Multi-scale pulmonary nodule classification with deep feature fusion via residual network
Journal of Ambient Intelligence and Humanized Computing(2018)
摘要
The early stage detection of benign and malignant pulmonary nodules plays an important role in clinical diagnosis. The malignancy risk assessment is usually used to guide the doctor in identifying the cancer stage and making follow-up prognosis plan. However, due to the variance of nodules on size, shape, and location, it has been a big challenge to classify the nodules in computer aided diagnosis system. In this paper, we design a novel model based on convolution neural network to achieve automatic pulmonary nodule malignancy classification. By using our model, the multi-scale features are extracted through the multi-convolution process, and the structure of residual blocks allows the network to capture more high-level and semantic information. Moreover, a strategy is proposed to fuse the features from the last avg-pooling layer and the ones from the last residual block to further enhance the performance of our model. Experimental results on the public Lung Image Database Consortium dataset demonstrate that our model can achieve a lung nodule classification accuracy of 87.5% which outperforms state-of-the-art methods.
更多查看译文
关键词
Pulmonary nodule classification,Multi-scale,Residual block,Feature fusion
AI 理解论文
溯源树
样例
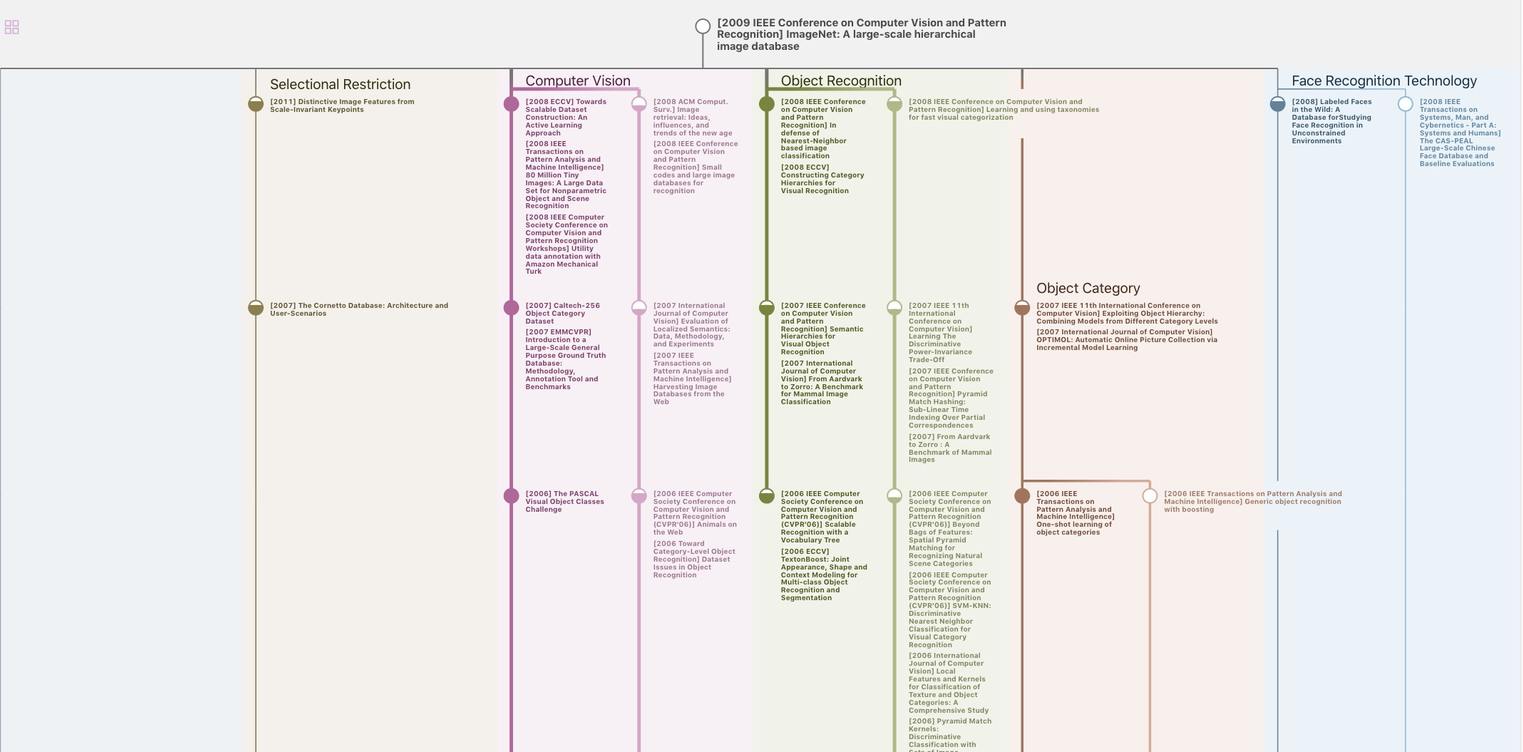
生成溯源树,研究论文发展脉络
Chat Paper
正在生成论文摘要