On the Power of Preprocessing in Decentralized Network Optimization.
IEEE INFOCOM 2019 - IEEE Conference on Computer Communications(2019)
摘要
As communication networks are growing at a fast pace, the need for more scalable approaches to operate such networks is pressing. Decentralization and locality are key concepts to provide scalability. Existing models for which local algorithms are designed fail to model an important aspect of many modern communication networks such as software-defined networks: the possibility to precompute distributed network state. We take this as an opportunity to study the fundamental question of how and to what extent local algorithms can benefit from preprocessing. In particular, we show that preprocessing allows for significant speedups of various networking problems. A main benefit is the precomputation of structural primitives, where purely distributed algorithms have to start from scratch. Maybe surprisingly, we also show that there are strict limitations on how much preprocessing can help in different scenarios. To this end, we provide approximation bounds for the maximum independent set problem—which however show that our obtained speedups are asymptotically optimal. Even though we show that physical link failures in general hinder the power of preprocessing, we can still facilitate the precomputation of symmetry breaking processes to bypass various runtime barriers. We believe that our model and results are of interest beyond the scope of this paper and apply to other dynamic networks as well.
更多查看译文
关键词
Computational modeling,Optimization,Network topology,Approximation algorithms,Topology,Communication networks,Scalability
AI 理解论文
溯源树
样例
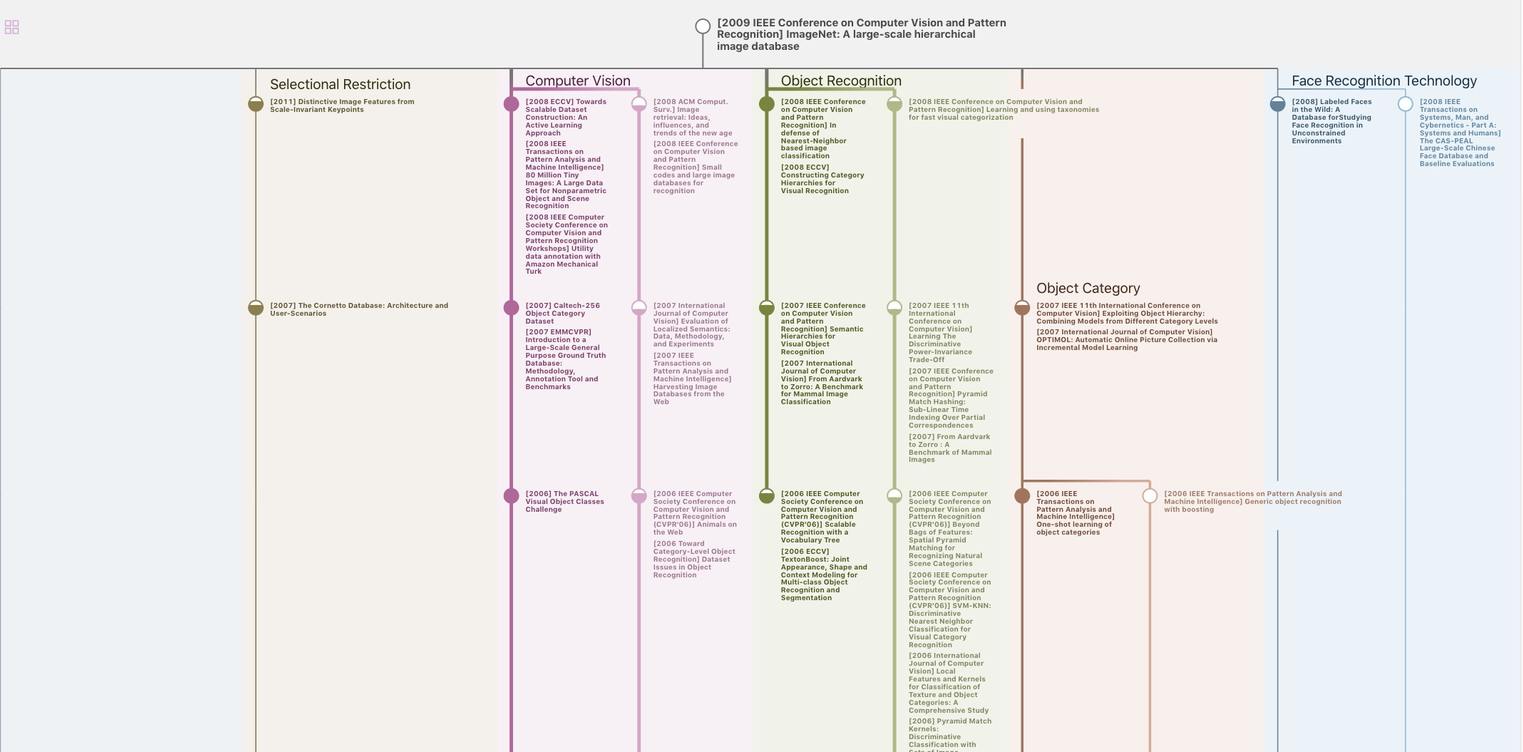
生成溯源树,研究论文发展脉络
Chat Paper
正在生成论文摘要