Deep Semantic Lane Segmentation For Mapless Driving
2018 IEEE/RSJ INTERNATIONAL CONFERENCE ON INTELLIGENT ROBOTS AND SYSTEMS (IROS)(2018)
摘要
In autonomous driving systems a strong relation to highly accurate maps is taken to be inevitable, although street scenes change frequently. However, a preferable system would be to equip the automated cars with a sensor system that is able to navigate urban scenarios without an accurate map. We present a novel pipeline using a deep neural network to detect lane semantics and topology given RGB images. On the basis of this classification, the information about the road scene can be extracted just from the sensor setup supporting mapless autonomous driving. In addition to superseding the huge effort of creating and maintaining highly accurate maps, our system reduces the need for precise localization.Using an extended Cityscapes dataset, we show accurate ego lane detection including lane semantics on challenging scenarios for autonomous driving.
更多查看译文
关键词
deep semantic lane segmentation,autonomous driving systems,automated cars,sensor system,urban scenarios,deep neural network,lane semantics,road scene,mapless autonomous driving,street scenes,RGB images,lane detection,cityscapes dataset
AI 理解论文
溯源树
样例
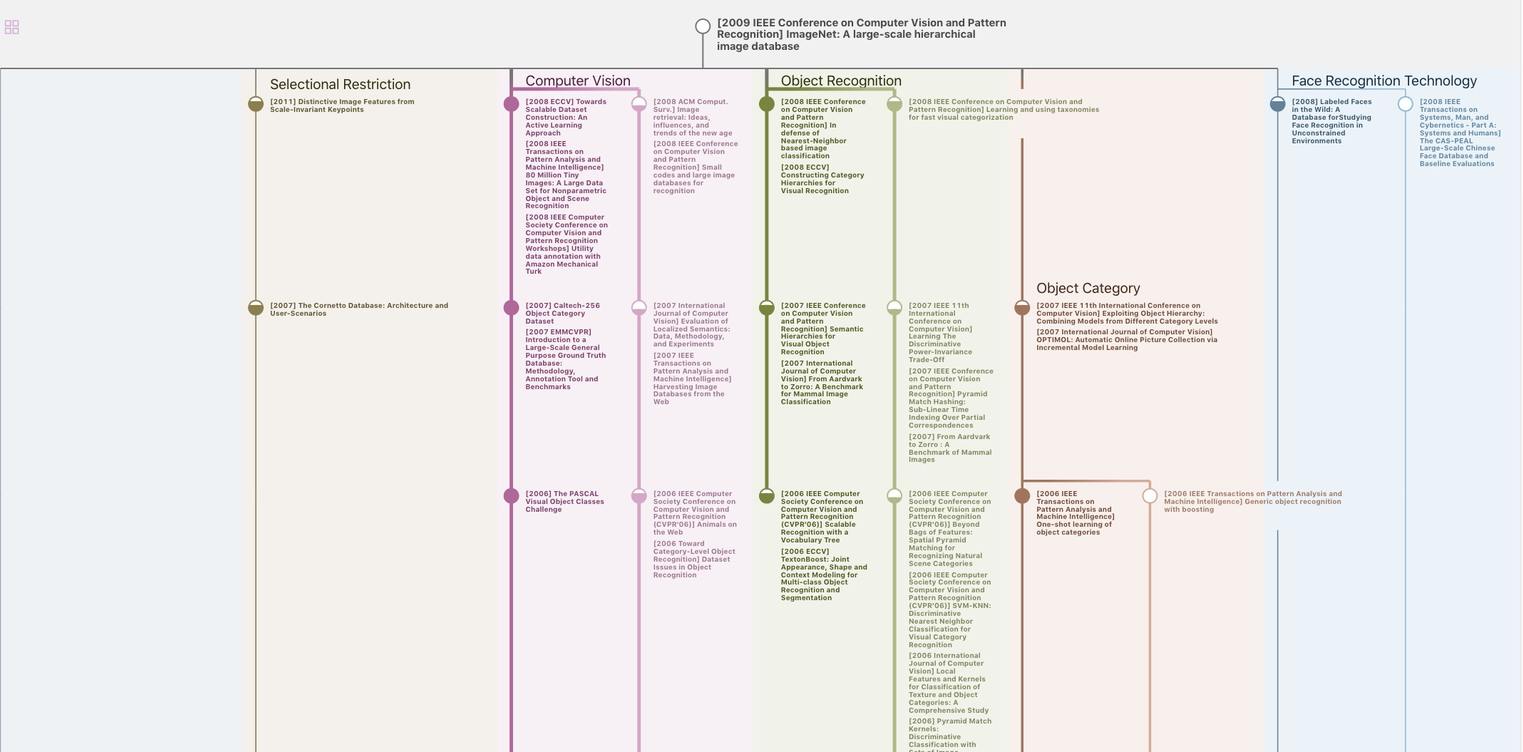
生成溯源树,研究论文发展脉络
Chat Paper
正在生成论文摘要