An Adaptive Computation Framework of Distributed Deep Learning Models for Internet-of-Things Applications
2018 IEEE 24th International Conference on Embedded and Real-Time Computing Systems and Applications (RTCSA)(2018)
摘要
We propose the computation framework that facilitates the inference of the distributed deep learning model to be performed collaboratively by the devices in a distributed computing hierarchy. For example, in Internet-of-Things (IoT) applications, the three-tier computing hierarchy consists of end devices, gateways, and server(s), and the model inference could be done adaptively by one or more computing tiers from the bottom to the top of the hierarchy. By allowing the trained models to run on the actually distributed systems, which has not done by the previous work, the proposed framework enables the co-design of the distributed deep learning models and systems. In particular, in addition to the model accuracy, which is the major concern for the model designers, we found that as various types of computing platforms are present in IoT applications fields, measuring the delivered performance of the developed models on the actual systems is also critical to making sure that the model inference does not cost too much time on the end devices. Furthermore, the measured performance of the model (and the system) would be a good input to the model/system design in the next design cycle, e.g., to determine a better mapping of the network layers onto the hierarchy tiers. On top of the framework, we have built the surveillance system for detecting objects as a case study. In our experiments, we evaluate the delivered performance of model designs on the two-tier computing hierarchy, show the advantages of the adaptive inference computation, analyze the system capacity under the given workloads, and discuss the impact of the model parameter setting on the system capacity. We believe that the enablement of the performance evaluation expedites the design process of the distributed deep learning models/systems.
更多查看译文
关键词
Distributed systems design,distributed deep learning models design,parallel computing,Internet-of-Things applications,embedded devices,OpenCL accelerations,MQTT
AI 理解论文
溯源树
样例
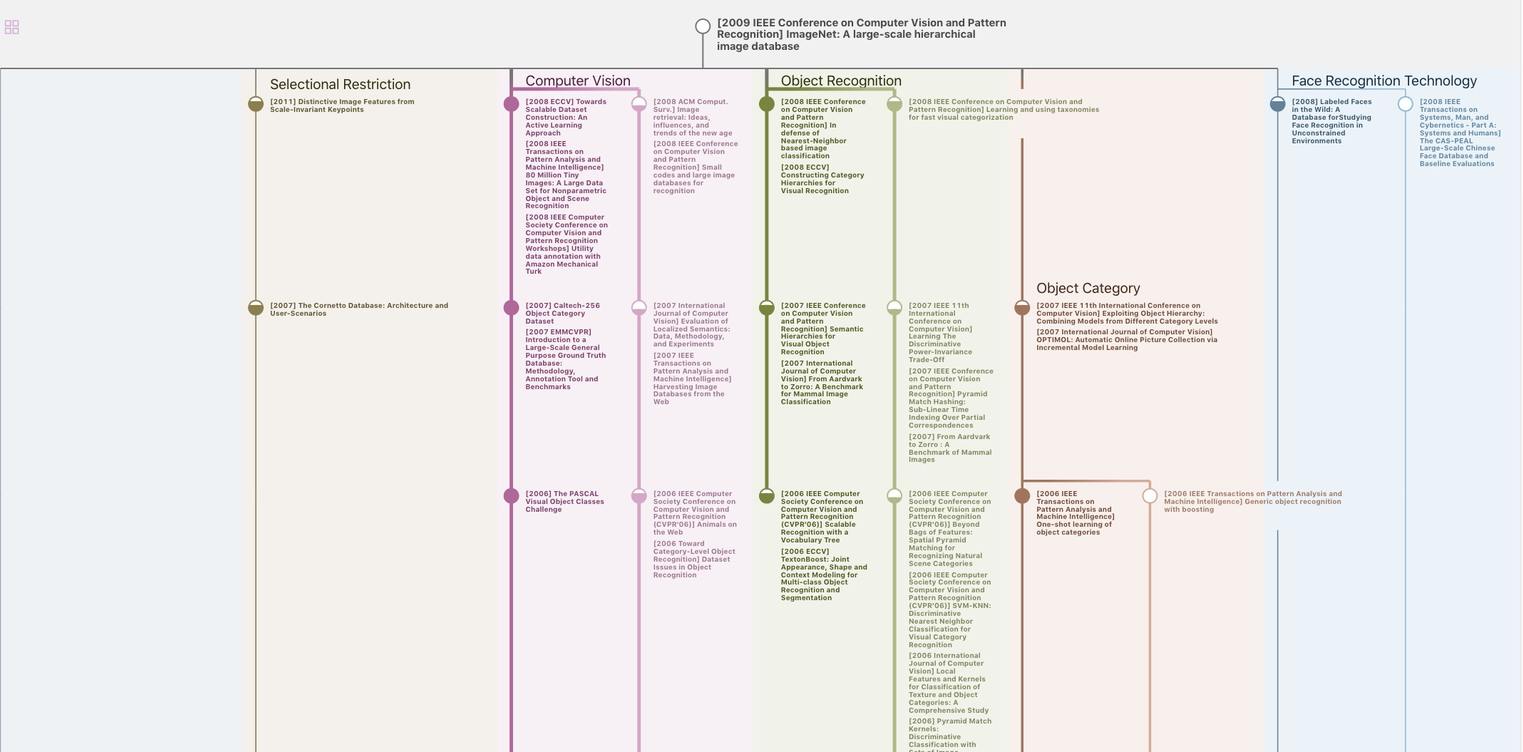
生成溯源树,研究论文发展脉络
Chat Paper
正在生成论文摘要