Work-in-Progress: Making Machine Learning Real-Time Predictable
2018 IEEE Real-Time Systems Symposium (RTSS)(2018)
摘要
Machine learning (ML) on edge computing devices is becoming popular in the industry as a means to make control systems more intelligent and autonomous. The new trend is to utilize embedded edge devices, as they boast higher computational power and larger memories than before, to perform ML tasks that had previously been limited to cloud-hosted deployments. In this work, we assess the real-time predictability and consider data privacy concerns by comparing traditional cloud services with edge-based ones for certain data analytics tasks. We identify the subset of ML problems appropriate for edge devices by investigating if they result in real-time predictable services for a set of widely used ML libraries. We specifically enhance the Caffe library to make it more suitable for real-time predictability. We then deploy ML models with high accuracy scores on an embedded system, exposing it to industry sensor data from the field, to demonstrates its efficacy and suitability for real-time processing.
更多查看译文
关键词
Edge Computing, Real-time Predictability, Keras, Caffe
AI 理解论文
溯源树
样例
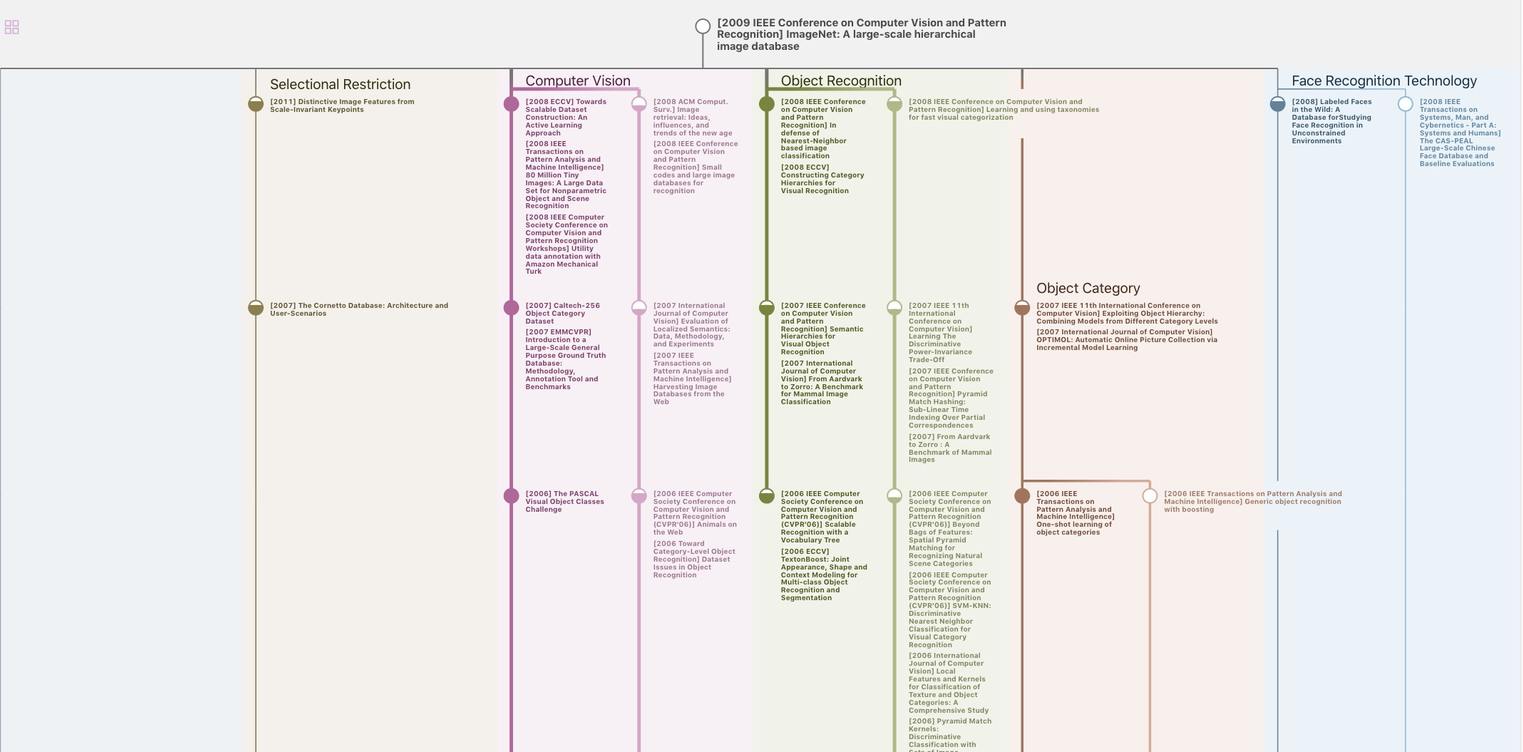
生成溯源树,研究论文发展脉络
Chat Paper
正在生成论文摘要