DeepType: On-Device Deep Learning for Input Personalization Service with Minimal Privacy Concern.
Proceedings of the ACM on Interactive, Mobile, Wearable and Ubiquitous Technologies(2018)
摘要
Mobile users spend an extensive amount of time on typing. A more efficient text input instrument brings a significant enhancement of user experience. Deep learning techniques have been recently applied to suggesting the next words of input, but to achieve more accurate predictions, these models should be customized for individual users. Personalization is often at the expense of privacy concerns. Existing solutions require users to upload the historical logs of their input text to the cloud so that a deep learning predictor can be trained. In this work, we propose a novel approach, called DeepType, to personalize text input with better privacy. The basic idea is intuitive: training deep learning predictors on the device instead of on the cloud, so that the model makes personalized and private data never leaves the device to externals. With DeepType, a global model is first trained on the cloud using massive public corpora, and our personalization is done by incrementally customizing the global model with data on individual devices. We further propose a set of techniques that effectively reduce the computation cost of training deep learning models on mobile devices at the cost of negligible accuracy loss. Experiments using real-world text input from millions of users demonstrate that DeepType significantly improves the input efficiency for individual users, and its incurred computation and energy costs are within the performance and battery restrictions of typical COTS mobile devices.
更多查看译文
关键词
Deep Learning,Mobile Computing,Personalization
AI 理解论文
溯源树
样例
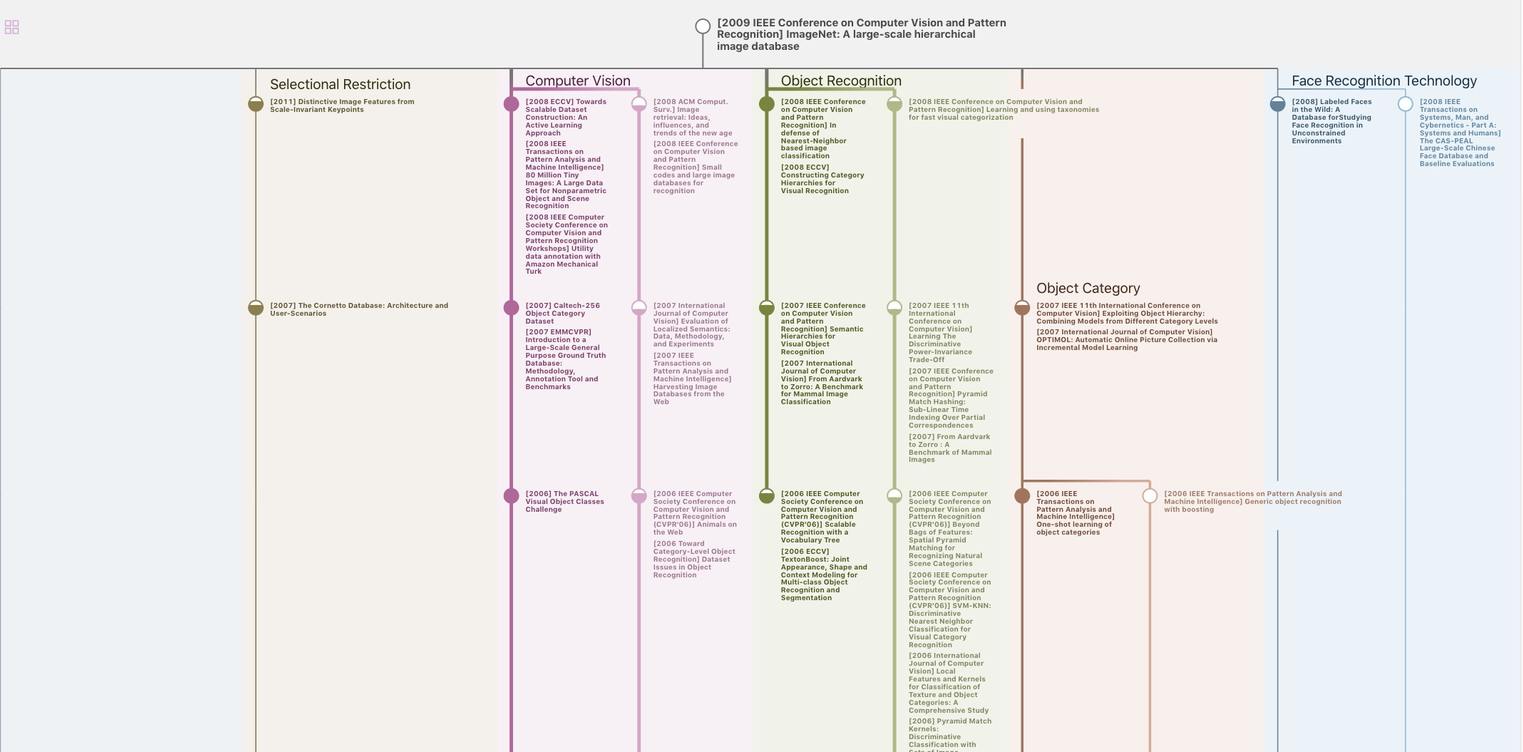
生成溯源树,研究论文发展脉络
Chat Paper
正在生成论文摘要