DeepMoCap: Deep Optical Motion Capture Using Multiple Depth Sensors and Retro-Reflectors.
SENSORS(2019)
摘要
In this paper, a marker-based, single-person optical motion capture method (DeepMoCap) is proposed using multiple spatio-temporally aligned infrared-depth sensors and retro-reflective straps and patches (reflectors). DeepMoCap explores motion capture by automatically localizing and labeling reflectors on depth images and, subsequently, on 3D space. Introducing a non-parametric representation to encode the temporal correlation among pairs of colorized depthmaps and 3D optical flow frames, a multi-stage Fully Convolutional Network (FCN) architecture is proposed to jointly learn reflector locations and their temporal dependency among sequential frames. The extracted reflector 2D locations are spatially mapped in 3D space, resulting in robust 3D optical data extraction. The subject's motion is efficiently captured by applying a template-based fitting technique on the extracted optical data. Two datasets have been created and made publicly available for evaluation purposes; one comprising multi-view depth and 3D optical flow annotated images (DMC2.5D), and a second, consisting of spatio-temporally aligned multi-view depth images along with skeleton, inertial and ground truth MoCap data (DMC3D). The FCN model outperforms its competitors on the DMC2.5D dataset using 2D Percentage of Correct Keypoints (PCK) metric, while the motion capture outcome is evaluated against RGB-D and inertial data fusion approaches on DMC3D, outperforming the next best method by 4.5% in total 3D PCK accuracy.
更多查看译文
关键词
motion capture,deep learning,retro-reflectors,retro-reflective markers,multiple depth sensors,low-cost,deep mocap,depth data,3D data,3D vision,optical mocap,marker-based mocap
AI 理解论文
溯源树
样例
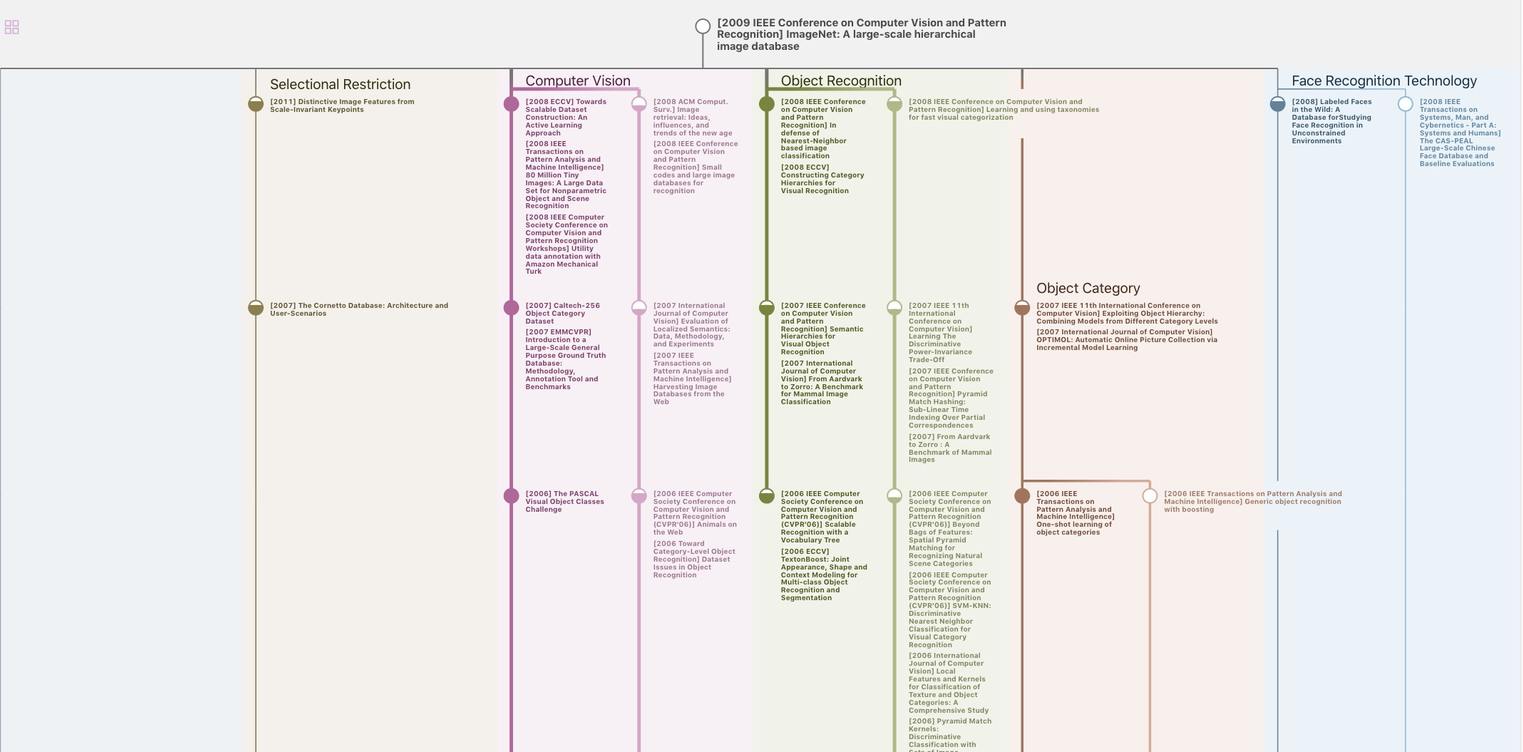
生成溯源树,研究论文发展脉络
Chat Paper
正在生成论文摘要