Online prediction of threading task failure using Convolutional Neural Networks.
IROS(2018)
摘要
Fasteners assembly automation in different industries require flexible systems capable of dealing with faulty situations. Fault detection and isolation (FDI) techniques are used to detect failure and deal with them, avoiding losses on parts, tools or robots. However, FDI usually deals with the faults after or at the moment they occur. Thus, we propose a method that predicts potential failures online, based on the forces and torques signatures captured during the task. We demonstrate the approach experimentally using an industrial robot, equipped with a force-torque sensor and a pneumatic gripper, used to align and thread nuts into bolts. All effort information is fed into a supervised machine learning algorithm, based on a Convolutional Neural Network (CNN) classifier. The network was able to predict and classify the threading task outcomes in 3 groups: mounted, not mounted or jammed. Our approach was able to reduce in 10.9% the threading task execution time when compared to a reference without FDI, but had problem to predict jammed cases. The same experiment was also performed with other two additional learning algorithms, and the results were systematically compared.
更多查看译文
关键词
online prediction,fasteners assembly automation,flexible systems,industrial robot,force-torque sensor,pneumatic gripper,supervised machine learning algorithm,threading task execution time,task failure,FDI techniques,convolutional neural network classifier,fault detection and isolation,CNN
AI 理解论文
溯源树
样例
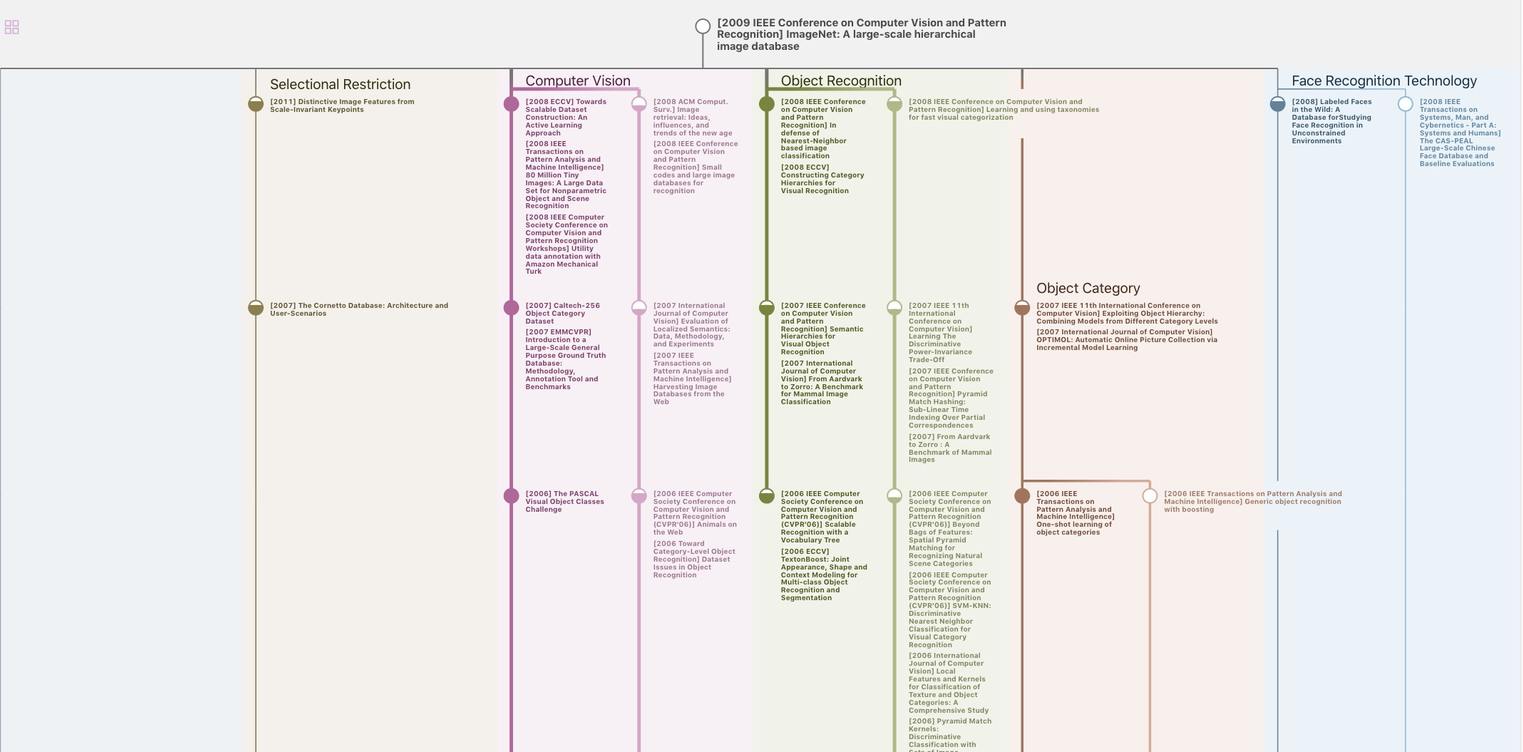
生成溯源树,研究论文发展脉络
Chat Paper
正在生成论文摘要