Robust Local Learning And Discriminative Concept Factorization For Data Representation
IEEE ACCESS(2018)
摘要
Concept factorization (CF), as a matrix factorization method, has been applied widely in obtaining an optimal data representation and has yielded impressive results. However, some shortcomings exist in the existing CF method. 1) The standard concept factorization uses the squared loss function that is sensitive to outlier points and noises. 2) The graph generated by the original data does not reflect the real geometric structure of the data distribution. 3) The discriminant information is ignored. Herein, we propose a novel method, called robust local learning and discriminative concept factorization (RLLDCF) for data representation. Specifically, RLLDCF adopts the ,l(2,1)-norm-based loss function to improve its robustness against noises and outliers, and exploits the discriminative information by local linear regression constraints. In addition, the method obtains the topology structure of the data distribution during learning rather than known a priori and fixed. A new iterative multiplicative updating rule is derived to solve RLLDCF's objective function. The convergence of the optimization algorithm is proved both theoretically and empirically. Numerous experiments on both synthetic and real-world datasets are conducted, and the results indicate that our proposed method is significantly better than all the comparison methods, thus validating the effectiveness and robustness of RLLDCF.
更多查看译文
关键词
Clustering, data representation, l(2,1)-norm, local adaptive learning, local linear regression
AI 理解论文
溯源树
样例
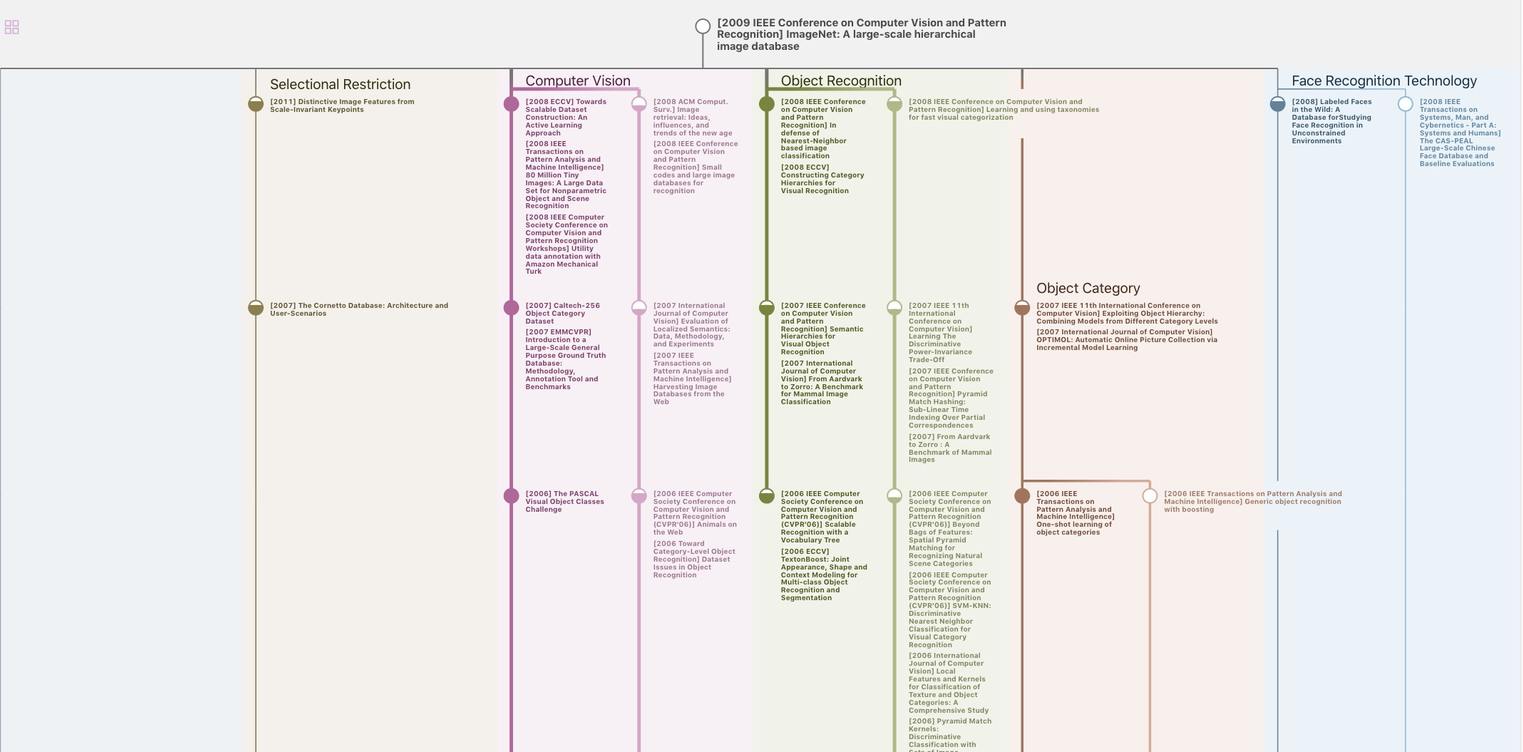
生成溯源树,研究论文发展脉络
Chat Paper
正在生成论文摘要