An Always-On 3.8 $\mu$ J/86% CIFAR-10 Mixed-Signal Binary CNN Processor With All Memory on Chip in 28-nm CMOS
IEEE Journal of Solid-State Circuits(2019)
摘要
The trend of pushing inference from cloud to edge due to concerns of latency, bandwidth, and privacy has created demand for energy-efficient neural network hardware. This paper presents a mixed-signal binary convolutional neural network (CNN) processor for always-on inference applications that achieves 3.8
$\mu \text{J}$
/classification at 86% accuracy on the CIFAR-10 image classification data set. The goal of this paper is to establish the minimum-energy point for the representative CIFAR-10 inference task, using the available design tradeoffs. The BinaryNet algorithm for training neural networks with weights and activations constrained to +1 and −1 drastically simplifies multiplications to XNOR and allows integrating all memory on-chip. A weight-stationary, data-parallel architecture with input reuse amortizes memory access across many computations, leaving wide vector summation as the remaining energy bottleneck. This design features an energy-efficient switched-capacitor (SC) neuron that addresses this challenge, employing a 1024-bit thermometer-coded capacitive digital-to-analog converter (CDAC) section for summing pointwise products of CNN filter weights and activations and a 9-bit binary-weighted section for adding the filter bias. The design occupies 6 mm
2
in 28-nm CMOS, contains 328 kB of on-chip SRAM, operates at 237 frames/s (FPS), and consumes 0.9 mW from 0.6 V/0.8 V supplies. The corresponding energy per classification (3.8
$\mu \text{J}$
) amounts to a 40
$\times $
improvement over the previous low-energy benchmark on CIFAR-10, achieved in part by sacrificing some programmability. The SC neuron array is 12.9
$\times $
more energy efficient than a synthesized digital implementation, which amounts to a 4
$\times $
advantage in system-level energy per classification.
更多查看译文
关键词
Topology,Neurons,Memory management,Hardware,Arrays,Parallel processing,Task analysis
AI 理解论文
溯源树
样例
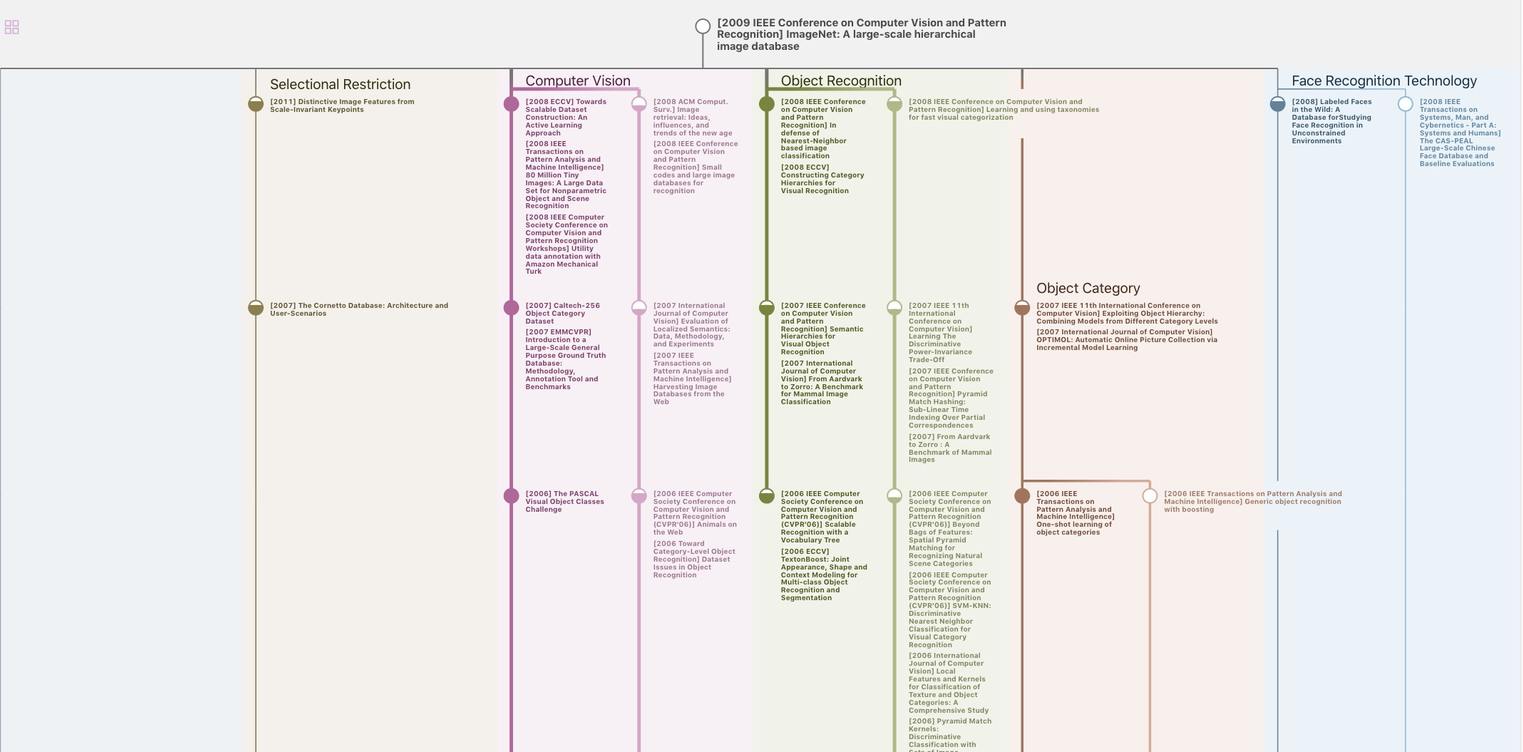
生成溯源树,研究论文发展脉络
Chat Paper
正在生成论文摘要