Predicting adverse drug reactions through interpretable deep learning framework
BMC Bioinformatics(2018)
摘要
Background Adverse drug reactions (ADRs) are unintended and harmful reactions caused by normal uses of drugs. Predicting and preventing ADRs in the early stage of the drug development pipeline can help to enhance drug safety and reduce financial costs. Methods In this paper, we developed machine learning models including a deep learning framework which can simultaneously predict ADRs and identify the molecular substructures associated with those ADRs without defining the substructures a-priori . Results We evaluated the performance of our model with ten different state-of-the-art fingerprint models and found that neural fingerprints from the deep learning model outperformed all other methods in predicting ADRs. Via feature analysis on drug structures, we identified important molecular substructures that are associated with specific ADRs and assessed their associations via statistical analysis. Conclusions The deep learning model with feature analysis, substructure identification, and statistical assessment provides a promising solution for identifying risky components within molecular structures and can potentially help to improve drug safety evaluation.
更多查看译文
关键词
Chemical fingerprint,Adverse drug reaction,Deep learning
AI 理解论文
溯源树
样例
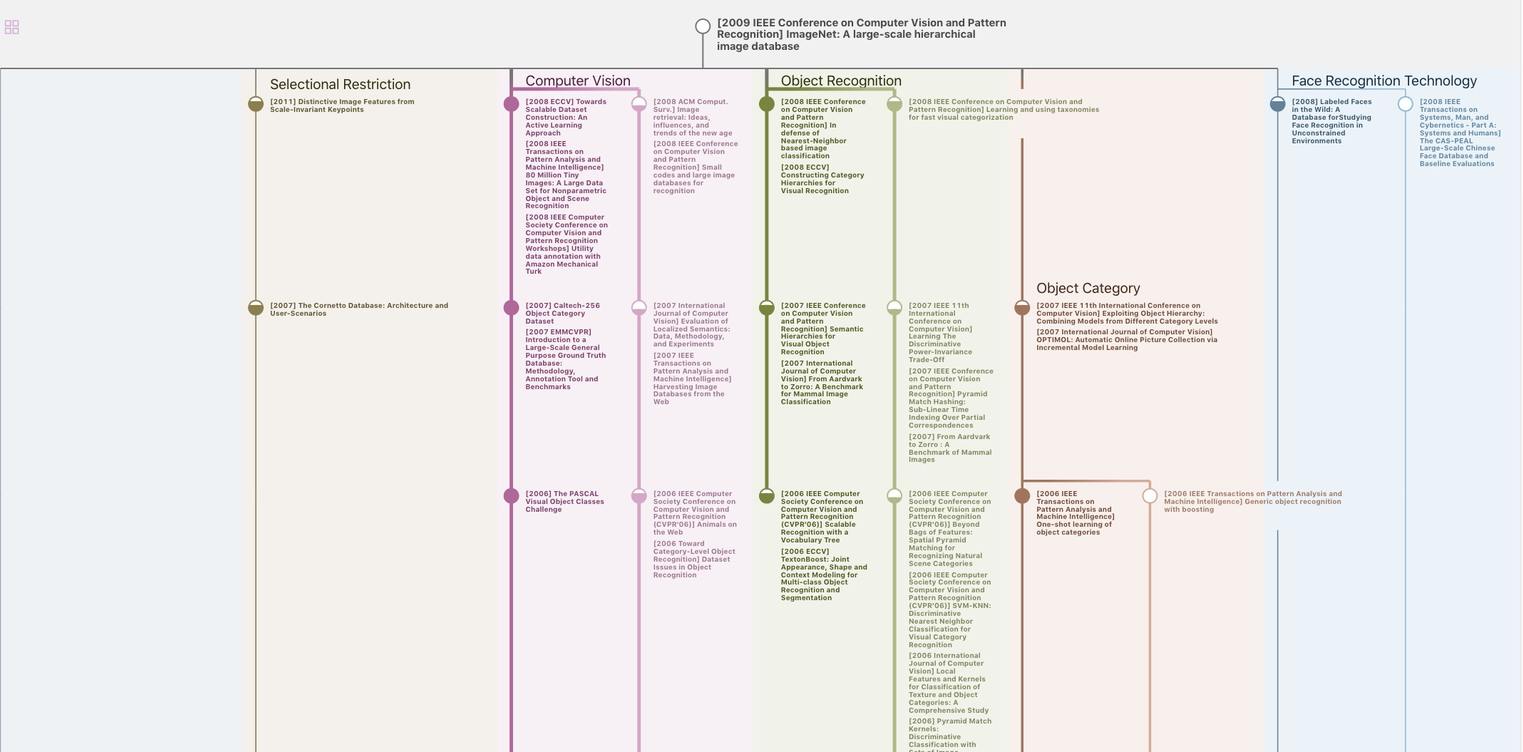
生成溯源树,研究论文发展脉络
Chat Paper
正在生成论文摘要