On the effect of model mismatch for sequential Info-Greedy Sensing
EURASIP Journal on Advances in Signal Processing(2018)
摘要
We characterize the performance of sequential information-guided sensing (Info-Greedy Sensing) when the model parameters (means and covariance matrices) are estimated and inaccurate. Our theoretical results focus on Gaussian signals and establish performance bounds for signal estimators obtained by Info-Greedy Sensing, in terms of conditional entropy (related to the estimation error) and additional power required due to inaccurate models. We also show covariance sketching can be used as an efficient initialization for Info-Greedy Sensing. Numerical examples demonstrate the good performance of Info-Greedy Sensing algorithms compared with random measurement schemes in the presence of model mismatch.
更多查看译文
关键词
Sequential compressed sensing,Adaptive sensing,Mutual information,Model mismatch
AI 理解论文
溯源树
样例
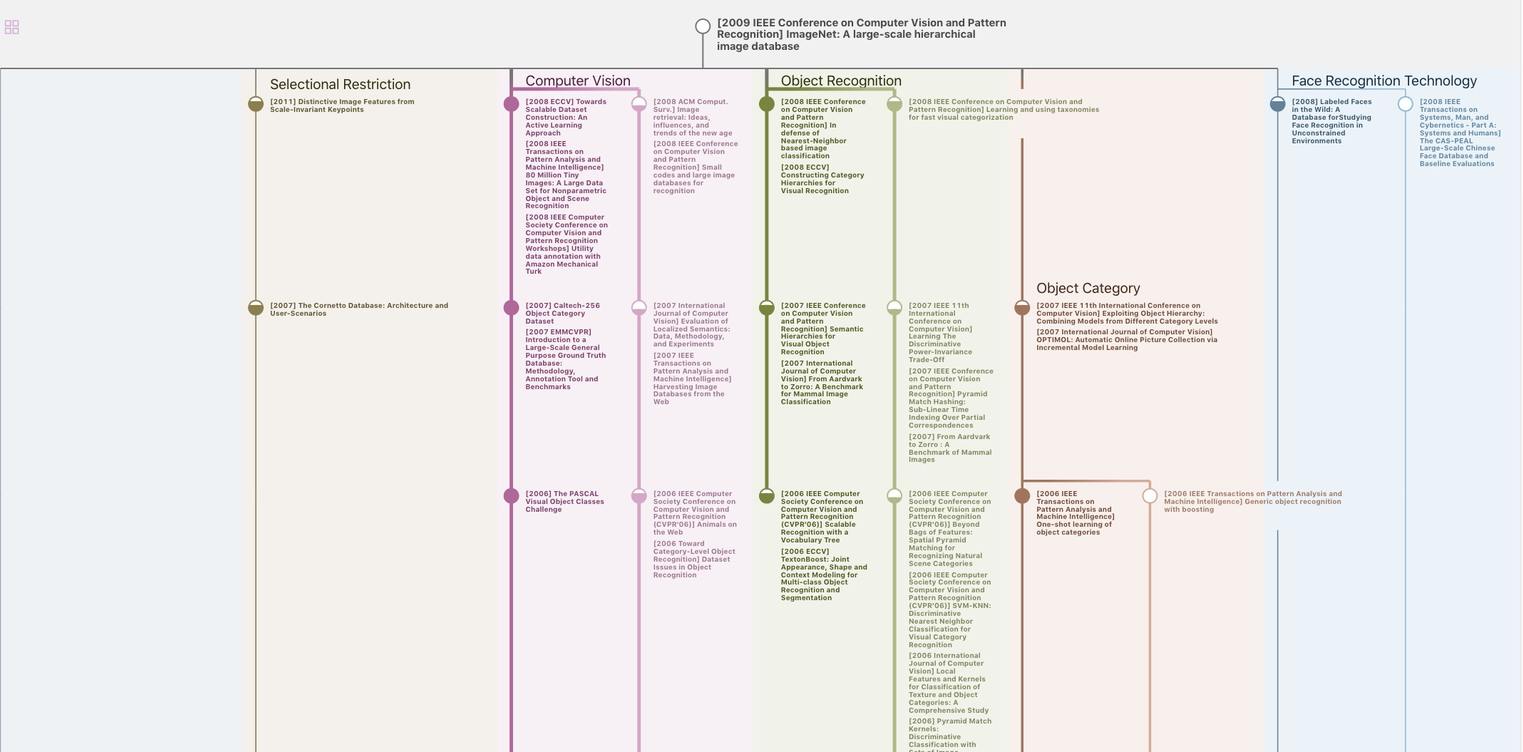
生成溯源树,研究论文发展脉络
Chat Paper
正在生成论文摘要