Analysis, Classification And Marker Discovery Of Gene Expression Data With Evolving Spiking Neural Networks
NEURAL INFORMATION PROCESSING (ICONIP 2018), PT V(2018)
摘要
The paper presents a methodology to assess the problems behind static gene expression data modelling and analysis with machine learning techniques. As a case study, transcriptomic data collected during a longitudinal study on the effects of diet on the expression of oxidative phosphorylation genes was used. Data were collected from 60 abdominally overweight men and women after an observation period of eight weeks, whilst they were following three different diets. Real-valued static gene expression data were encoded into spike trains using Gaussian receptive fields for multinomial classification using an evolving spiking neural network (eSNN) model. Results demonstrated that the proposed method can be used for predictive modelling of static gene expression data and future works are proposed regarding the application of eSNNs for personalised modelling.
更多查看译文
关键词
Evolving spiking neural networks, Gaussian receptive fields, Static data, Gene expression, Microarray, Transcriptome data analysis
AI 理解论文
溯源树
样例
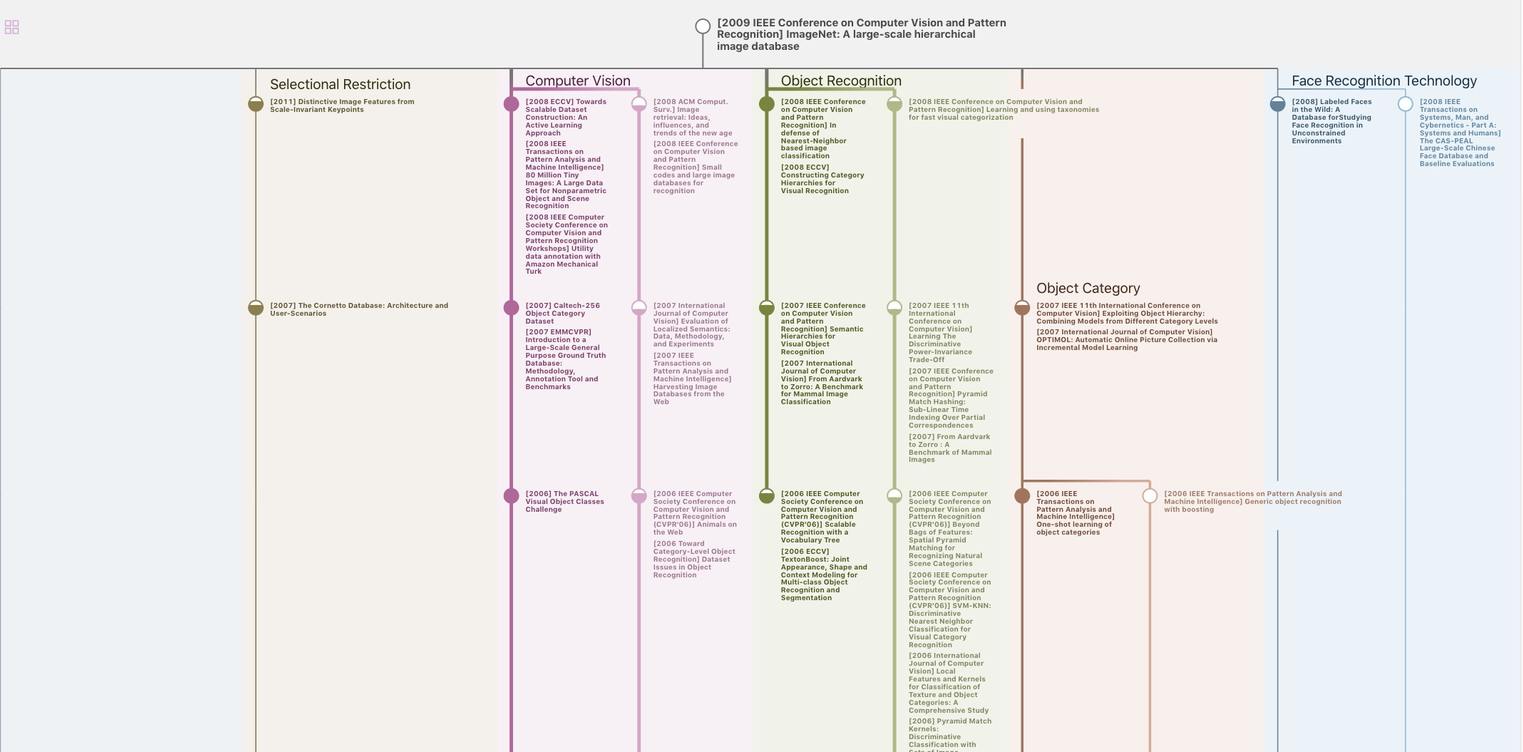
生成溯源树,研究论文发展脉络
Chat Paper
正在生成论文摘要