Meritocratic Fairness For Infinite And Contextual Bandits
PROCEEDINGS OF THE 2018 AAAI/ACM CONFERENCE ON AI, ETHICS, AND SOCIETY (AIES'18)(2018)
摘要
We study fairness in linear bandit problems. Starting from the notion of meritocratic fairness introduced in [13], we carry out a more refined analysis of a more general problem, achieving better performance guarantees with fewer modelling assumptions on the number and structure of available choices as well as the number selected. We also analyze the previously-unstudied question of fairness in infinite linear bandit problems, obtaining instance-dependent regret upper bounds as well as lower bounds demonstrating that this instance-dependence is necessary. The result is a framework for meritocratic fairness in an online linear setting that is substantially more powerful, general, and realistic than the current state of the art.
更多查看译文
AI 理解论文
溯源树
样例
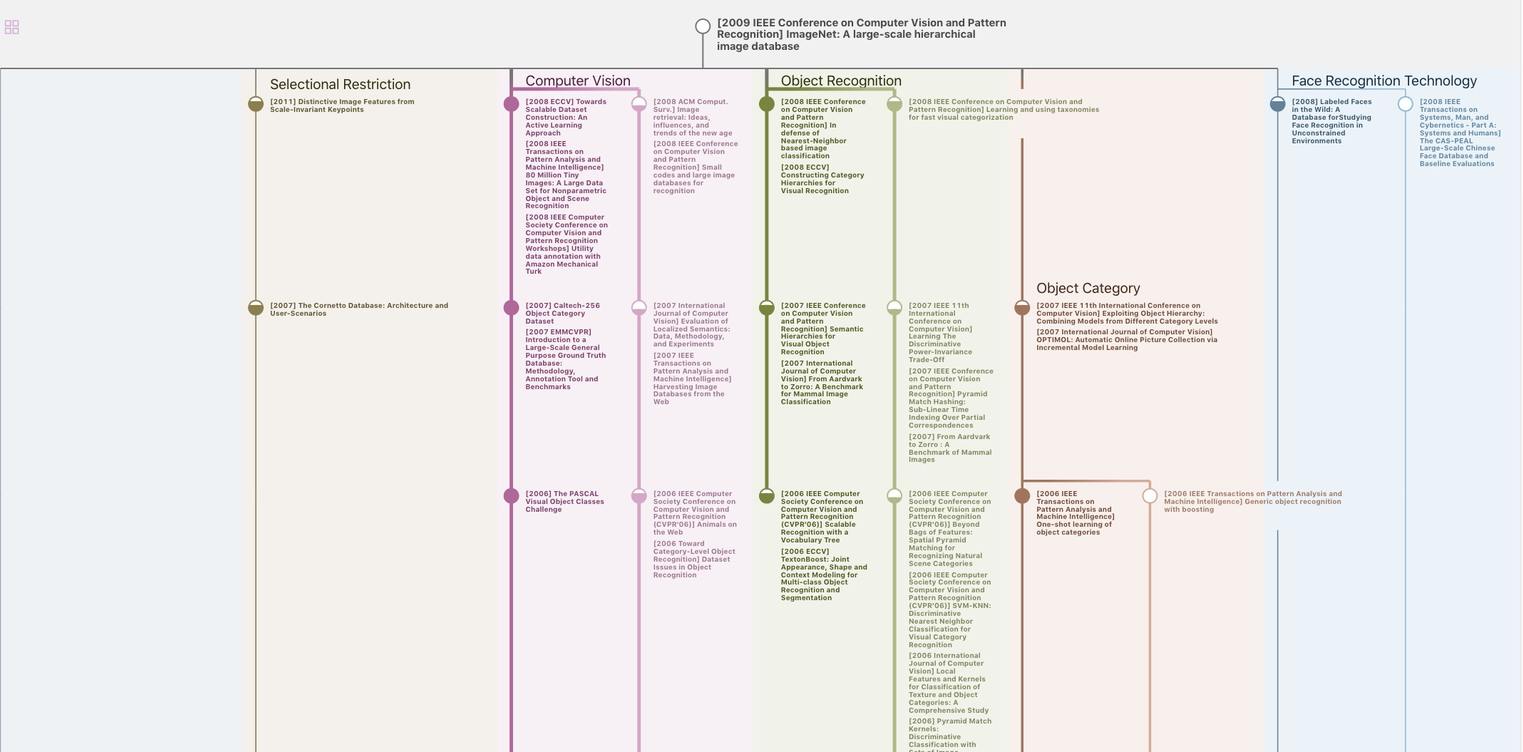
生成溯源树,研究论文发展脉络
Chat Paper
正在生成论文摘要