A Reinforcement Learning Approach To Adaptive Redundancy For Routing In Tactical Networks
2018 IEEE MILITARY COMMUNICATIONS CONFERENCE (MILCOM 2018)(2018)
摘要
Providing deterministic communication guarantees in dynamic and unreliable tactical networks is an ongoing challenge. Traditional routing protocols cannot adapt to the frequent topology changes inherent in battlefield scenarios, and robust flooding approaches are prohibitively expensive in terms of overhead. This paper presents a novel adaptive routing algorithm based on techniques from reinforcement learning. An online, collaborative learning algorithm gathers information about the path quality and availability to improve the packet forwarding process. Redundant routing is used when the network is highly dynamic or unknown which simultaneously increases reliability and provides ample opportunities to quickly learn the network state. This unique approach provides the benefits of learning without the drawback of a lengthy training period. The algorithm has been implemented in a Linux environment and evaluated using the CORE network emulator.
更多查看译文
关键词
reinforcement learning approach,adaptive redundancy,unreliable tactical networks,traditional routing protocols,battlefield scenarios,robust flooding approaches,collaborative learning algorithm,path quality,packet forwarding process,redundant routing,network state,CORE network emulator,deterministic communication,frequent topology,Linux environment,reinforcement learning
AI 理解论文
溯源树
样例
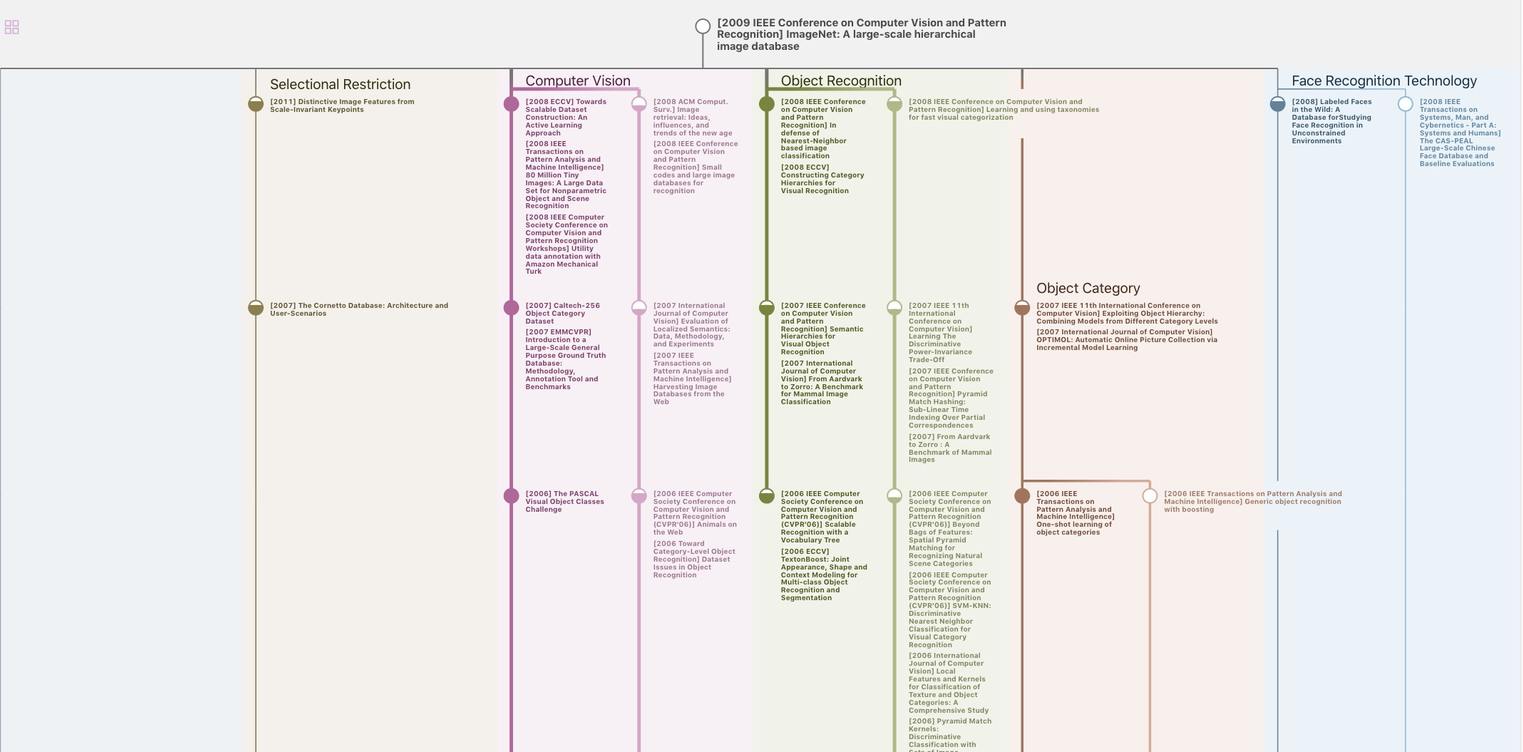
生成溯源树,研究论文发展脉络
Chat Paper
正在生成论文摘要