Studentized Autoregressive Time Series Residuals
Computational Statistics(2019)
摘要
Summary In this paper we develop large sample approximations for the variances of the residuals obtained from either a least squares or rank analysis of a first order autoregressive process. The formulas are elaborate, but can easily be computed either recursively or via an object oriented language such as S-PLUS. More importantly, our findings indicate that the variances of the residuals depend on much more than just the standard deviation of the error distribution. Thus, we caution against the use of the naive standardization for time series diagnostic procedures. Furthermore, the results can be used to form studentized residuals analogous to those used in the linear regression setting. We compare these new residuals to the conditionally studentized residuals via an example and simulation study. The study reveals some minor differences between the two sets of residuals in regards to outlier detection. Based on our findings, we conclude that the classical studentized linear regression residuals, found in such packages as SAS, SPSS, and RGLM can effectively be used in an autoregressive time series context.
更多查看译文
关键词
Autoregressive time series,Least squares,Outliers,R-Estimates,Studentized residuals
AI 理解论文
溯源树
样例
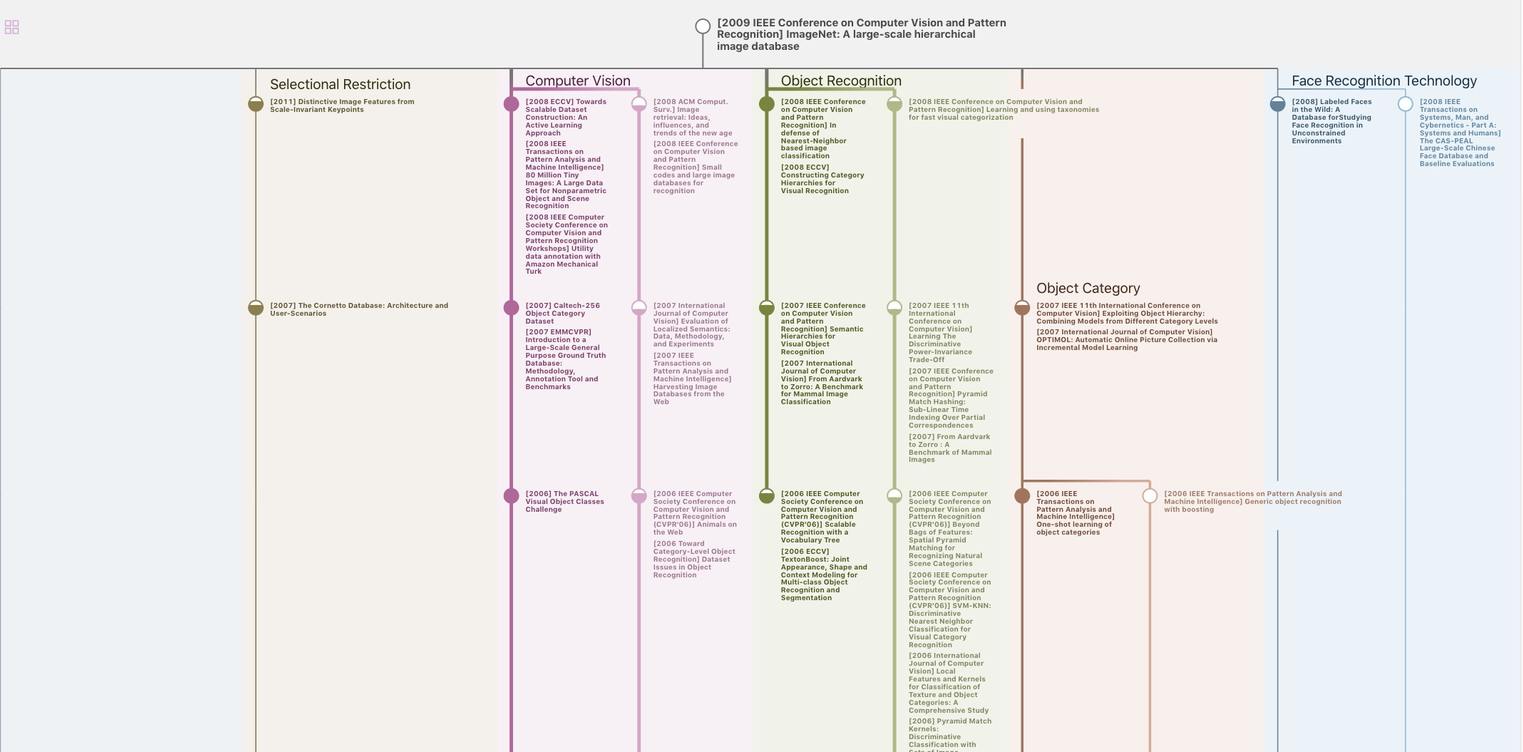
生成溯源树,研究论文发展脉络
Chat Paper
正在生成论文摘要