Adversarial Training for Video Disentangled Representation.
MMM(2019)
摘要
The strong demand for video analytics is largely due to the widespread application of CCTV. Perfectly encoding moving objects and scenes with different sizes and complexity in an unsupervised manner is still a challenge and seriously affects the quality of video prediction and subsequent analysis. In this paper, we introduce adversarial training to improve DrNet which disentangles a video with stationary scene and moving object representations, while taking the tiny objects and complex scene into account. These representations can be used for subsequent industrial applications such as vehicle density estimation, video retrieval, etc. Our experiment on LASIESTA database confirms the validity of this method in both reconstruction and prediction performance. Meanwhile, we propose an experiment that vanishes one of the codes and reconstructs the images by concatenating these zero and non-zero codes. This experiment separately evaluates the moving object and scene coding quality and shows that the adversarial training achieves a significant reconstruction quality in visual effect, despite of complex scene and tiny object.
更多查看译文
关键词
video disentangled representation,training
AI 理解论文
溯源树
样例
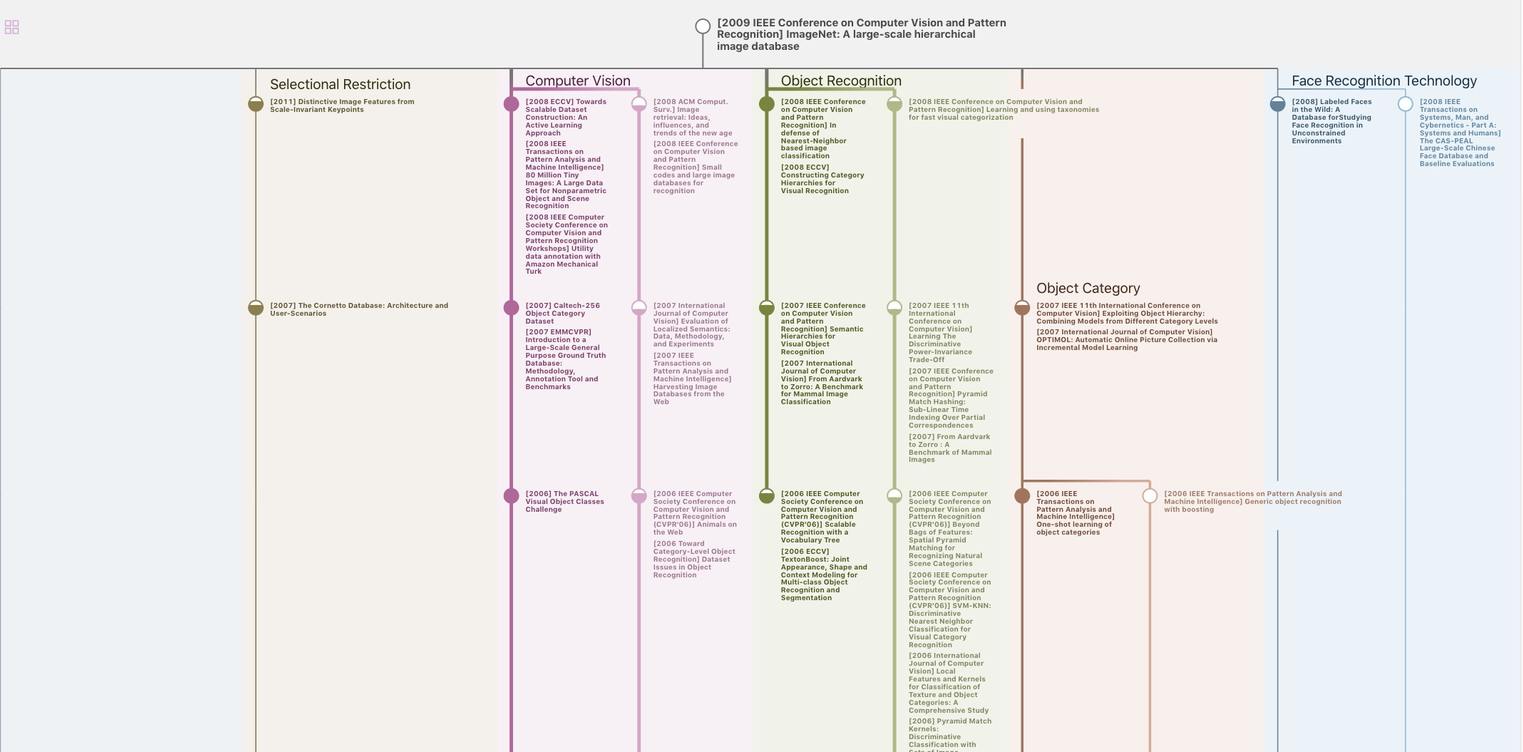
生成溯源树,研究论文发展脉络
Chat Paper
正在生成论文摘要