e ToxPred: a machine learning-based approach to estimate the toxicity of drug candidates
BMC PHARMACOLOGY & TOXICOLOGY(2019)
摘要
Background The efficiency of drug development defined as a number of successfully launched new pharmaceuticals normalized by financial investments has significantly declined. Nonetheless, recent advances in high-throughput experimental techniques and computational modeling promise reductions in the costs and development times required to bring new drugs to market. The prediction of toxicity of drug candidates is one of the important components of modern drug discovery. Results In this work, we describe e ToxPred, a new approach to reliably estimate the toxicity and synthetic accessibility of small organic compounds. e ToxPred employs machine learning algorithms trained on molecular fingerprints to evaluate drug candidates. The performance is assessed against multiple datasets containing known drugs, potentially hazardous chemicals, natural products, and synthetic bioactive compounds. Encouragingly, e ToxPred predicts the synthetic accessibility with the mean square error of only 4% and the toxicity with the accuracy of as high as 72%. Conclusions e ToxPred can be incorporated into protocols to construct custom libraries for virtual screening in order to filter out those drug candidates that are potentially toxic or would be difficult to synthesize. It is freely available as a stand-alone software at https://github.com/pulimeng/etoxpred .
更多查看译文
关键词
Virtual screening, Synthetic accessibility, Toxicity, Machine learning, Deep belief network, Extremely randomized trees
AI 理解论文
溯源树
样例
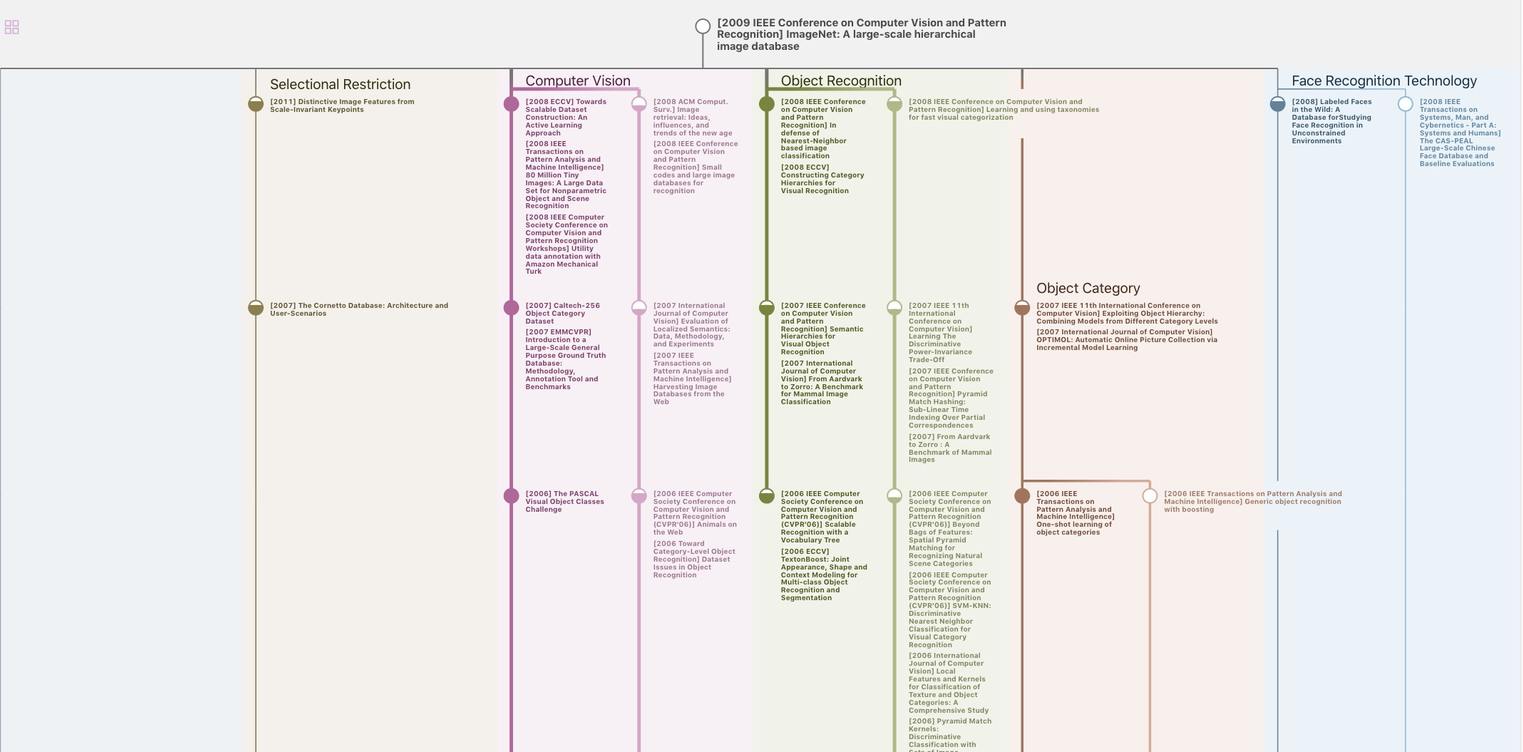
生成溯源树,研究论文发展脉络
Chat Paper
正在生成论文摘要