Forecasting Wavelet Transformed Time Series With Attentive Neural Networks
2018 IEEE INTERNATIONAL CONFERENCE ON DATA MINING (ICDM)(2018)
摘要
This paper studies the problem of time series fore-casting. A time series is defined as a sequence of data points listed in time order. Many real-life time series data are driven by multiple latent components which occur at different frequencies. Existing solutions to time series forecasting fail to identify and discriminate these frequency-domain components. Inspired by the recent advent of signal processing and speech recognition techniques that decompose a time series signal into its time-frequency representation - a scalogram (or spectrogram), this paper proposes to explicitly disclose frequency-domain information from a univariate time series using wavelet transform, towards improving forecasting accuracy. Based on the transformed data, we leverage different neural networks to capture local time-frequency features and global long-term trend simultaneously. We further employ the attention mechanism to fuse local and global features in an effective manner. The experimental results on real time series show that our proposed approach achieves better performance than various baseline methods.
更多查看译文
关键词
Time series forecasting, wavelet transform, attentive neural networks
AI 理解论文
溯源树
样例
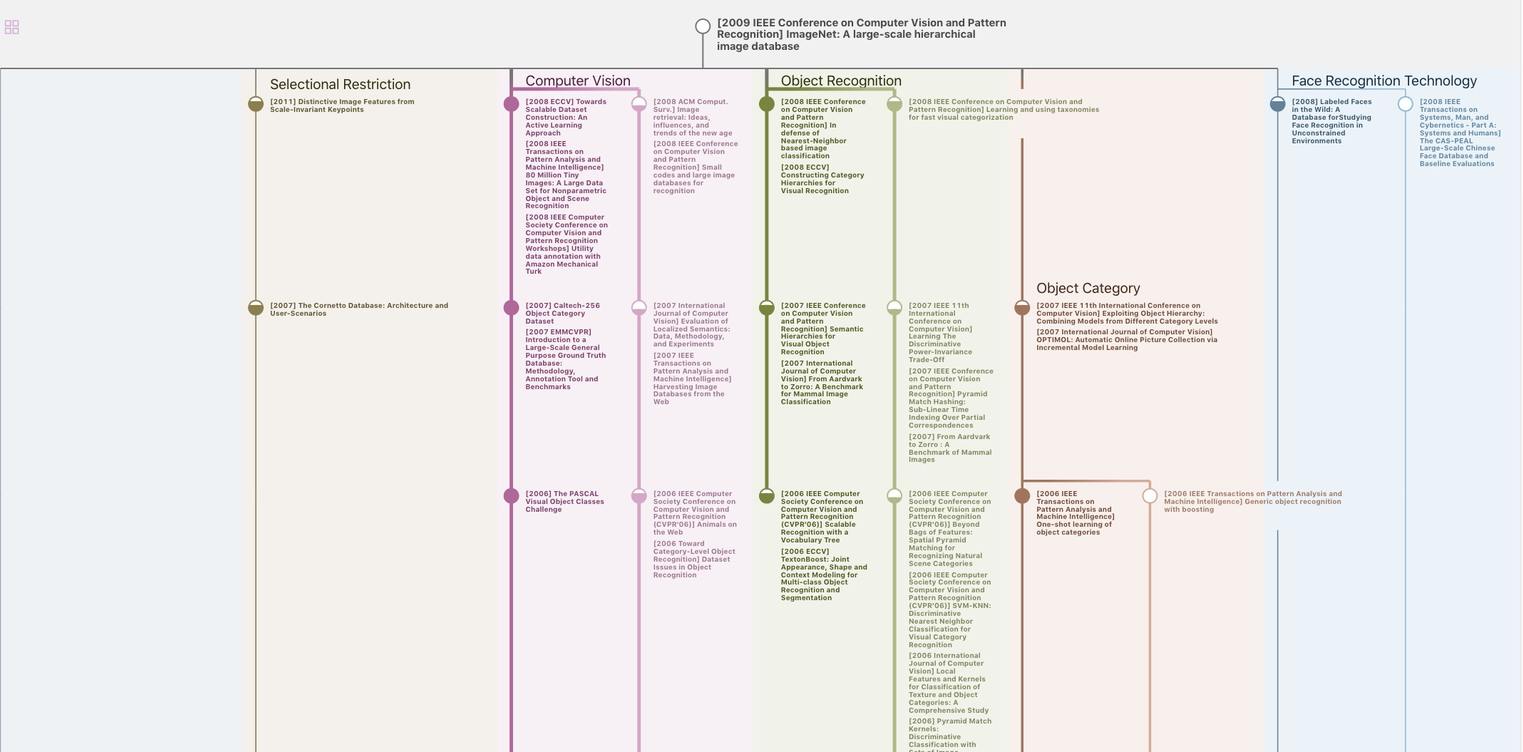
生成溯源树,研究论文发展脉络
Chat Paper
正在生成论文摘要