Separating Optical and Language Models Through Encoder-Decoder Strategy for Transferable Handwriting Recognition
2018 16th International Conference on Frontiers in Handwriting Recognition (ICFHR)(2018)
摘要
Lack of data can be an issue when beginning a new study on historical handwritten documents. To deal with this, we propose a deep-learning based recognizer which separates the optical and the language models in order to train them separately using different resources. In this work, we present the optical encoder part of a multilingual transductive transfer learning applied to historical handwriting recognition. The optical encoder transforms the input word image into a non-latent space that depends only on the letter-n-grams: it enables it to be independent of the language. This transformation avoids embedding a language model and operating the transfer learning across languages using the same alphabet. The language decoder creates from a vector of letter-n-grams a word as a sequence of characters. Experiments show that separating optical and language model can be a solution for multilingual transfer learning.
更多查看译文
关键词
Handwriting recognition, knowledge transfer, Optical model, Language model
AI 理解论文
溯源树
样例
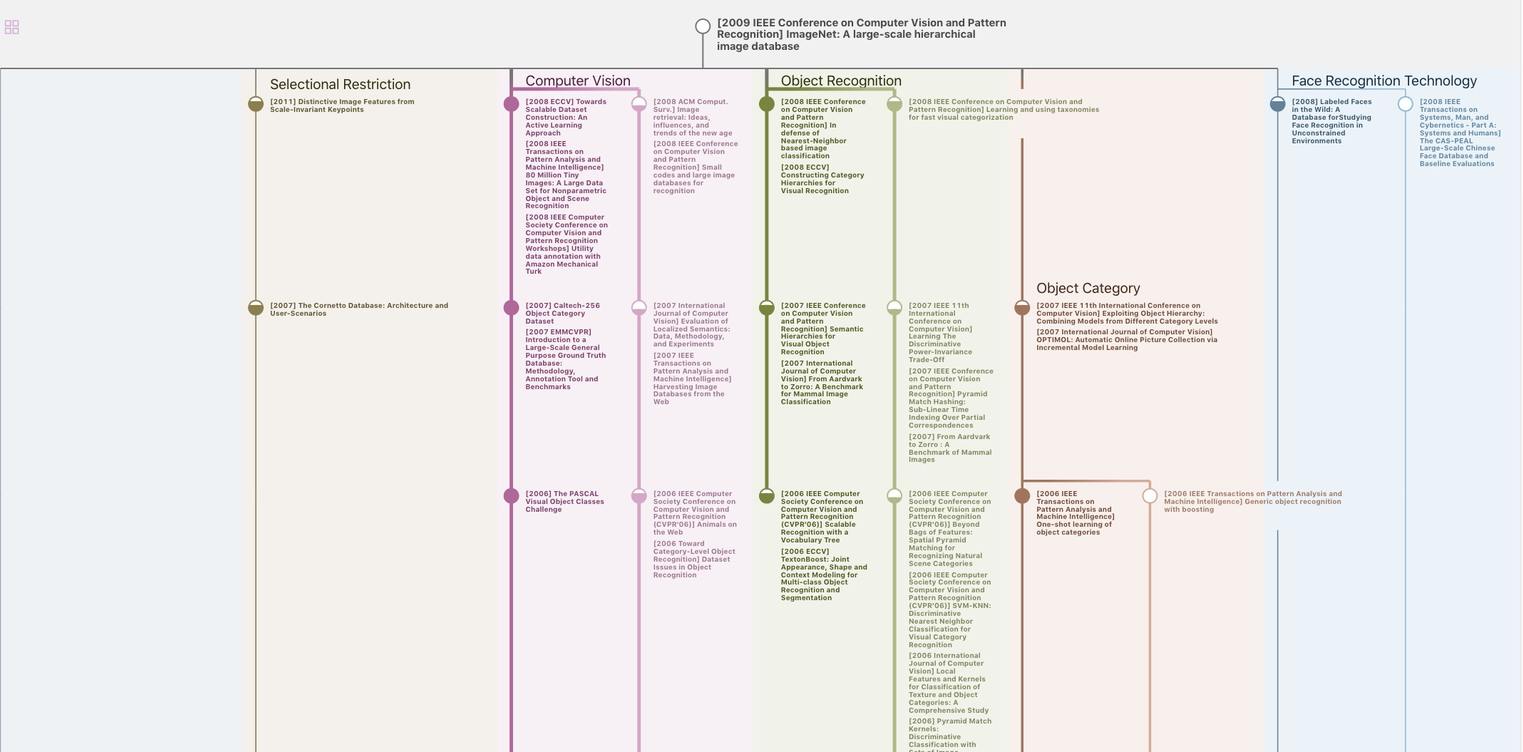
生成溯源树,研究论文发展脉络
Chat Paper
正在生成论文摘要