Application of audible sound signals for tool wear monitoring using machine learning techniques in end milling
The International Journal of Advanced Manufacturing Technology(2017)
摘要
Due to the demands of Computer-Integrated Manufacturing (CIM), the Tool Condition Monitoring (TCM) system, as a major component of CIM, is essential to improve the production quality, optimize the labor and maintenance costs, and minimize the manufacturing loses with the increase in productivity. To look for a reliable, efficient, and cost-effective solution, various monitoring systems employing different types of sensing techniques have been developed to detect the tool conditions as well as to monitor the abnormal cutting states. This paper explores the use of audible sound signals as sensing approach to detect the cutting tool wear and failure during end milling operation by using the Support Vector Machine (SVM) learning model as a decision-making algorithm. In this study, sound signals collected during the machining process are analyzed through frequency domain to extract signal features that correlate actual cutting phenomenon. The SVM method seeks to provide a linguistic model for tool wear estimation from the knowledge embedded in this machine learning approach. The performance evaluation results of the proposed algorithm have shown accurate predictions in detecting tool wear under various cutting conditions with rapid response rate, which provides the good solution for in-process TCM. In addition, the proposed monitoring system trained with sufficient signals collected from different positions has been proved to be position independent to monitor the tool wear conditions.
更多查看译文
关键词
Tool condition monitoring,Tool wear,Audible sound,Machine learning,Support vector machine
AI 理解论文
溯源树
样例
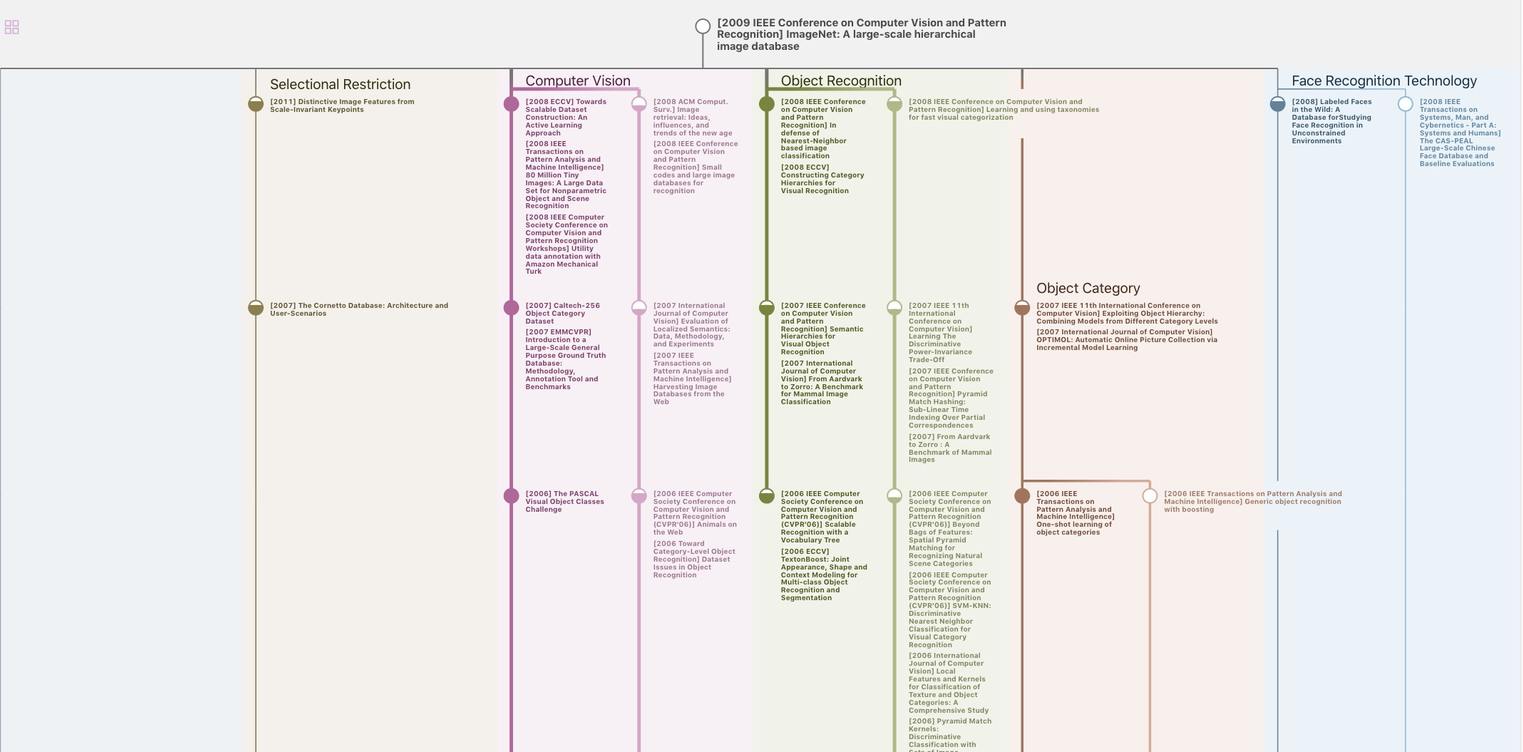
生成溯源树,研究论文发展脉络
Chat Paper
正在生成论文摘要