Network Traffic Obfuscation: An Adversarial Machine Learning Approach
2018 IEEE MILITARY COMMUNICATIONS CONFERENCE (MILCOM 2018)(2018)
摘要
An agent (D) aims to defend a network's traffic (T) from inference (classification) of applications or protocols (P) traversing that nework by an attacker (A). D aims to confuse A as to the nature of T by altering T to T' so that A cannot easily ascertain the class of T'. If D is successful, A concludes that T' belongs to class Q different from the true class P. A variety of approaches have been advanced to this general problem in the primary literature; however, research shows that even if the data contents of T are altered (e.g., through encryption), the meta-data aspects of T and T' are similar (e.g., similar packet statistics like size and inter-arrival time). Thus, inference of P is still possible from observing the statistical properties of T'; D must thus further obfuscate these features as well. However, heavy-handed obfuscation could break the protocol or incur substantial overhead; hence minimal perturbations are desired. In this paper, we assume that A is able to observe statistical properties of T. We study the question: how can D optimally create T ' so that A infers T ' belongs to a class other than the true class P, with the additional constraint that T ' is close to T? Insights from the emerging area of adversarial machine learning (AML) provide unique perspectives in answering this question.
更多查看译文
关键词
network traffic analysis, traffic obfuscation, adversarial machine learning
AI 理解论文
溯源树
样例
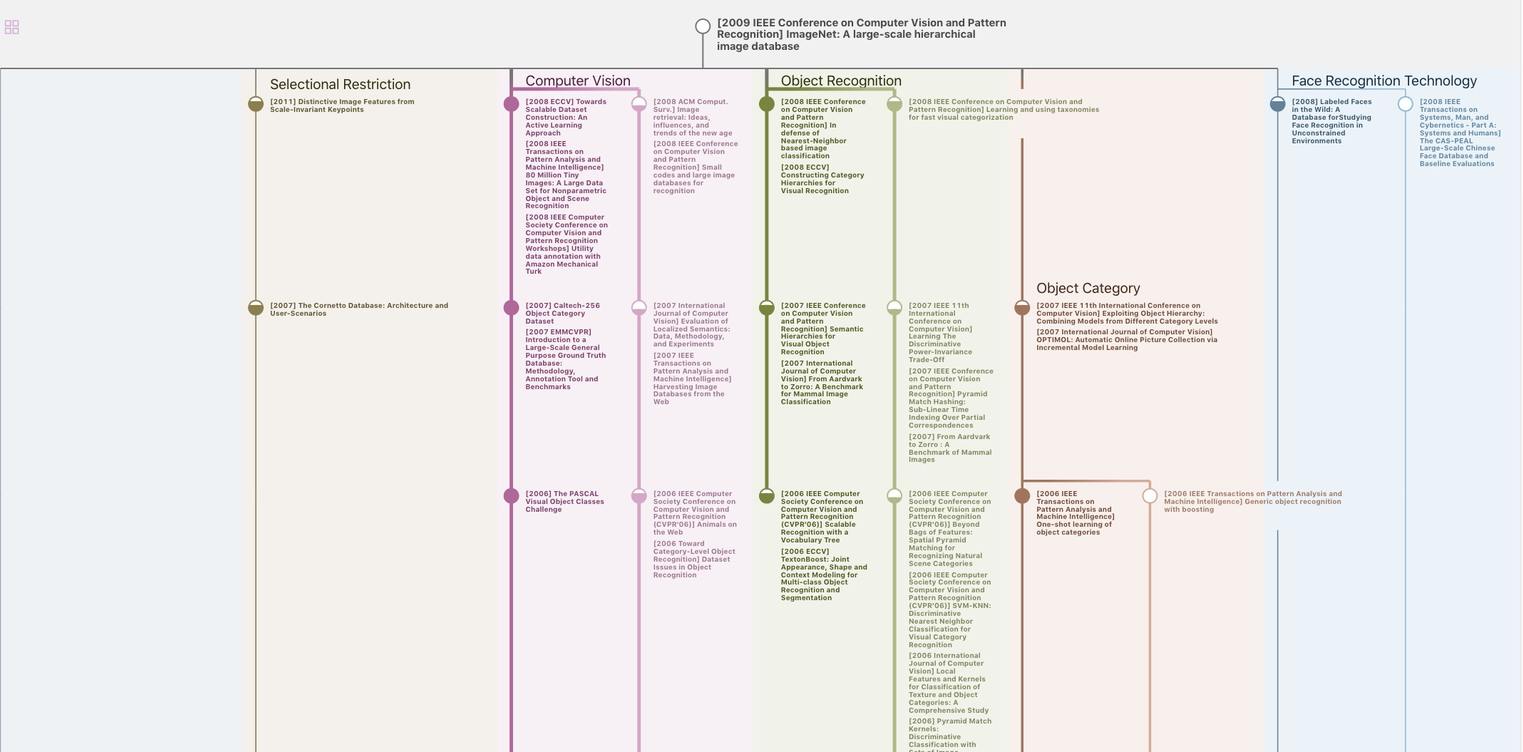
生成溯源树,研究论文发展脉络
Chat Paper
正在生成论文摘要