Detection of False Data Injection Attacks in Smart Grids Based on Forecasts
2018 IEEE International Conference on Communications, Control, and Computing Technologies for Smart Grids (SmartGridComm)(2018)
摘要
The bi-directional communication capabilities that emerged into the smart power grid play a critical role in the grid's secure, reliable and efficient operation. Nevertheless, the data communication functionalities introduced to Advanced Metering Infrastructure (AMI) nodes end the grid's isolation, and expose the network into an array of cyber-security threats that jeopardize the grid's stability and availability. For instance, malware amenable to inject false data into the AMI can compromise the grid's state estimation process and lead to catastrophic power outages. In this paper, we explore several statistical spatio-temporal models for efficient diagnosis of false data injection attacks in smart grids. The proposed methods leverage the data co-linearities that naturally arise in the AMI measurements of the electric network to provide forecasts for the network's AMI observations, aiming to quickly detect the presence of “bad data”. We evaluate the proposed approaches with data tampered with stealth attacks compiled via three different attack strategies. Further, we juxtapose them against two other forecasting-aided detection methods appearing in the literature, and discuss the trade-offs of all techniques when employed on real-world power grid data, obtained from a large university campus.
更多查看译文
关键词
grid state estimation process,grid stability,statistical spatiotemporal models,bidirectional communication capabilities,power grid data,forecasting-aided detection methods,stealth attacks,AMI measurements,catastrophic power outages,cyber-security threats,Advanced Metering Infrastructure,data communication functionalities,smart power grid,smart grids,false data injection attacks
AI 理解论文
溯源树
样例
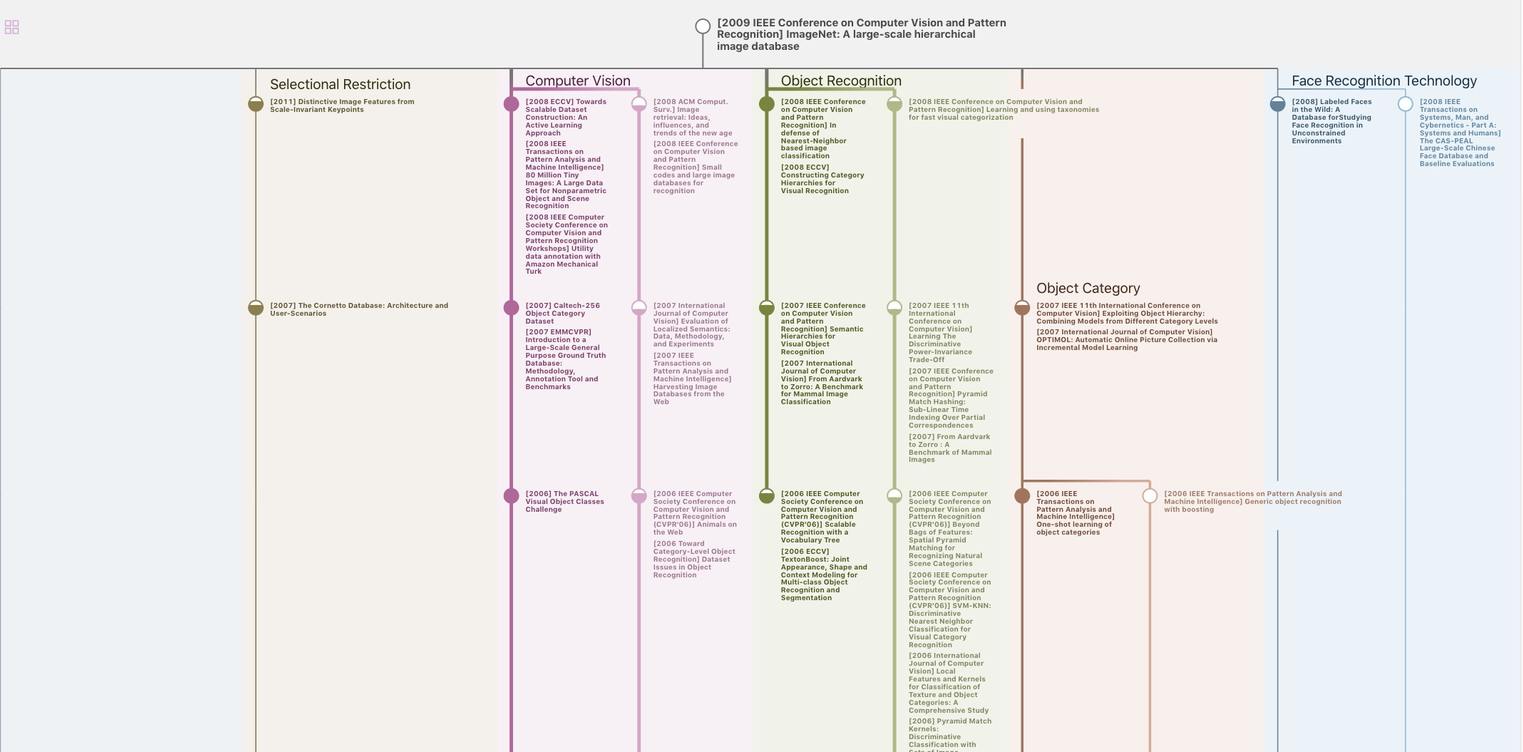
生成溯源树,研究论文发展脉络
Chat Paper
正在生成论文摘要