Recommendation algorithm based on improved spectral clustering and transfer learning
Pattern Analysis and Applications(2017)
摘要
Collaborative filtering (CF) recommendation has made great success in solving information overload. However, CF has some disadvantages such as cold start, data sparseness, low operation efficiency and knowledge cannot transfer between multiple rating matrixes. In this paper, we propose a recommendation algorithm based on improved spectral clustering and transfer learning (RAISCTL) to improve the forecasting accuracy and generalization ability of recommender system. RAISCTL firstly improves the spectral clustering by using the eigenvalue differences and orthogonal eigenvectors and realizes the automatic determination of cluster numbers. In addition, the improved spectral clustering algorithm is used to cluster the two dimensions of the users and items of the original rating matrix. Then, RAISCTL decomposes the rating matrix after clustering and gets the sharing group rating matrix. Finally, RAISCTL makes rating forecasting and recommendations based on the sharing group rating matrix and transfer learning. The simulation results show that RAISCTL can effectively improve the recommendation accuracy and generalization ability compared with other 8 conventional CF approaches.
更多查看译文
关键词
Spectral clustering,Recommendation algorithm,Recommender systems,Collaborative filtering,Transfer learning
AI 理解论文
溯源树
样例
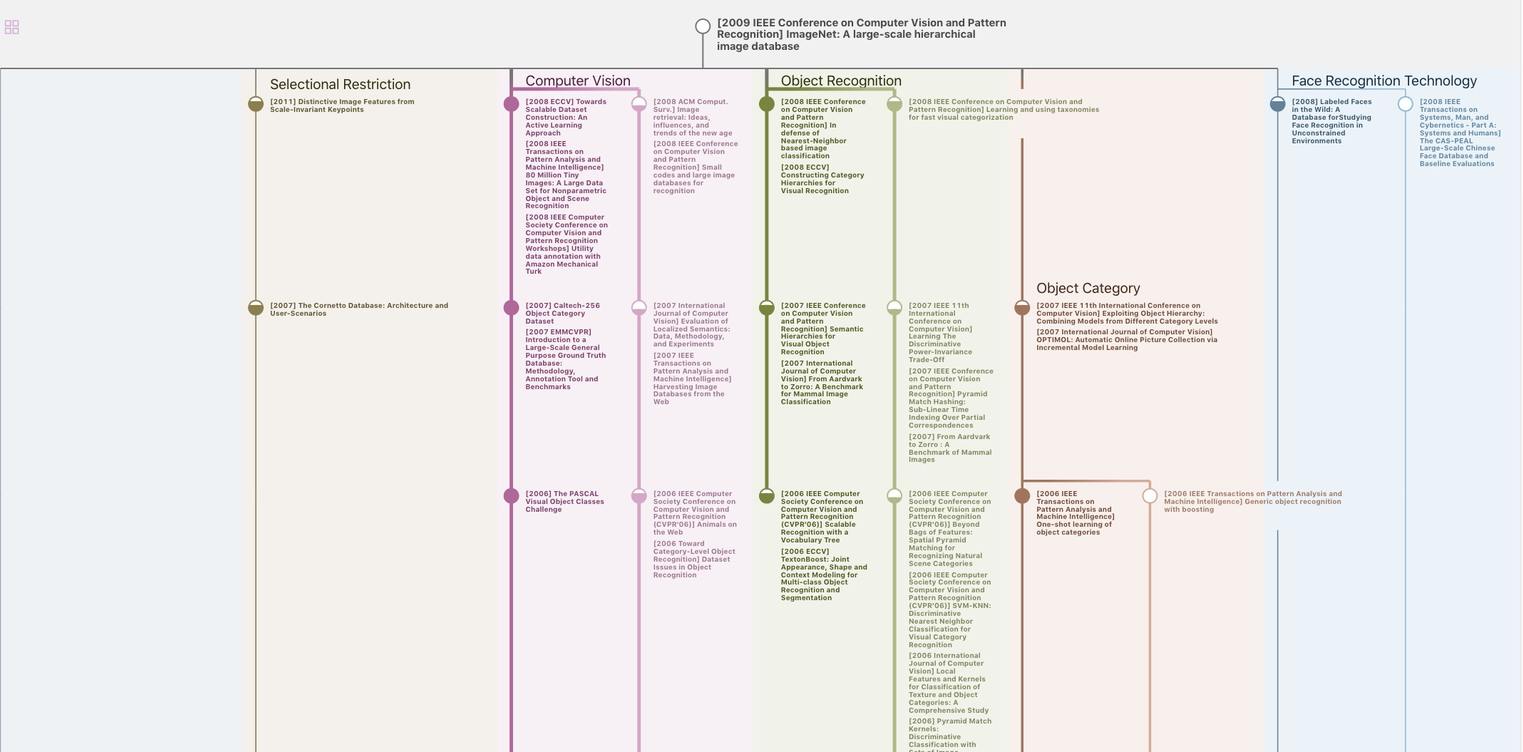
生成溯源树,研究论文发展脉络
Chat Paper
正在生成论文摘要