Hierarchical Human Machine Interaction Learning for a Lower Extremity Augmentation Device
International Journal of Social Robotics(2018)
摘要
For several years considerable effort has been devoted to the study of human augmentation robots. Traditionally, the focus of exoskeleton system has always been on model-based control framework. It seeks to model the dynamic system from prior knowledge of the robot as well as the pilot. However, in lower extremity exoskeleton, the control method depends on not only the modelling accuracy but also the physical human–machine interaction changed from personal physical conditions. To address this problem, in this paper, we present a model-free incremental human–machine interaction learning methodology. In a higher level, the methodology can plan the motion of exoskeleton with the sequence of rhythmic movement primitives. In the lower level, the gain scheming is updated from the dynamic system based on a novel proposed learning algorithm efficient PI^2 -CMA-ES. Compared with PI^BB , a particular feature is that it directly operates on the Cholesky decomposition of the covariance matrix, reducing the computational effort from O(n^3) to O(n^2) . To evaluate our proposed methodology, we not only demonstrate its applications on the single leg exoskeleton platform but also test on our lower extremity augmentation device. Experimental results show that the proposed methodology can minimize the interaction between the pilot and the exoskeleton compared with the traditional model-based control strategy.
更多查看译文
关键词
Exoskeleton,Rhythmic movement primitives (RMPs),Reinforcement learning,PI^2,CMA-ES,Human machine interaction (HMI)
AI 理解论文
溯源树
样例
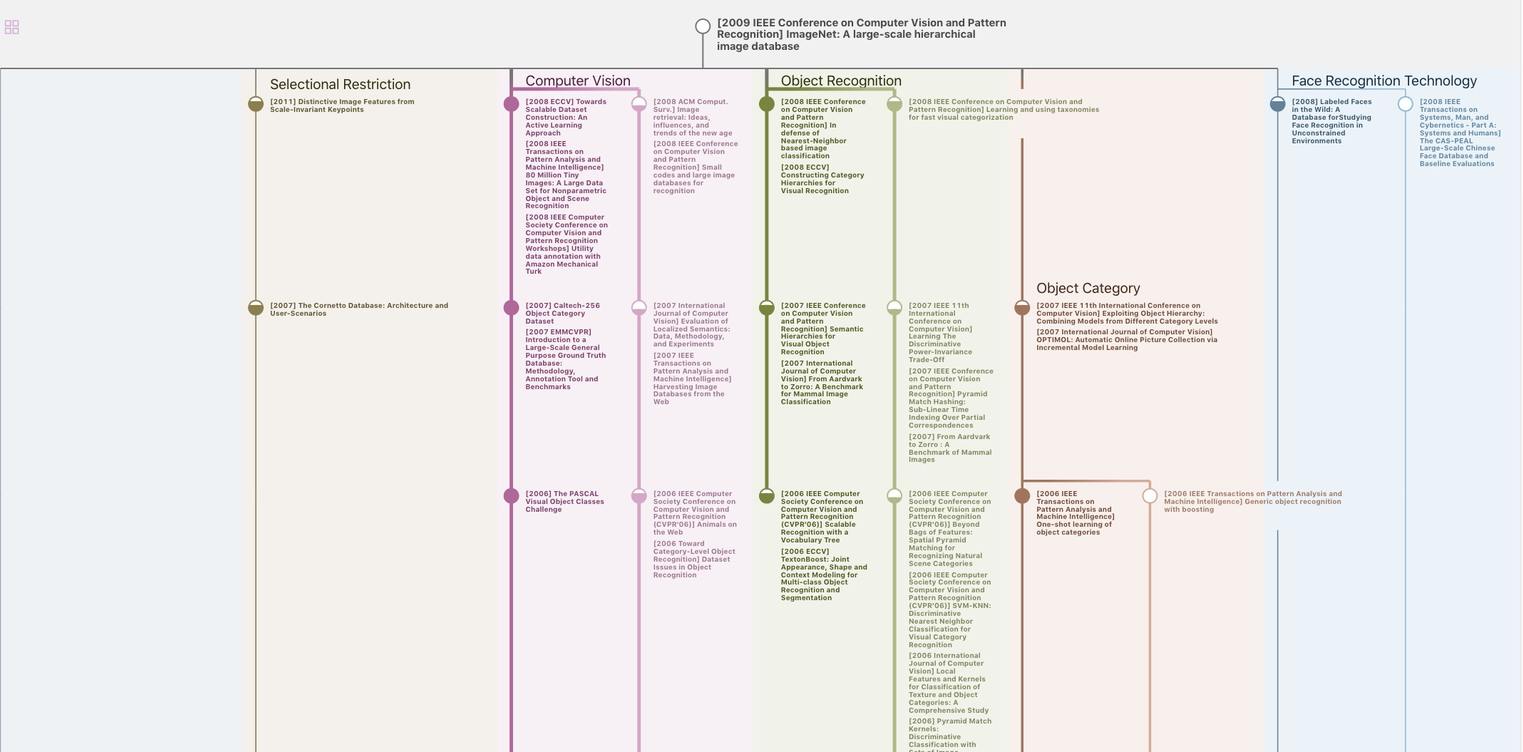
生成溯源树,研究论文发展脉络
Chat Paper
正在生成论文摘要