Towards Robust Human Activity Recognition from RGB Video Stream with Limited Labeled Data
2018 17th IEEE International Conference on Machine Learning and Applications (ICMLA)(2018)
摘要
Human activity recognition based on video streams has received numerous attentions in recent years. Due to lack of depth information, RGB video based activity recognition performs poorly compared to RGB-D video based solutions. On the other hand, acquiring depth information, inertia etc. is costly and requires special equipment, whereas RGB video streams are available in ordinary cameras. Hence, our goal is to investigate whether similar or even higher accuracy can be achieved with RGB-only modality. In this regard, we propose a novel framework that couples skeleton data extracted from RGB video and deep Bidirectional Long Short Term Memory (BLSTM) model for activity recognition. A big challenge of training such a deep network is the limited training data, and exploring RGB-only stream significantly exaggerates the difficulty. We therefore propose a set of algorithmic techniques to train this model effectively, e.g., data augmentation, dynamic frame dropout and gradient injection. The experiments demonstrate that our RGB-only solution surpasses the state-of-the-art approaches that all exploit RGB-D video streams by a notable margin. This makes our solution widely deployable with ordinary cameras.
更多查看译文
关键词
Computer Vision,Deep Learning,LSTM,Limited Data,RGB,Human Activity Recognition
AI 理解论文
溯源树
样例
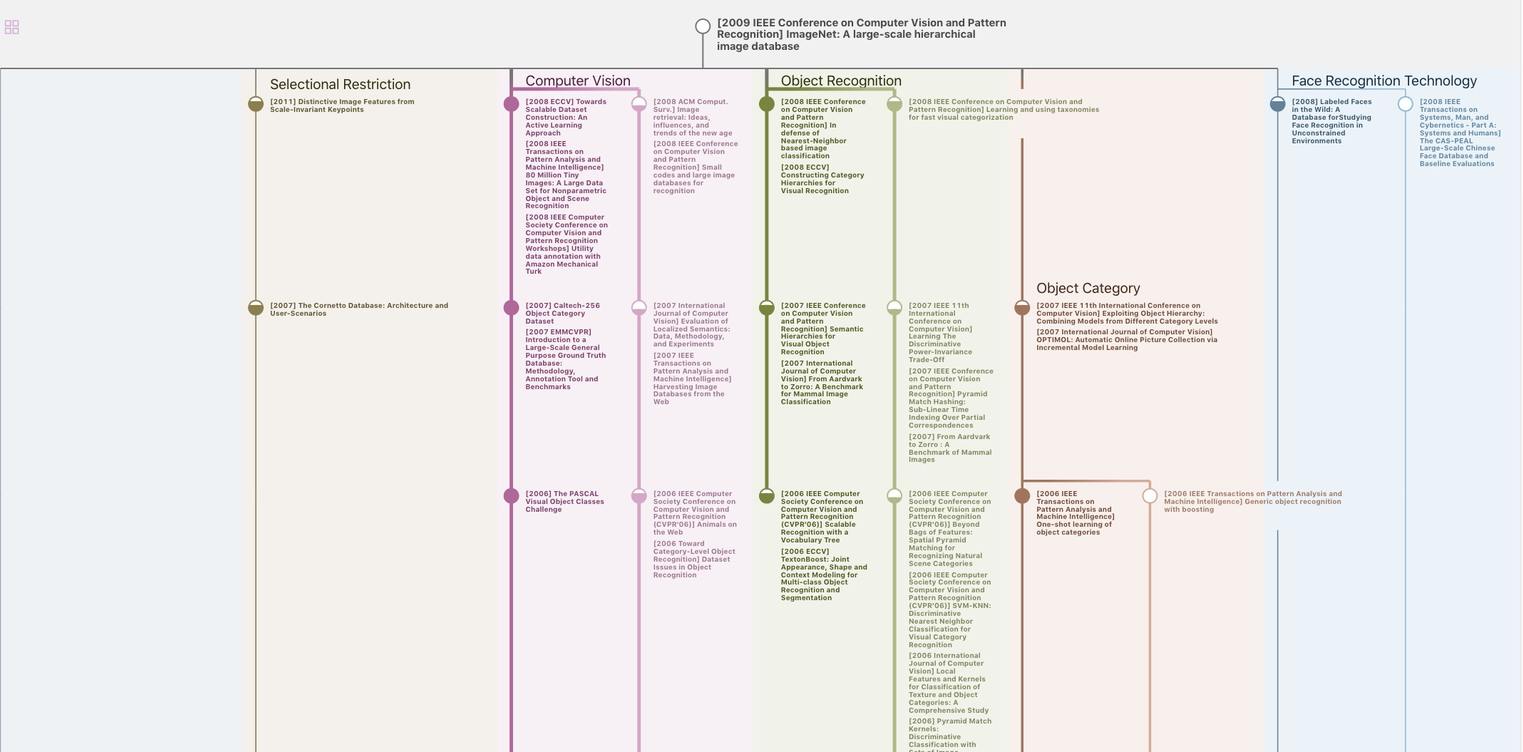
生成溯源树,研究论文发展脉络
Chat Paper
正在生成论文摘要