Multi-task Character-Level Attentional Networks for Medical Concept Normalization
Neural processing letters/Neural Processing Letters(2018)
摘要
Recognizing standard medical concepts in the colloquial text is significant for kinds of applications such as the medical question answering system. Recently, word-level neural network methods, which can learn complex informal expression features, achieved remarkable performance on this task. However, they have two main limitations: (1) Existing word-level methods cannot learn character structure features inside words and suffer from "Out-of-vocabulary" (OOV) words, which are common in noisy colloquial text. (2) Since these methods handle the normalization task as a classification issue, concept phrases are represented by category labels. Hence the word morphological information inside the concept is lost. In this work, we present a multi-task character-level attentional network model for medical concept normalization. Specifically, the character-level encoding scheme of our model can alleviate the OOV word problem. The attention mechanism can effectively exploit the word morphological information through multi-task training. It generates higher attention weights on domain-related positions in the text sequence, helping the downstream convolution focus on the characters that are related to medical concepts. To test our model, we first introduce a labeled Chinese dataset (overall 314,991 records) for this task. Other two real-world English datasets are also used. Our model outperforms state-of-the-art methods on all three datasets. Besides, by adding four types noises to the datasets, we validate the robustness of our model against common noises in the colloquial text.
更多查看译文
关键词
Convolutional neural network,Multi-task learning,Medical concept normalization
AI 理解论文
溯源树
样例
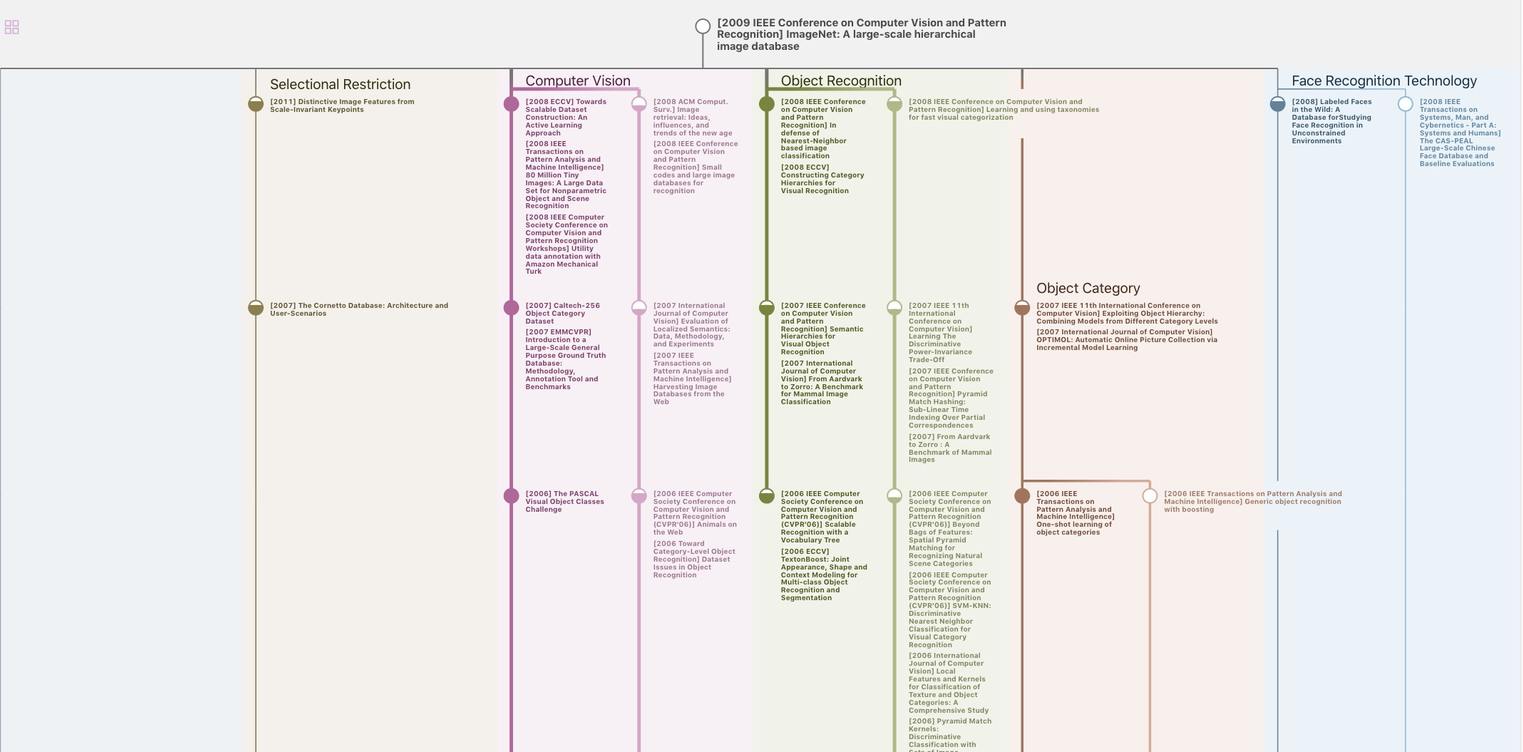
生成溯源树,研究论文发展脉络
Chat Paper
正在生成论文摘要