A case study : Influence of Dimension Reduction on regression trees-based Algorithms -Predicting Aeronautics Loads of a Derivative Aircraft.
arXiv: Learning(2018)
摘要
In aircraft industry, market needs evolve quickly in a high competitiveness context. This requires adapting a given aircraft model in minimum time considering for example an increase of range or the number of passengers (cf A330 NEO family). The computation of loads and stress to resize the airframe is on the critical path of this aircraft variant definition: this is a consuming and costly process, one of the reason being the high dimen-sionality and the large amount of data. This is why Airbus has invested since a couple of years in Big Data approaches (statistic methods up to machine learning) to improve the speed, the data value extraction and the responsiveness of this process. This paper presents recent advances in this work made in cooperation between Airbus, ENAC and Institut de Mathe-matiques de Toulouse in the framework of a proof of value sprint project. It compares the influence of three dimensional reduction techniques (PCA, polynomial fitting, combined) on the extrapolation capabilities of Regression Trees based algorithms for loads prediction. It shows that AdaBoost with Random Forest offers promising results in average in terms of accuracy and computational time to estimate loads on which a PCA is applied only on the outputs.
更多查看译文
关键词
Regression trees, Aeronautics, Dimensional reduction, Extrapolation
AI 理解论文
溯源树
样例
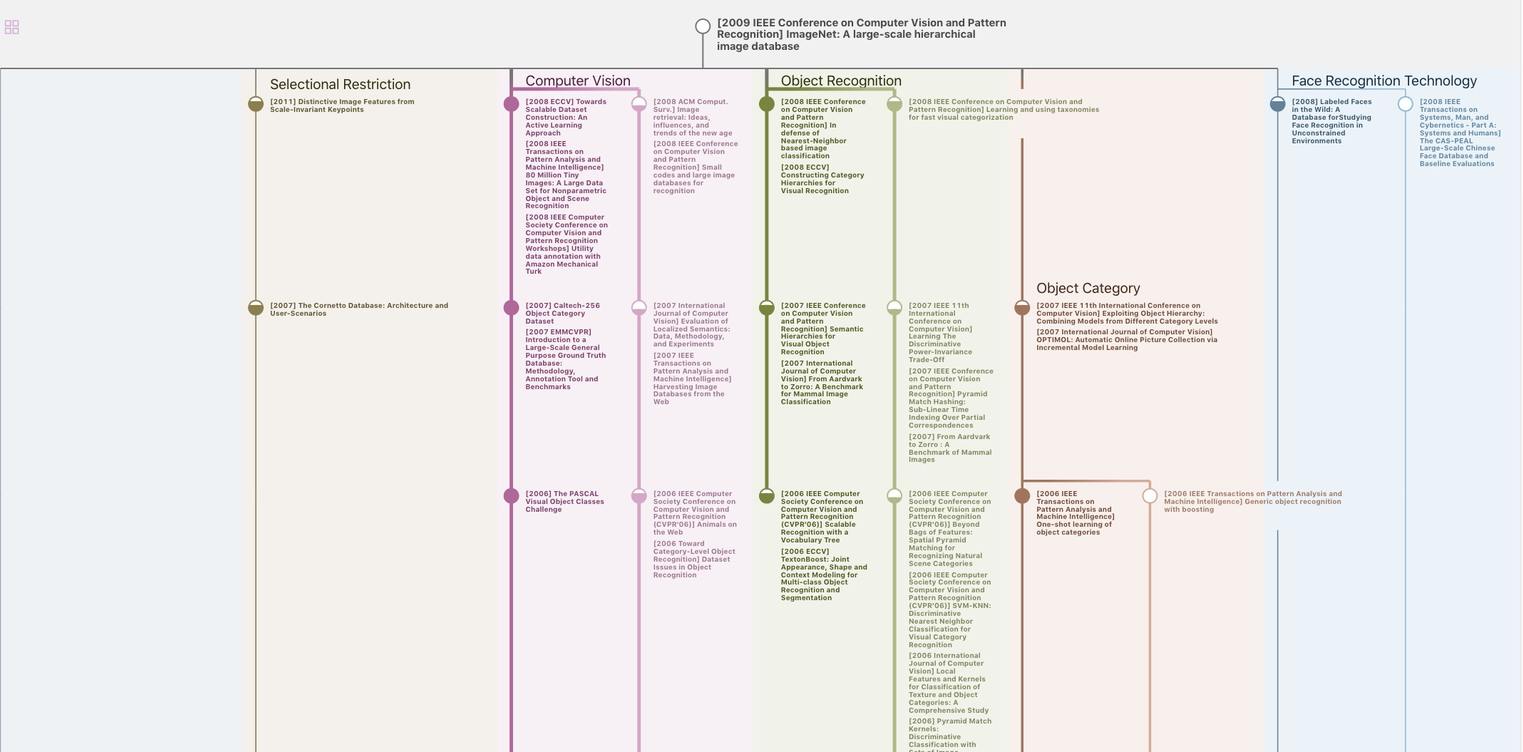
生成溯源树,研究论文发展脉络
Chat Paper
正在生成论文摘要