Lego-Loam: Lightweight And Ground-Optimized Lidar Odometry And Mapping On Variable Terrain
2018 IEEE/RSJ INTERNATIONAL CONFERENCE ON INTELLIGENT ROBOTS AND SYSTEMS (IROS)(2018)
摘要
We propose a lightweight and ground-optimized lidar odometry and mapping method, LeGO-LOAM, for real-time six degree-of-freedom pose estimation with ground vehicles. LeGO-LOAM is lightweight, as it can achieve real-time pose estimation on a low-power embedded system. LeGO-LOAM is ground-optimized, as it leverages the presence of a ground plane in its segmentation and optimization steps. We first apply point cloud segmentation to filter out noise, and feature extraction to obtain distinctive planar and edge features. A two-step Levenberg-Marquardt optimization method then uses the planar and edge features to solve different components of the six degree-of-freedom transformation across consecutive scans. We compare the performance of LeGO-LOAM with a state-of-the-art method, LOAM, using datasets gathered from variable-terrain environments with ground vehicles, and show that LeGO-LOAM achieves similar or better accuracy with reduced computational expense. We also integrate LeGO-LOAM into a SLAM framework to eliminate the pose estimation error caused by drift, which is tested using the KITTI dataset.
更多查看译文
关键词
SLAM framework,edge features,feature extraction,point cloud segmentation,lightweight and ground-optimized lidar odometry,real-time six degree-of-freedom pose estimation,low-power embedded system,ground plane,two-step Levenberg-Marquardt optimization method,optimization steps,ground vehicles,LeGO-LOAM
AI 理解论文
溯源树
样例
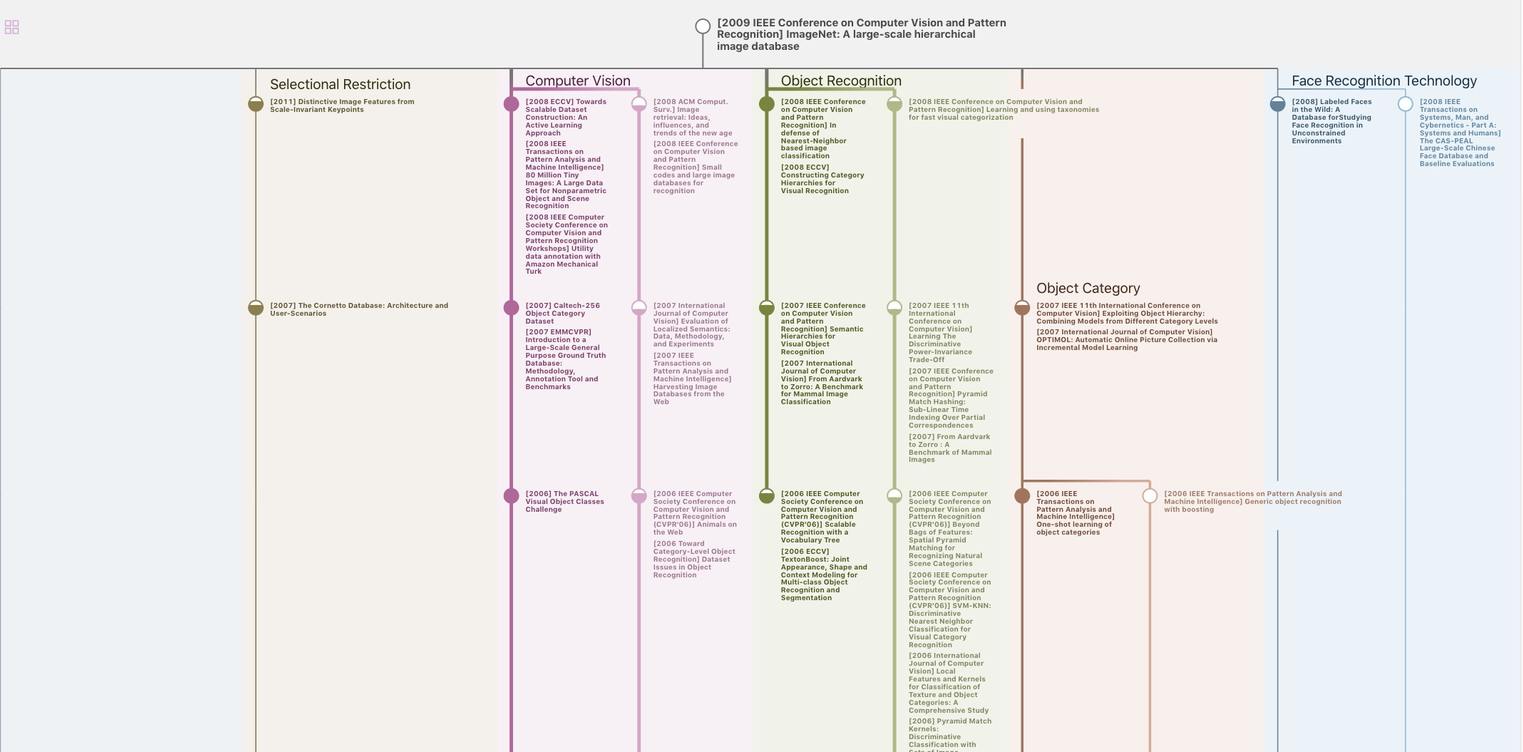
生成溯源树,研究论文发展脉络
Chat Paper
正在生成论文摘要