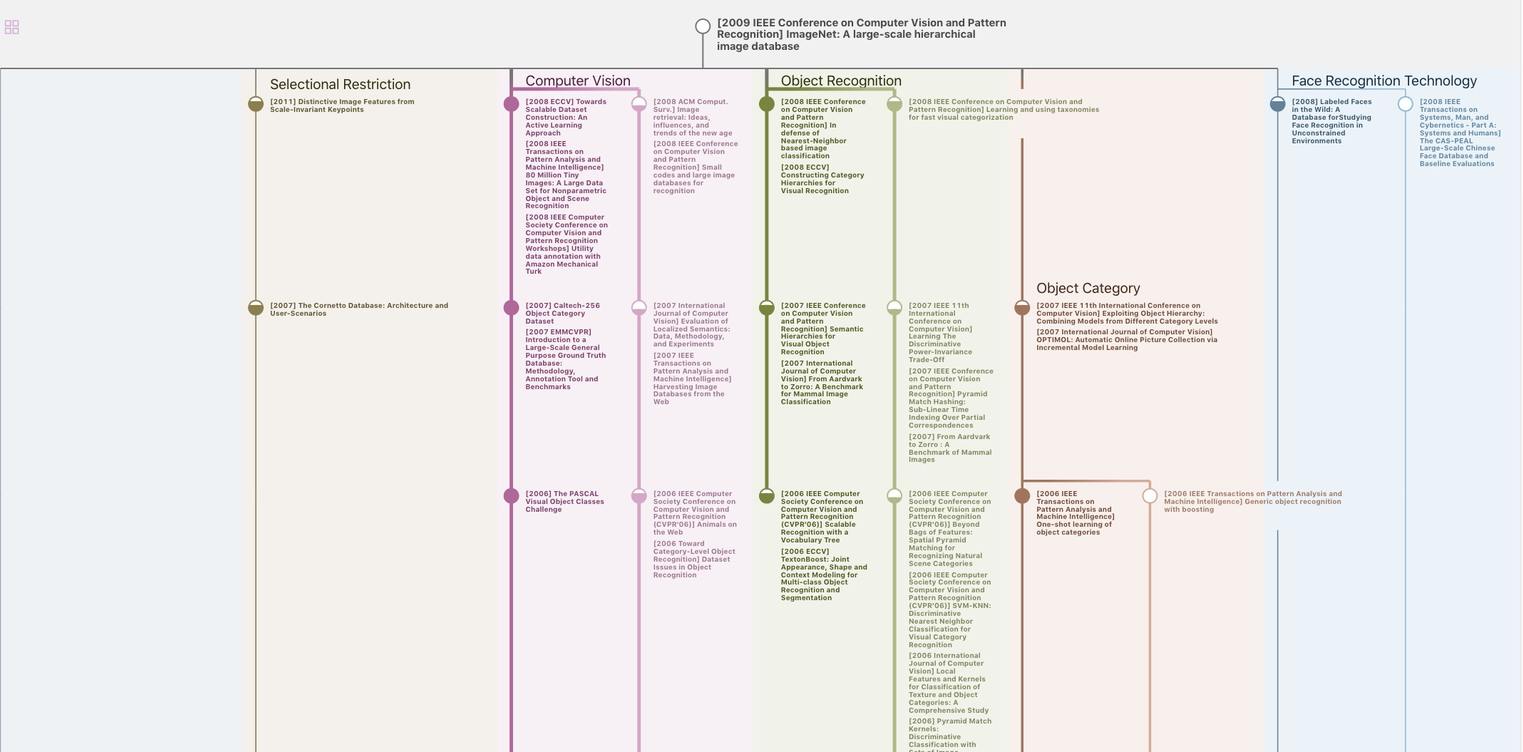
生成溯源树,研究论文发展脉络
Chat Paper
正在生成论文摘要