Dimension-independent Sparse Fourier Transform.
SODA '19: Symposium on Discrete Algorithms San Diego California January, 2019(2019)
摘要
The Discrete Fourier Transform (DFT) is a fundamental computational primitive, and the fastest known algorithm for computing the DFT is the FFT (Fast Fourier Transform) algorithm. One remarkable feature of FFT is the fact that its runtime depends only on the size N of the input vector, but not on the dimensionality of the input domain: FFT runs in time O(N log N) irrespective of whether the DFT in question is on ZN or Zdn for some d > 1, where N = nd.
The state of the art for Sparse FFT, i.e. the problem of computing the DFT of a signal that has at most k nonzeros in Fourier domain, is very different: all current techniques for sublinear time computation of Sparse FFT incur an exponential dependence on the dimension d in the runtime. In this paper we give the first algorithm that computes the DFT of a k-sparse signal in time poly(k, log N) in any dimension d, avoiding the curse of dimensionality inherent in all previously known techniques. Our main tool is a new class of filters that we refer to as adaptive aliasing filters: these filters allow isolating frequencies of a k-Fourier sparse signal using O(k) samples in time domain and O(k log N) runtime per frequency, in any dimension d.
We also investigate natural average case models of the input signal: worst case support in Fourier domain with randomized values and random locations in Fourier domain with worst case signal values. Our techniques lead to an Õ(k2) time algorithm for the former and an Õ(k) time algorithm for the latter.
更多查看译文
AI 理解论文
溯源树
样例
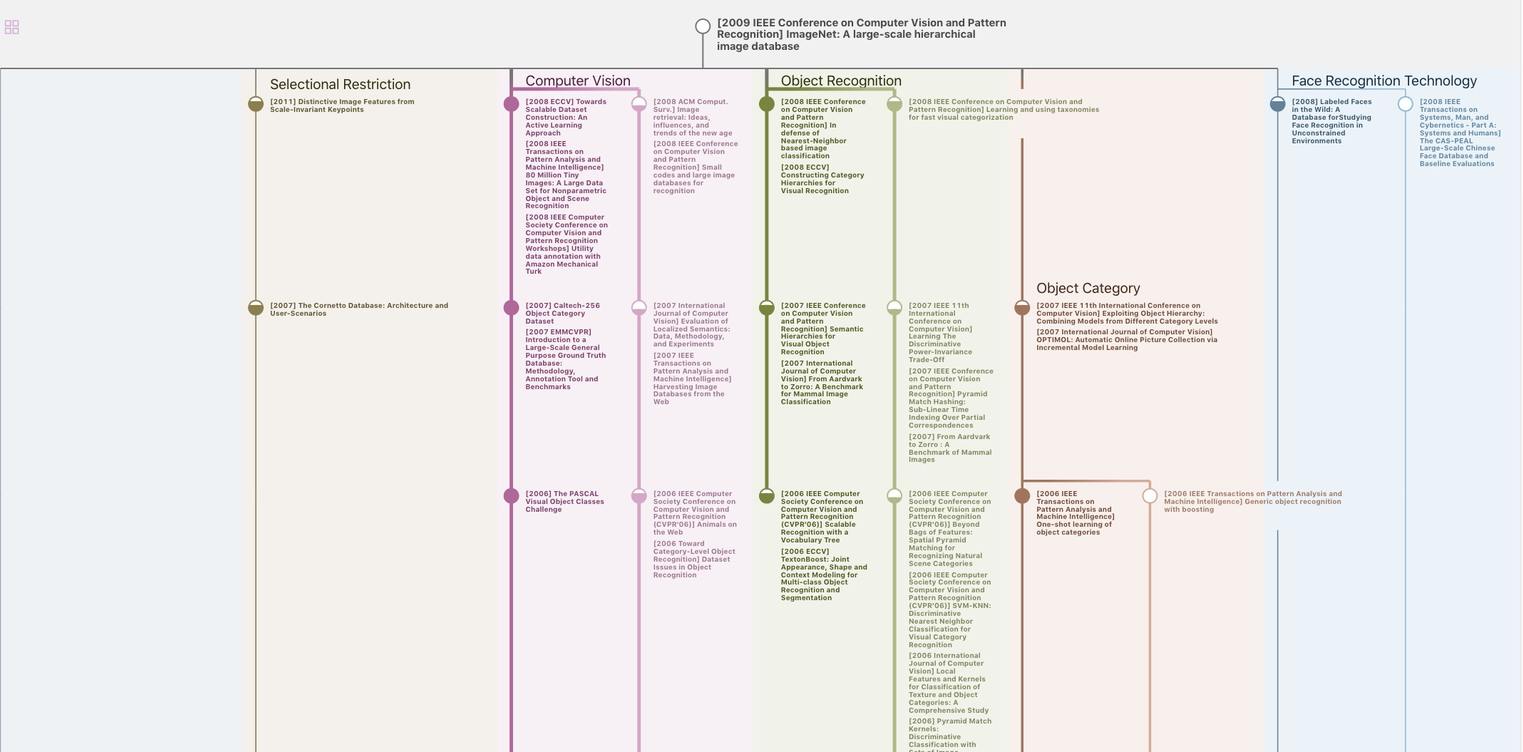
生成溯源树,研究论文发展脉络
Chat Paper
正在生成论文摘要