To Reverse the Gradient or Not: An Empirical Comparison of Adversarial and Multi-task Learning in Speech Recognition
ICASSP 2019 - 2019 IEEE International Conference on Acoustics, Speech and Signal Processing (ICASSP)(2019)
摘要
Transcribed datasets typically contain speaker identity for each instance in the data. We investigate two ways to incorporate this information during training: Multi-Task Learning and Adversarial Learning. In multi-task learning, the goal is speaker prediction; we expect a performance improvement with this joint training if the two tasks of speech recognition and speaker recognition share a common set of underlying features. In contrast, adversarial learning is a means to learn representations invariant to the speaker. We then expect better performance if this learnt invariance helps generalizing to new speakers. While the two approaches seem natural in the context of speech recognition, they are incompatible because they correspond to opposite gradients back-propagated to the model. In order to better understand the effect of these approaches in terms of error rates, we compare both strategies in controlled settings. Moreover, we explore the use of additional untranscribed data in a semi-supervised, adversarial learning manner to improve error rates. Our results show that deep models trained on big datasets already develop invariant representations to speakers without any auxiliary loss. When considering adversarial learning and multi-task learning, the impact on the acoustic model seems minor. However, models trained in a semi-supervised manner can improve error-rates.
更多查看译文
关键词
automatic speech recognition,adversarial learning,multi-task learning,neural networks
AI 理解论文
溯源树
样例
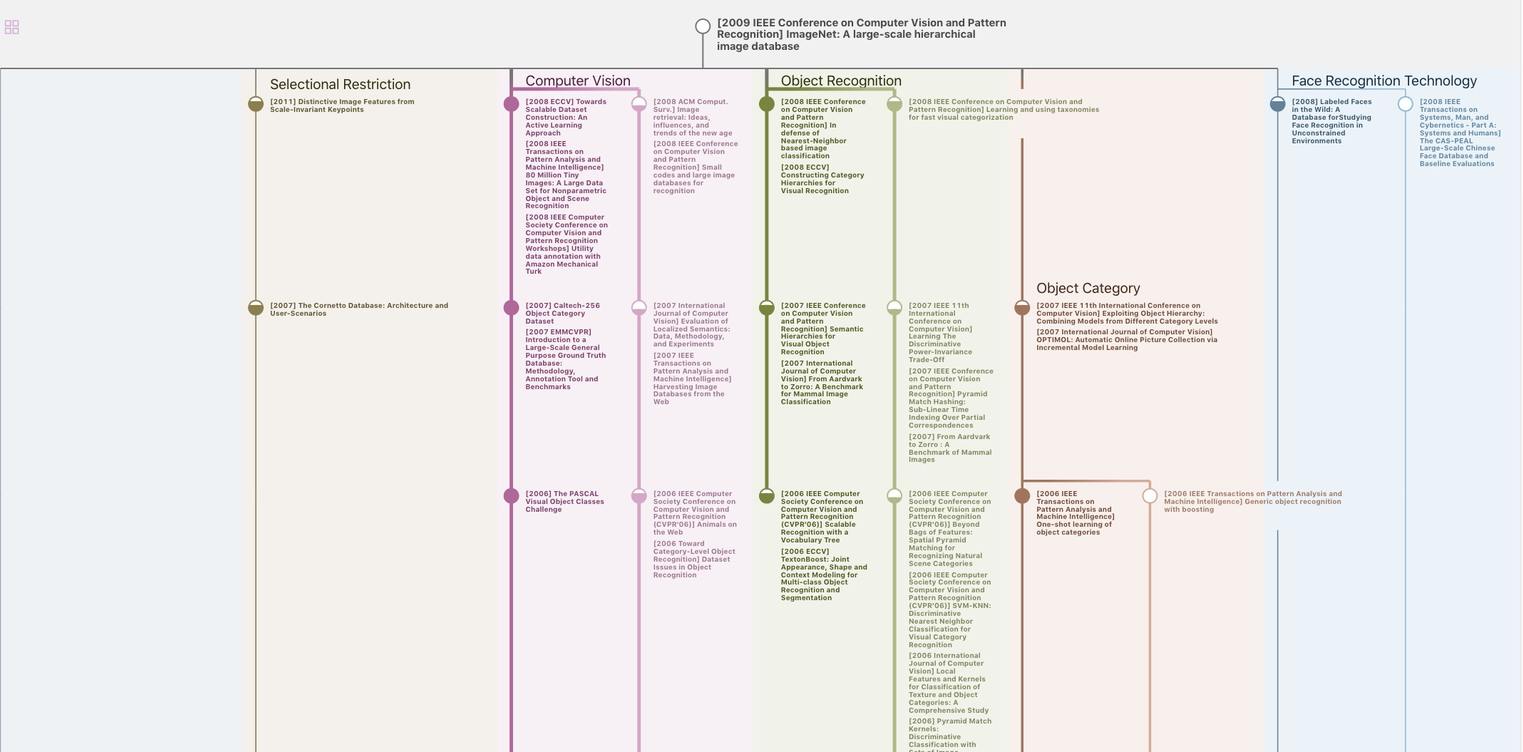
生成溯源树,研究论文发展脉络
Chat Paper
正在生成论文摘要