Learning Image-Conditioned Dynamics Models For Control Of Underactuated Legged Millirobots
2018 IEEE/RSJ INTERNATIONAL CONFERENCE ON INTELLIGENT ROBOTS AND SYSTEMS (IROS)(2018)
摘要
Millirobots are a promising robotic platform for many applications due to their small size and low manufacturing costs. Legged millirobots, in particular, can provide increased mobility in complex environments and improved scaling of obstacles. However, controlling these small, highly dynamic, and underactuated legged systems is difficult. Hand-engineered controllers can sometimes control these legged millirobots, but they have difficulties with dynamic maneuvers and complex terrains. We present an approach for controlling a real-world legged millirobot that is based on learned neural network models. Using less than 17 minutes of data, our method can learn a predictive model of the robot's dynamics that can enable effective gaits to be synthesized on the fly for following user-specified waypoints on a given terrain. Furthermore, by leveraging expressive, high-capacity neural network models, our approach allows for these predictions to be directly conditioned on camera images, endowing the robot with the ability to predict how different terrains might affect its dynamics. This enables sample-efficient and effective learning for locomotion of a dynamic legged millirobot on various terrains, including gravel, turf, carpet, and styrofoam. Videos and further details can be found at https://sites.google.com/view/imageconddyn
更多查看译文
关键词
underactuated legged systems,hand-engineered controllers,dynamic maneuvers,complex terrains,real-world legged millirobot,learned neural network models,predictive model,expressive capacity neural network models,high-capacity neural network models,effective learning,dynamic legged millirobot,image-conditioned dynamics models,underactuated legged millirobots,low manufacturing costs,complex environments,highly dynamic systems
AI 理解论文
溯源树
样例
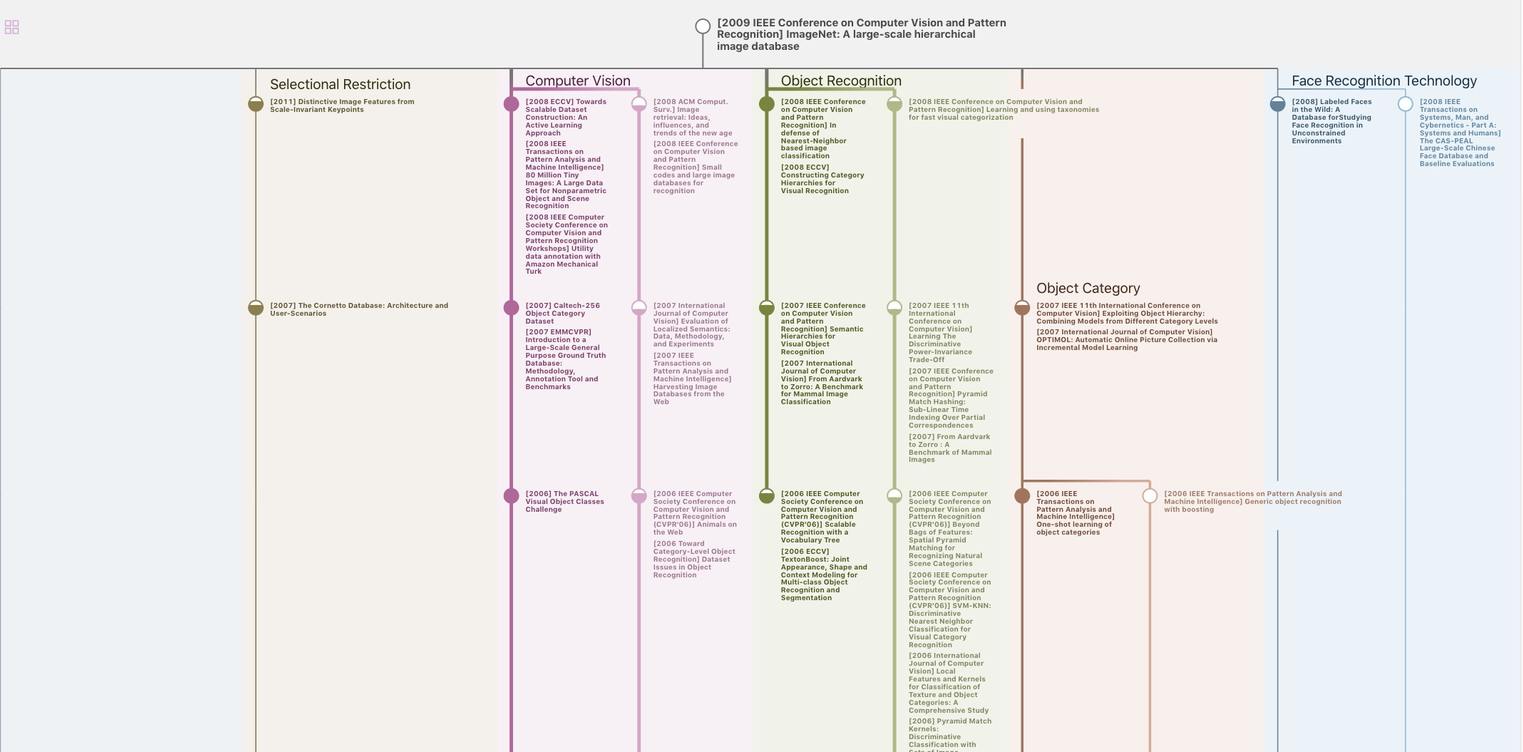
生成溯源树,研究论文发展脉络
Chat Paper
正在生成论文摘要