Sparse Bayesian Learning-Based Target Imaging and Parameter Estimation for Monostatic MIMO Radar Systems.
IEEE ACCESS(2018)
摘要
This paper presents novel sparse Bayesian learning (SBL)-based target imaging and parameter estimation techniques in monostatic multiple-input multiple-output (MIMO) radar systems for practical scenarios with insufficient observation samples and unknown target parameters. First, the SBL framework is developed for a single measurement vector setting with an underlying sparse target reflectivity parameter vector. This is subsequently extended to scenarios with multiple observation snapshots considering uncorrelated as well as correlated target reflectivity parameters. Variants are also proposed for challenging scenarios considering the presence of ground clutter. Cramer-Rao bounds are derived for the reflectivity, Doppler, and range estimates to comprehensively characterize the performance of the proposed estimation schemes. A joint parameter estimation and imaging scheme is developed based on a Taylor series expansion of the MIMO radar dictionary matrix. Simulation results demonstrate enhanced imaging and estimation accuracy of the proposed SBL schemes in comparison with the existing techniques for MIMO radar systems.
更多查看译文
关键词
Monostatic MIMO radar,sparse Bayesian learning,target imaging,parameter estimation,Cramer-Rao bound
AI 理解论文
溯源树
样例
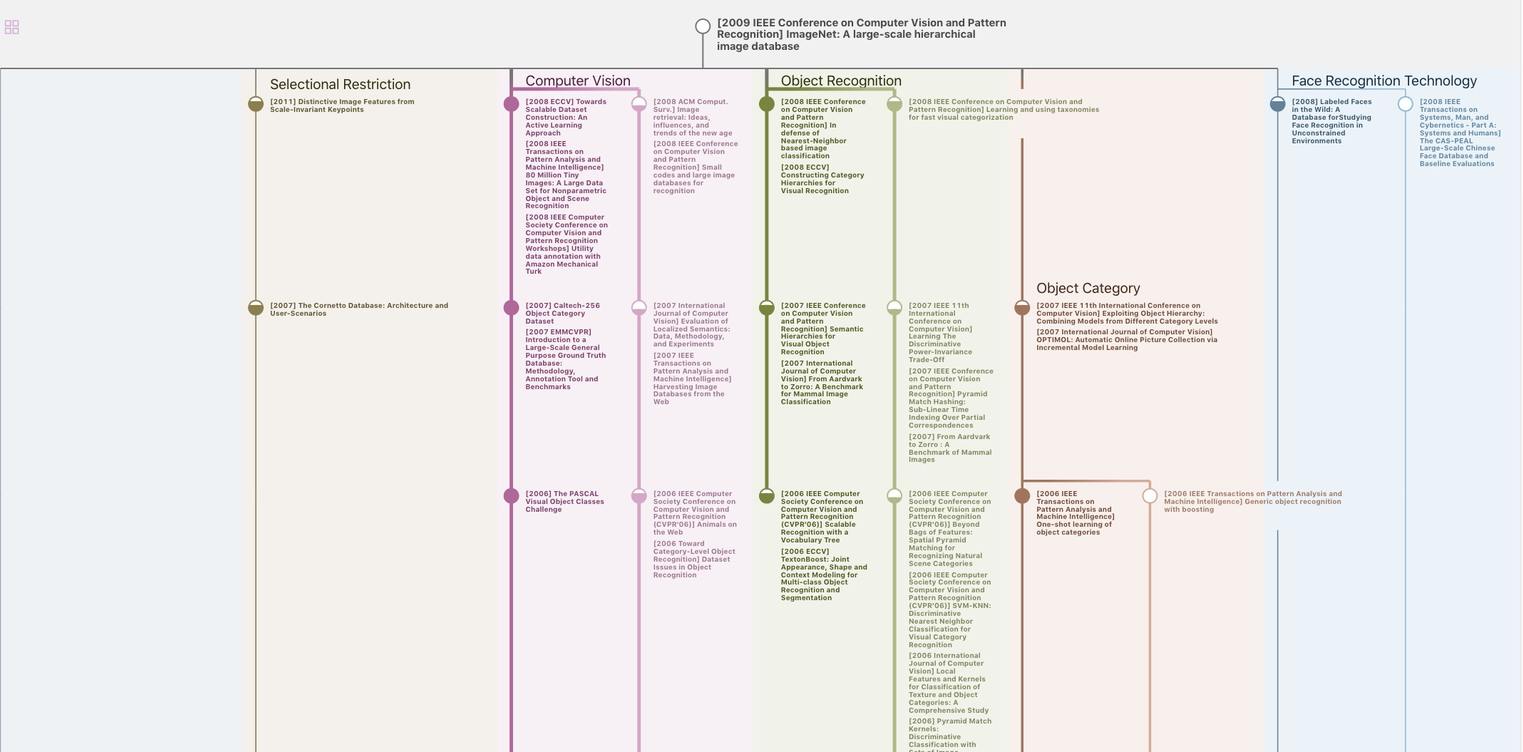
生成溯源树,研究论文发展脉络
Chat Paper
正在生成论文摘要