A Feature-Aware Online Learning Approach for Support Vector Machine Classification
2018 15th International Conference on Control, Automation, Robotics and Vision (ICARCV)(2018)
摘要
Online machine learning algorithm has attracted increasing attention especially in the era of Industry 4.0. The reason is that traditional batch learning algorithm cannot deal with the streaming data produced by sensorized machines and make real-time decisions. In this paper, we propose a Feature-aware online learning approach of Support Vector Machine (FSVM) for classification problem. Usually, online learning algorithm has limited access to streaming data due to resource and computation constraints. In FSVM, we introduced a feature vector selection method to reduce the size of training dataset without losing key information and maintain an acceptable classification accuracy. Here, such small set of selected feature vectors is able to represent the original dataset. What is more, we can detect feature drifting by checking whether or not a new input data can be represented by the current feature vectors. We evaluate the performance of FSVM based on several realworld datasets. The results show that even train the SVM model with around 10% data, an acceptable misclassification rate can be reached.
更多查看译文
关键词
resource,computation constraints,FSVM,feature vector selection method,training dataset,acceptable classification accuracy,input data,Support Vector Machine classification,online machine learning algorithm,sensorized machines,real-time decisions,Industry 4.0,feature-aware online learning approach,feature vectors,data streaming
AI 理解论文
溯源树
样例
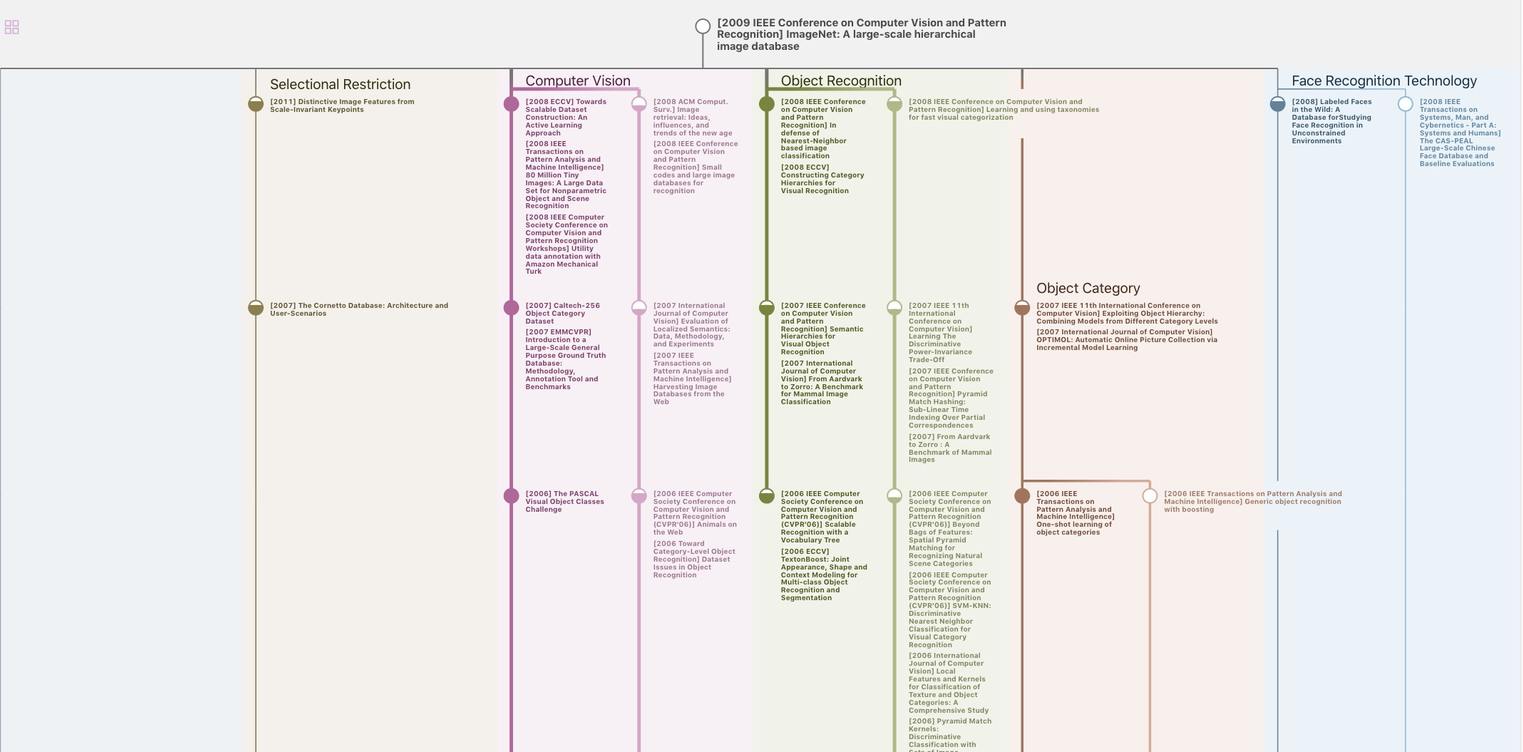
生成溯源树,研究论文发展脉络
Chat Paper
正在生成论文摘要