Forecasting Traffic Flow in Big Cities Using Modified Tucker Decomposition.
ADMA(2018)
摘要
An efficient traffic-network is an essential demand for any smart city. Usually, city traffic forms a huge network with millions of locations and trips. Traffic flow prediction using such large data is a classical problem in intelligent transportation system (ITS). Many existing models such as ARIMA, SVR, ANN etc, are deployed to retrieve important characteristics of traffic-network and for forecasting mobility. However, these methods suffer from the inability to handle higher data dimensionality. The tensor-based approach has recently gained success over the existing methods due to its ability to decompose high dimension data into factor components. We present a modified Tucker decomposition method which predicts traffic mobility by approximating very large networks so as to handle the dimensionality problem. Our experiments on two big-city traffic-networks show that our method reduces the forecasting error, for up to 7 days, by around 80% as compared to the existing state of the art methods. Further, our method also efficiently handles the data dimensionality problem as compared to the existing methods.
更多查看译文
关键词
ODM, CP decomposition, Tucker, Time-series, CUR, Traffic flow
AI 理解论文
溯源树
样例
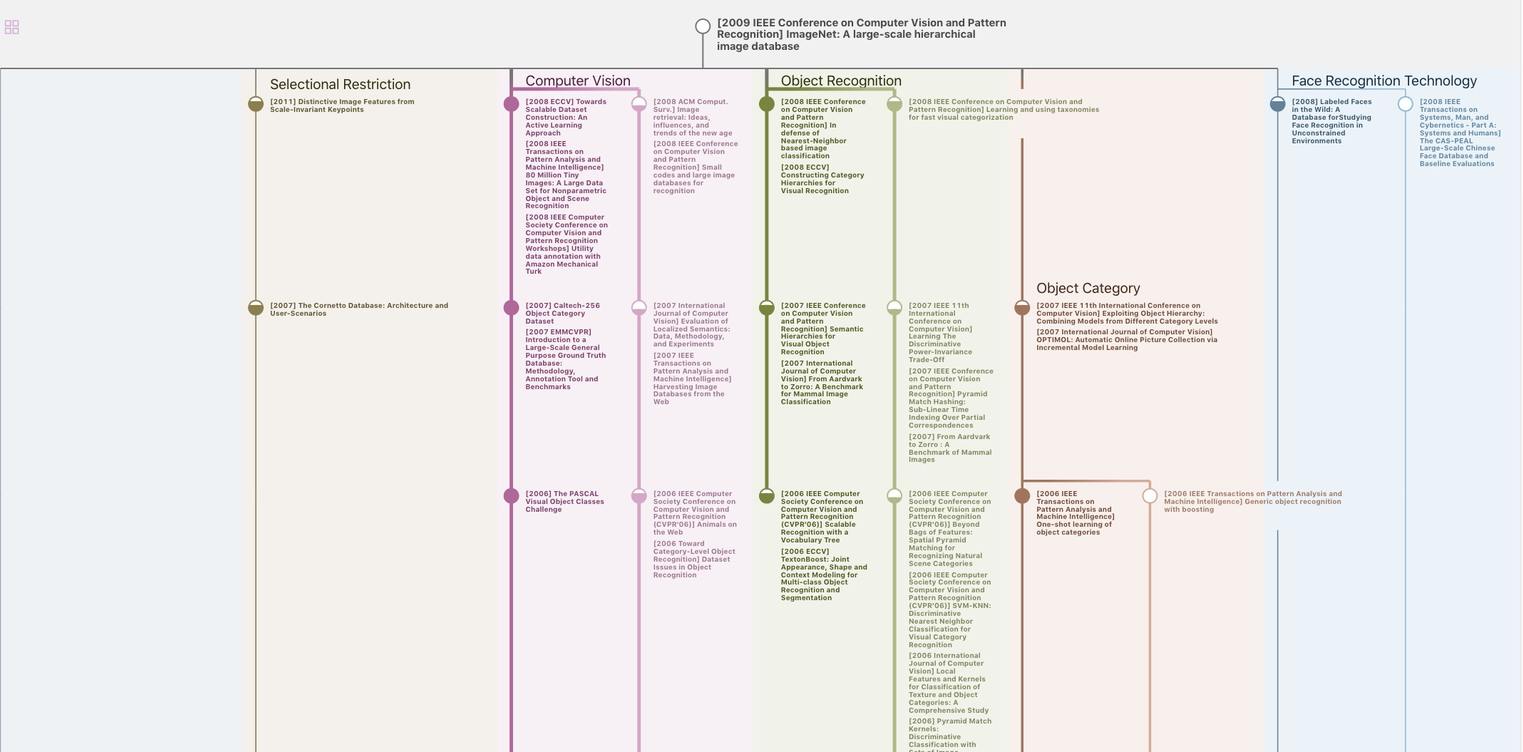
生成溯源树,研究论文发展脉络
Chat Paper
正在生成论文摘要