An adaptive RBF-HDMR modeling approach under limited computational budget
Structural and Multidisciplinary Optimization(2017)
摘要
The metamodel-based high-dimensional model representation (e.g., RBF-HDMR) has recently been proven to be very promising for modeling high dimensional functions. A frequently encountered scenario in practical engineering problems is the need of building accurate models under limited computational budget. In this context, the original RBF-HDMR approach may be intractable due to the independent and successive treatment of the component functions, which translates in a lack of knowledge on when the modeling process will stop and how many points (simulations) it will cost. This article proposes an adaptive and tractable RBF-HDMR (ARBF-HDMR) modeling framework. Given a total of N m a x points, it first uses N i n i points to build an initial RBF-HDMR model for capturing the characteristics of the target function f , and then keeps adaptively identifying, sampling and modeling the potential cuts with the remaining N m a x − N i n i points. For the second-order ARBF-HDMR, N i n i ∈ [2 n + 2,2 n 2 + 2] not only depends on the dimensionality n but also on the characteristics of f . Numerical results on nine cases with up to 30 dimensions reveal that the proposed approach provides more accurate predictions than the original RBF-HDMR with the same computational budget, and the version that uses the maximin sampling criterion and the best-model strategy is a recommended choice. Moreover, the second-order ARBF-HDMR model significantly outperforms the first-order model; however, if the computational budget is strictly limited (e.g., 2 n + 1 < N m a x ≪ 2 n 2 + 2), the first-order model becomes a better choice. Finally, it is noteworthy that the proposed modeling framework can work with other metamodeling techniques.
更多查看译文
关键词
Metamodeling,Adaptive high dimensional model representation,Limited computational budget,Tractable process
AI 理解论文
溯源树
样例
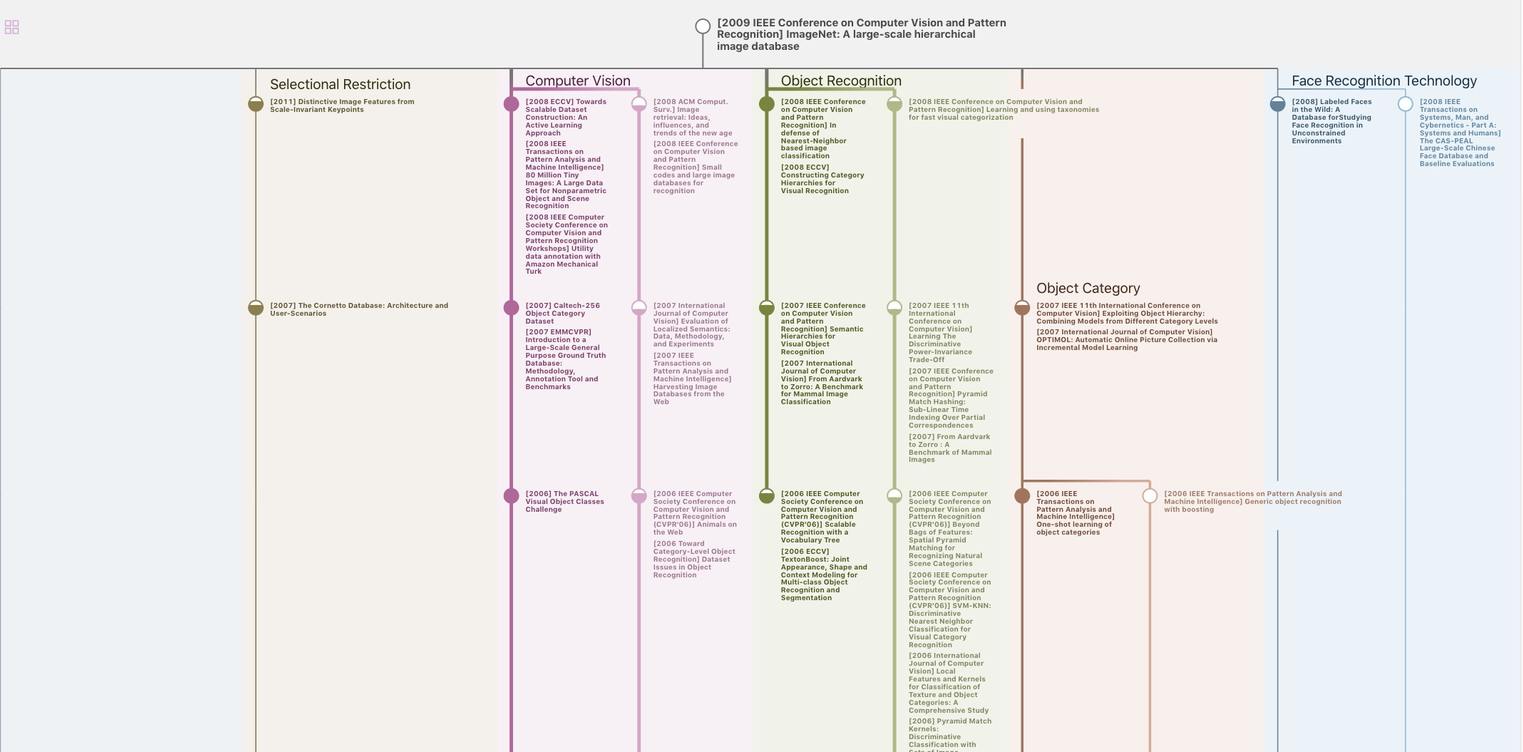
生成溯源树,研究论文发展脉络
Chat Paper
正在生成论文摘要