Boosting the Deep Multidimensional Long-Short-Term Memory Network for Handwritten Recognition Systems
2018 16th International Conference on Frontiers in Handwriting Recognition (ICFHR)(2018)
摘要
One of the main challenges in the handwriting recognition area lies in identifying complete lines of handwritten text. In this paper, we propose a handwriting recognition system based on a deep multidimensional long-short-term memory (MDLSTM) network within a hybrid hidden Markov model framework. The MDLSTM architecture was elaborated to enhance the recognition performance and decrease the recognition time. Accordingly, we present modifications regarding the layers order and the number of pooling layers compared to a standard MDLSTM model. Since the results reported in the literature for deeper MDLSTM architectures relies on optimizing the network width with a fixed depth, we investigate the trade-off between both these properties to obtain an optimal topology. The system was evaluated with English handwritten text lines from the IAM database and the experiments demonstrated that the proposed MDLSTM architecture was able to maintain a robust recognition performance (around 3.6% CER and 10.5% WER) and present significant speedups, approximately 48% and 32% faster than the state-of-the-art MDLSTM optical model, regarding the learning and classification times, respectively. The full system including a decoder with linguistic knowledge presents competitive results with the state-of-the-art.
更多查看译文
关键词
MDLSTM,handwriting recognition,recurrent neural networks
AI 理解论文
溯源树
样例
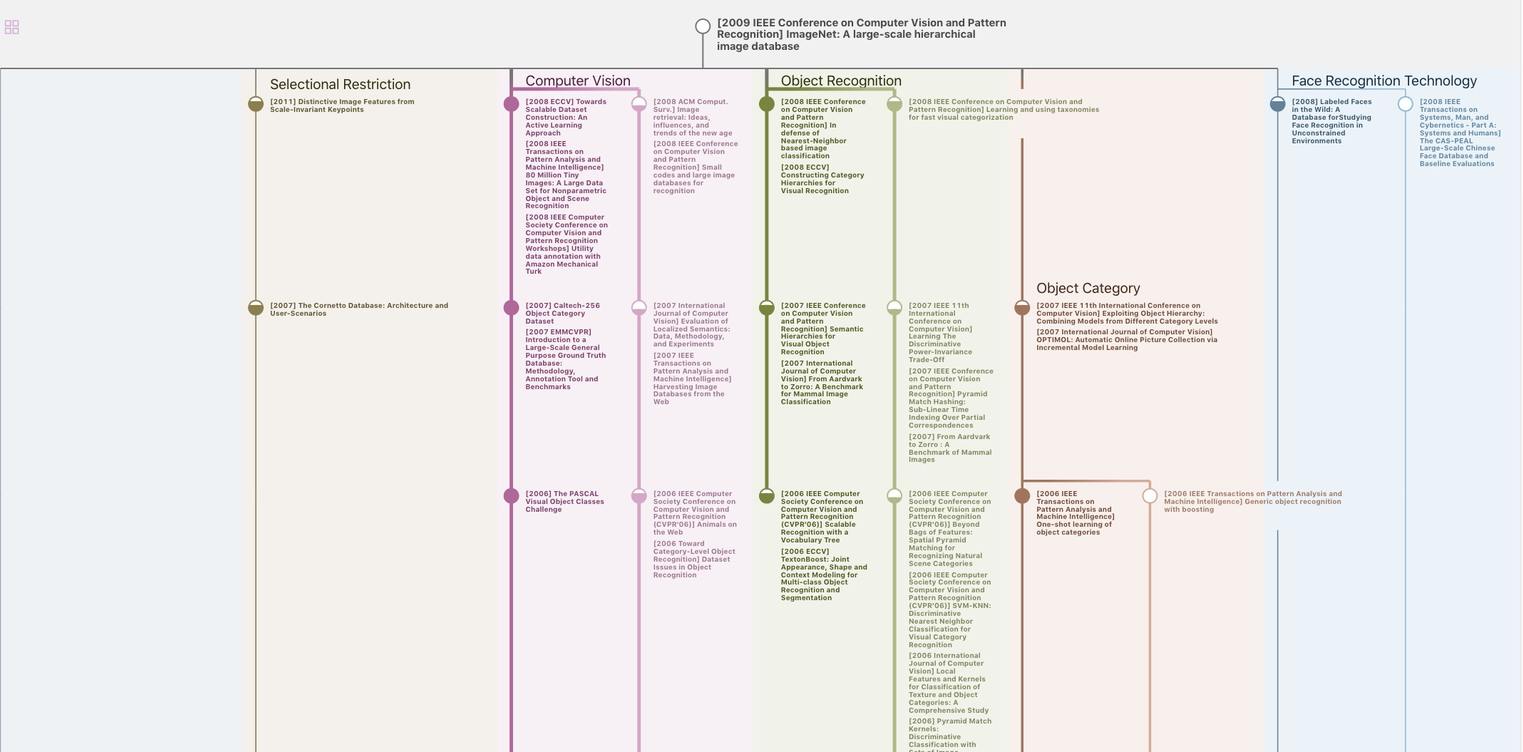
生成溯源树,研究论文发展脉络
Chat Paper
正在生成论文摘要