Improving Offline Value-Function Approximations For Pomdps By Reducing Discount Factors
2018 IEEE/RSJ INTERNATIONAL CONFERENCE ON INTELLIGENT ROBOTS AND SYSTEMS (IROS)(2018)
摘要
A common solution criterion for partially observable Markov decision processes (POMDPs) is to maximize the expected sum of exponentially discounted rewards, for which a variety of approximate methods have been proposed. Those that plan in the belief space typically provide tighter performance guarantees, but those that plan over the state space (e.g., QMDP and FIB) often require much less memory and computation. This paper presents an encouraging result that shows that reducing the discount factor while planning in the state space can actually improve performance significantly when evaluated on the original problem. This phenomenon is confirmed by both a theoretical analysis as well as a series of empirical studies on benchmark problems. As predicted by the theory and confirmed empirically, the phenomenon is most prominent when the observation model is noisy or rewards are sparse.
更多查看译文
关键词
exponentially discounted rewards,state space,observation model,offline value-function approximations,partially observable Markov decision processes,POMDP,discount factor reduction
AI 理解论文
溯源树
样例
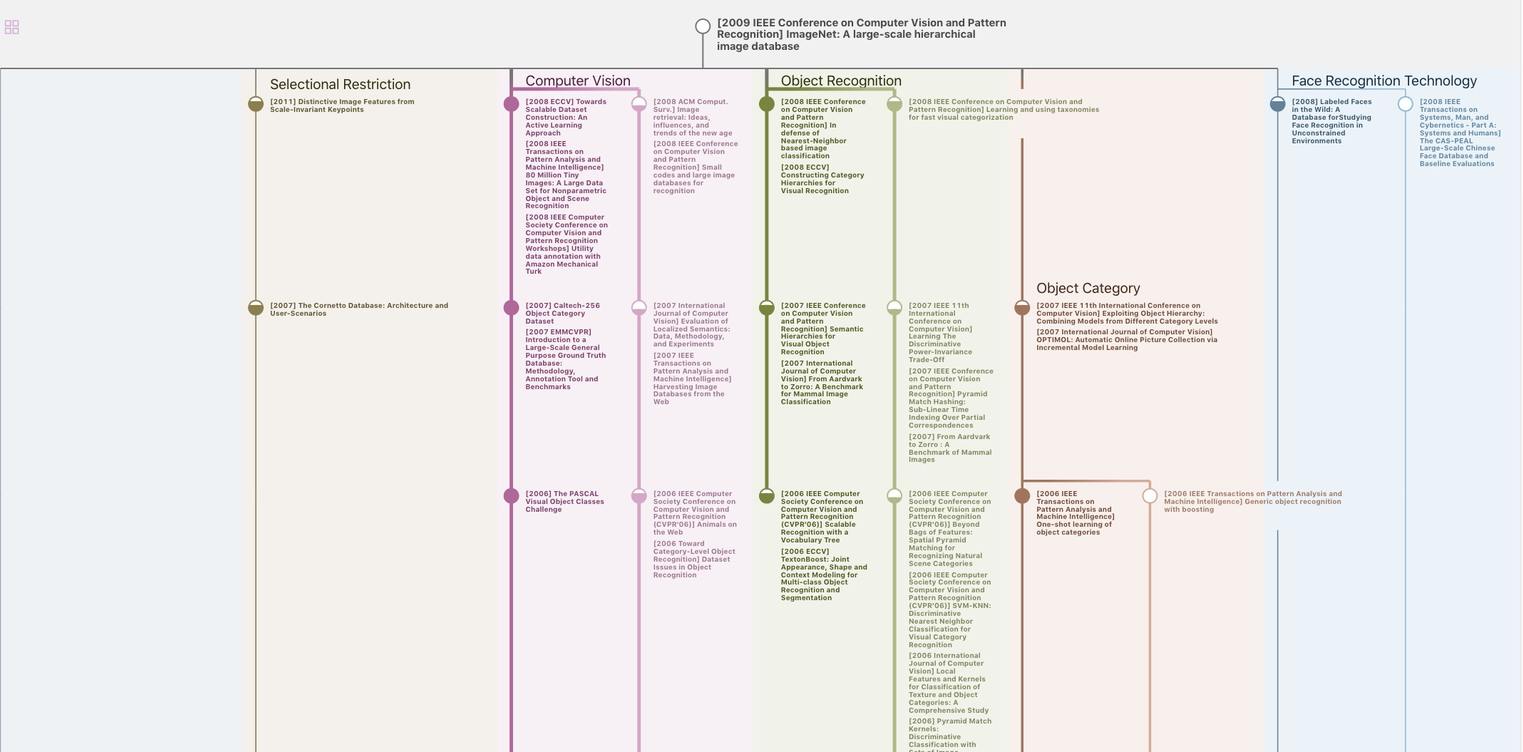
生成溯源树,研究论文发展脉络
Chat Paper
正在生成论文摘要