Enablers Of Adversarial Attacks In Machine Learning
2018 IEEE MILITARY COMMUNICATIONS CONFERENCE (MILCOM 2018)(2018)
摘要
The proliferation of machine learning (ML) and artificial intelligence (AI) systems for military and security applications creates substantial challenges for designing and deploying such mechanisms that would learn, adapt, reason and act with Dinky, Dirty, Dynamic, Deceptive, Distributed (D5) data. While Dinky and Dirty challenges have been extensively explored in ML theory, the Dynamic challenge has been a persistent problem in ML applications (when the statistical distribution of training data differs from that of test data). The most recent Deceptive challenge is a malicious distribution shift between training and test data that amplifies the effects of the Dynamic challenge to the complete breakdown of the ML algorithms. Using the MNIST dataset as a simple calibration example, we explore the following two questions: (1) What geometric and statistical characteristics of data distribution can be exploited by an adversary with a given magnitude of the attack? (2) What counter-measures can be used to protect the constructed decision rule (at the cost of somewhat decreased performance) against malicious distribution shift within a given magnitude of the attack? While not offering a complete solution to the problem, we collect and interpret obtained observations in a way that provides practical guidance for making more adversary-resistant choices in the design of ML algorithms.
更多查看译文
关键词
Supervised learning, support vector machines, kernel, data models, feature extraction, training, training data, classification algorithms, machine learning algorithms, learning systems, distribution functions, distortion, adversarial examples, neural networks
AI 理解论文
溯源树
样例
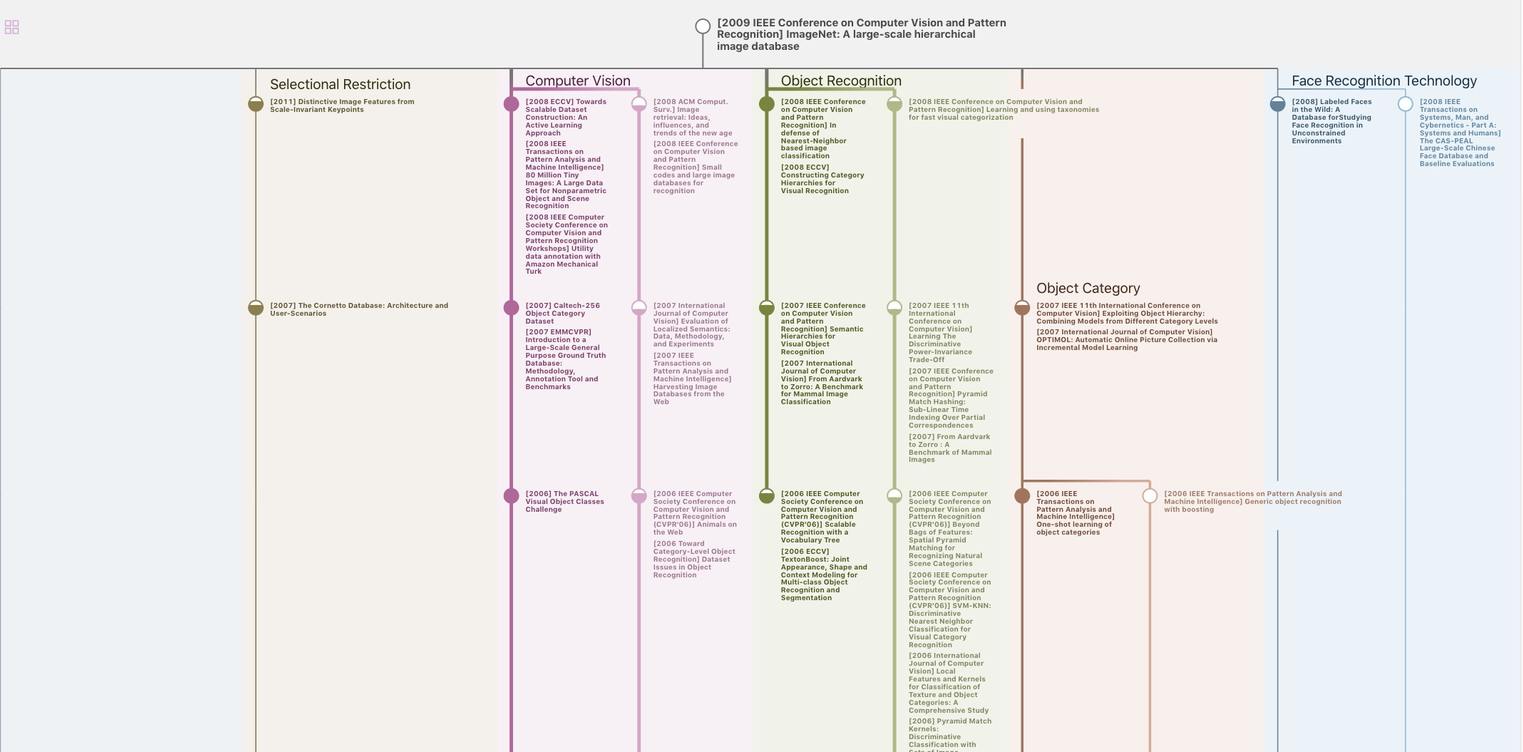
生成溯源树,研究论文发展脉络
Chat Paper
正在生成论文摘要